On the Out-of-distribution Generalization of Probabilistic Image Modelling.
Annual Conference on Neural Information Processing Systems(2021)
摘要
Out-of-distribution (OOD) detection and lossless compression constitute two problems that can be solved by the training of probabilistic models on a first dataset with subsequent likelihood evaluation on a second dataset, where data distributions differ. By defining the generalization of probabilistic models in terms of likelihood we show that, in the case of image models, the OOD generalization ability is dominated by local features. This motivates our proposal of a Local Autoregressive model that exclusively models local image features towards improving OOD performance. We apply the proposed model to OOD detection tasks and achieve state-of-the-art unsupervised OOD detection performance without the introduction of additional data. Additionally, we employ our model to build a new lossless image compressor: NeLLoC (Neural Local Lossless Compressor) and report state-of-the-art compression rates and model size.
更多查看译文
关键词
probabilistic image
AI 理解论文
溯源树
样例
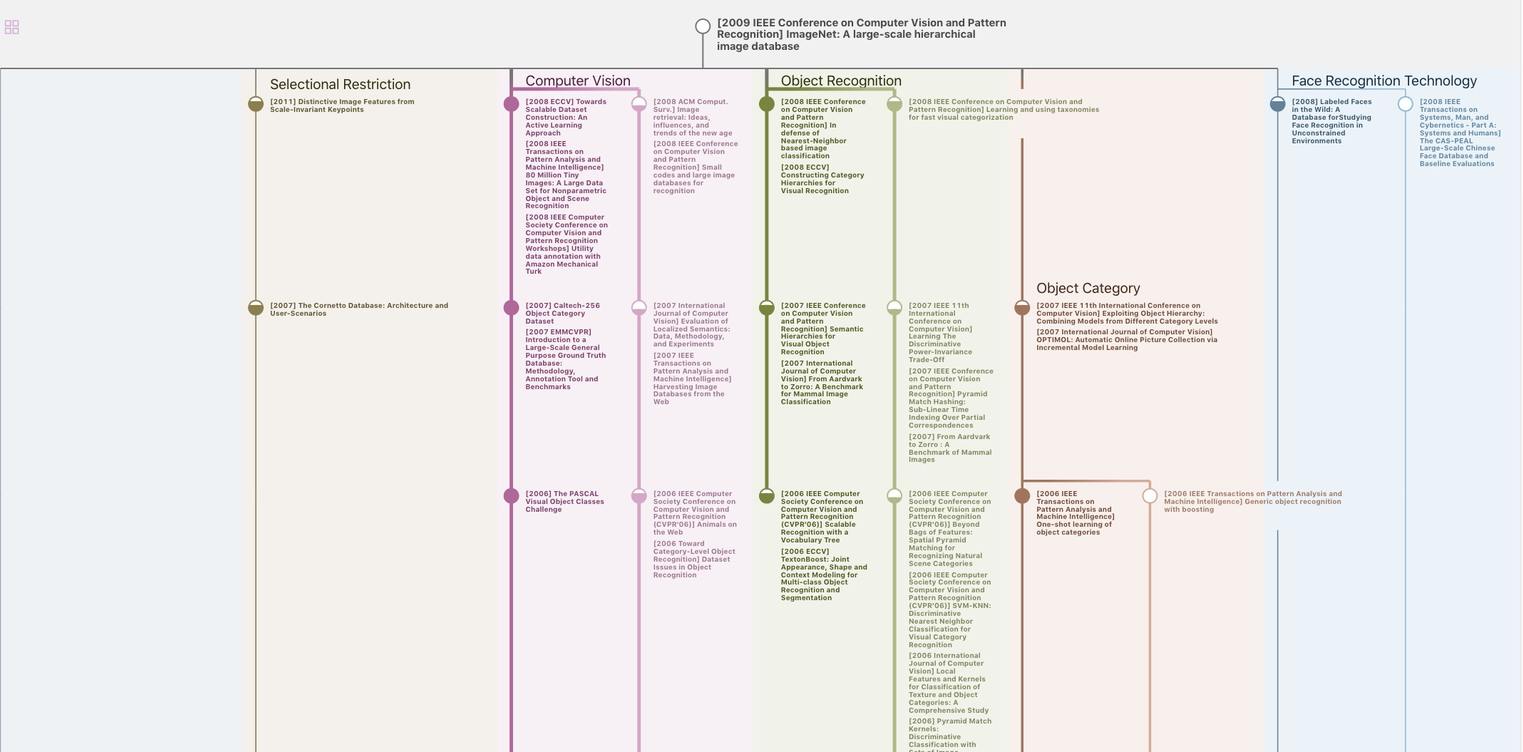
生成溯源树,研究论文发展脉络
Chat Paper
正在生成论文摘要