Node Feature Kernels Increase Graph Convolutional Network Robustness
INTERNATIONAL CONFERENCE ON ARTIFICIAL INTELLIGENCE AND STATISTICS, VOL 151(2022)
摘要
The robustness of the much used Graph Convolutional Networks (GCNs) to perturbations of their input is becoming a topic of increasing importance. In this paper the random GCN is introduced for which a random matrix theory analysis is possible. This analysis suggests that if the graph is sufficiently perturbed, or in the extreme case random, then the GCN fails to benefit from the node features. It is furthermore observed that enhancing the message passing step in GCNs by adding the node feature kernel to the adjacency matrix of the graph structure solves this problem. An empirical study of a GCN utilised for node classification on six real datasets further confirms the theoretical findings and demonstrates that perturbations of the graph structure can result in GCNs performing significantly worse than Multi-Layer Perceptrons run on the node features alone. In practice, adding a node feature kernel to the message passing of perturbed graphs results in a significant improvement of the GCN's performance, thereby rendering it more robust to graph perturbations. Our code is publicly available at: https: //github.com/ChangminWu/RobustGCN.
更多查看译文
关键词
graph,network
AI 理解论文
溯源树
样例
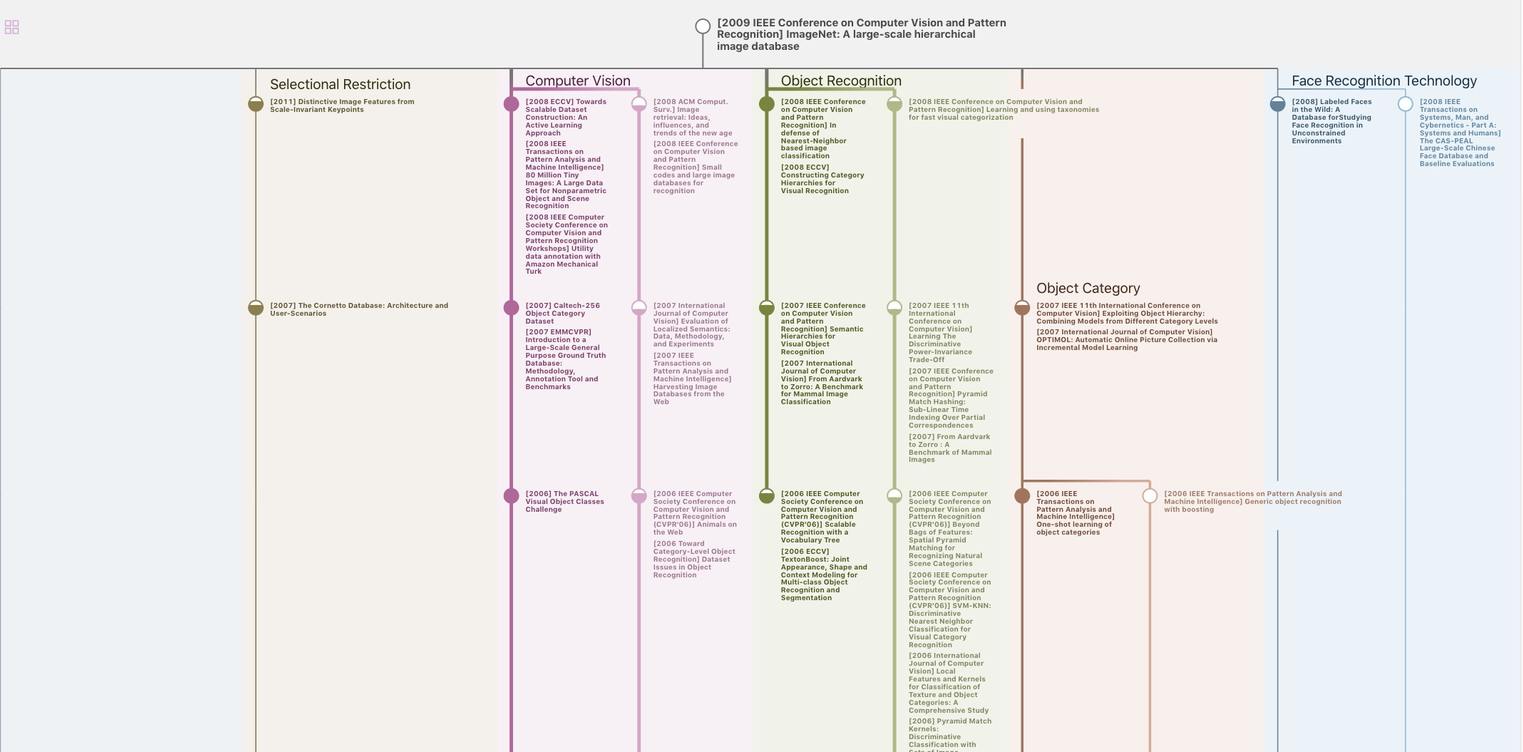
生成溯源树,研究论文发展脉络
Chat Paper
正在生成论文摘要