Screening the Discrepancy Function of a Computer Model
TECHNOMETRICS(2024)
摘要
Traditionally, screening refers to the problem of detecting influential (active) inputs in the computer model. We develop methodology that applies to screening, but the main focus is on detecting active inputs not in the computer model itself but rather on the discrepancy function that is introduced to account for model inadequacy when linking the computer model with field observations. We contend this is an important problem as it informs the modeler which are the inputs that are potentially being mishandled in the model, but also along which directions it may be less recommendable to use the model for prediction. The methodology is Bayesian and is inspired by the continuous spike-and-slab prior popularized by the literature on Bayesian variable selection. In our approach, and in contrast with previous proposals, a single MCMC sample from the full model allows us to compute the posterior probabilities of all the competing models, resulting in a methodology that is computationally very fast. The approach hinges on the ability to obtain posterior inclusion probabilities of the inputs, which are easy to interpret quantities, as the basis for selecting active inputs. For that reason, we name the methodology PIPS-posterior inclusion probability screening.
更多查看译文
关键词
Gaussian processes,Spike-and-slab prior,Uncertainty quantification,Variable selection
AI 理解论文
溯源树
样例
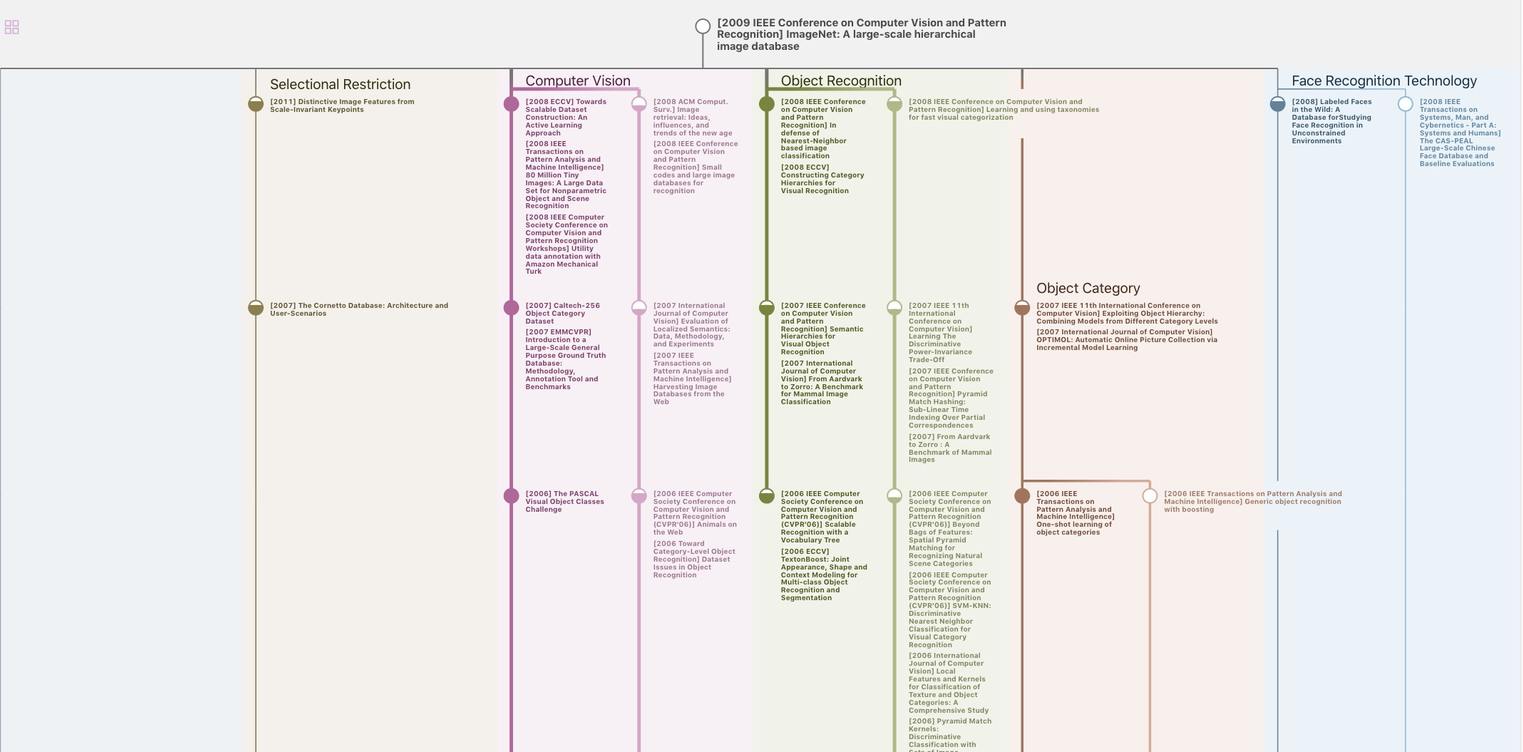
生成溯源树,研究论文发展脉络
Chat Paper
正在生成论文摘要