On the Approximation of Cooperative Heterogeneous Multi-Agent Reinforcement Learning (MARL) using Mean Field Control (MFC)
arxiv(2022)
摘要
Mean field control (MFC) is an effective way to mitigate the curse of dimensionality of cooperative multi-agent reinforcement learning (MARL) problems. This work considers a collection of NpOp heterogeneous agents that can be segregated into K classes such that the k-th class contains Nk homogeneous agents. We aim to prove approximation guarantees of the MARL problem for this heterogeneous system by its corresponding MFC problem. We consider three scenarios where the reward and transition dynamics of all agents are respec-tively taken to be functions of (1) joint state and action distributions across all classes, (2) individual distributions of each class, and (3) marginal distributions of the entire popula-tion. We show that, in these cases, the K-class MARL problem can be approximated by root|X|+root|U| E [\/ \/ ] E MFC with errors given as e1 = O(root Nk), e2 = O( Npopk |X | + |U|k root Nk ) 1 ([\/\/ ] [I E and e3 = O |X| + |U|ANpop k is an element of[K] , respectively, where A, B are root Nk + B root Np op some constants and |X|, |U| are the sizes of state and action spaces of each agent. Fi-nally, we design a Natural Policy Gradient (NPG) based algorithm that, in the three cases stated above, can converge to an optimal MARL policy within O(ej) error with a sample complexity of O(e-3 j ), j is an element of {1, 2, 3}, respectively.
更多查看译文
关键词
multi-agent learning, heterogeneous systems, mean-field control, approxima-tion guarantees, policy gradient algorithm
AI 理解论文
溯源树
样例
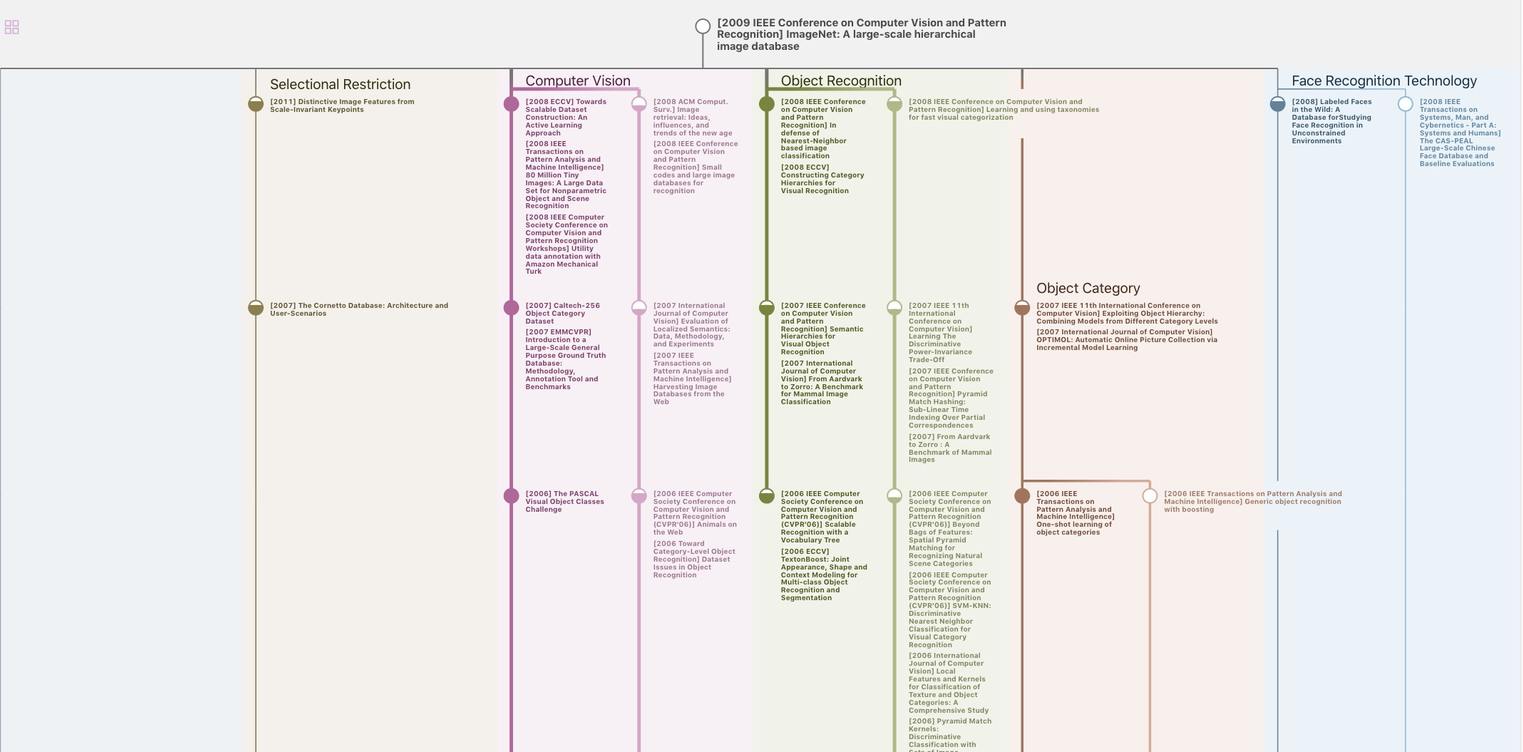
生成溯源树,研究论文发展脉络
Chat Paper
正在生成论文摘要