zELDA: fitting Lyman alpha line profiles using deep learning
MONTHLY NOTICES OF THE ROYAL ASTRONOMICAL SOCIETY(2022)
摘要
We present zELDA (redshift Estimator for Line profiles of Distant Lyman Alpha emitters), an open source code to fit Lyman alpha (Ly alpha) line profiles. The main motivation is to provide the community with an easy to use and fast tool to analyse Ly alpha line profiles uniformly to improve the understating of Ly alpha emitting galaxies. zELDA is based on line profiles of the commonly used 'shell-model' pre-computed with the full Monte Carlo radiative transfer code LyaRT. Via interpolation between these spectra and the addition of noise, we assemble a suite of realistic Ly alpha spectra which we use to train a deep neural network.We show that the neural network can predict the model parameters to high accuracy (e.g. less than or similar to 0.34 dex H I column density for R similar to 12 000) and thus allows for a significant speedup over existing fitting methods. As a proof of concept, we demonstrate the potential of zELDA by fitting 97 observed Ly alpha line profiles from the LASD data base. Comparing the fitted value with the measured systemic redshift of these sources, we find that Ly alpha determines their rest frame Ly alpha wavelength with a remarkable good accuracy of similar to 0.3 angstrom (similar to 75 km s(-1)). Comparing the predicted outflow properties and the observed Ly alpha luminosity and equivalent width, we find several possible trends. For example, we find an anticorrelation between the Ly alpha luminosity and the outflow neutral hydrogen column density, which might be explained by the radiative transfer process within galaxies.
更多查看译文
关键词
radiative transfer, Galaxies: ISM, ISM: jets and outflows
AI 理解论文
溯源树
样例
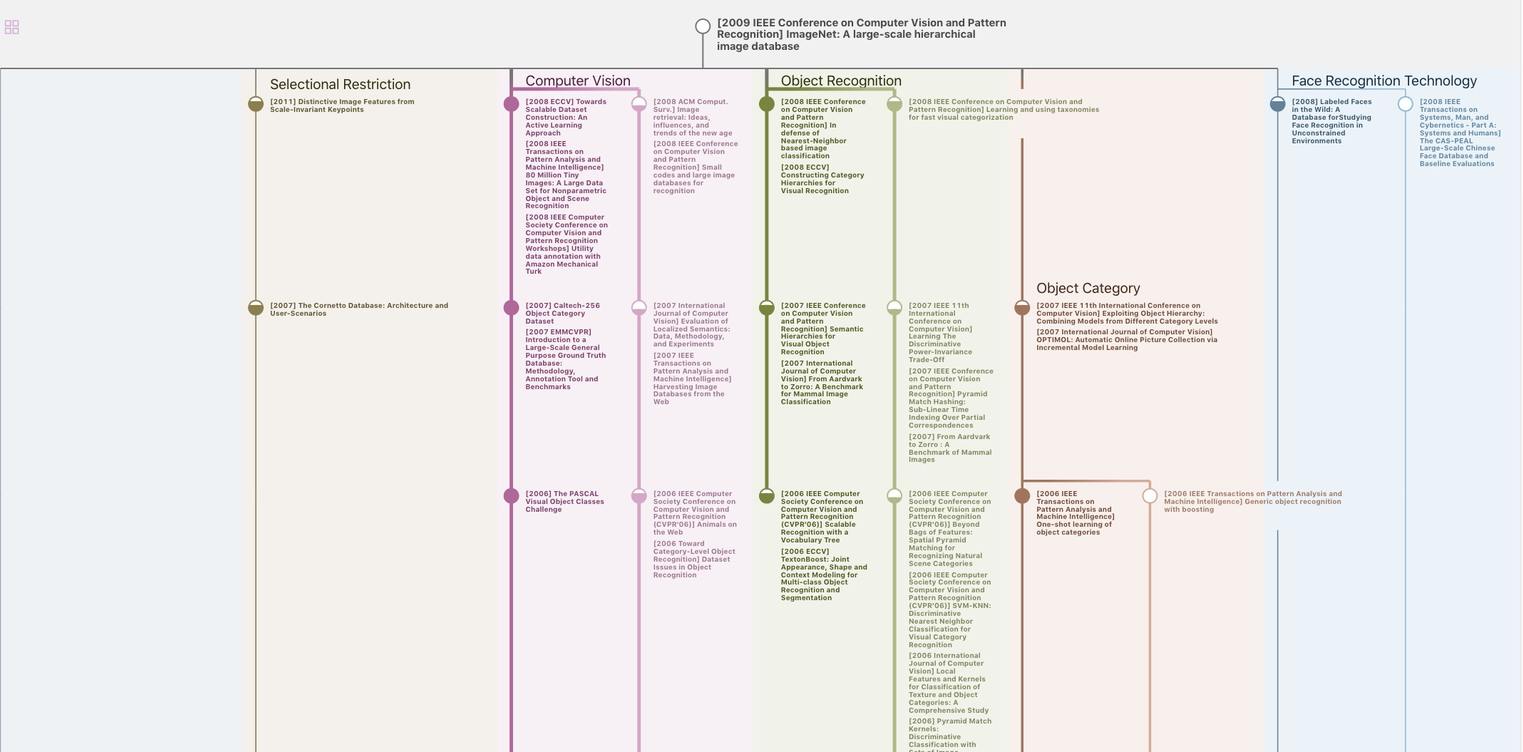
生成溯源树,研究论文发展脉络
Chat Paper
正在生成论文摘要