Generalised Unsupervised Domain Adaptation of Neural Machine Translation with Cross-Lingual Data Selection.
EMNLP(2021)
摘要
This paper considers the unsupervised domain adaptation problem for neural machine translation (NMT), where we assume the access to only monolingual text in either the source or target language in the new domain. We propose a cross-lingual data selection method to extract in-domain sentences in the missing language side from a large generic monolingual corpus. Our proposed method trains an adaptive layer on top of multilingual BERT by contrastive learning to align the representation between the source and target language. This then enables the transferability of the domain classifier between the languages in a zero-shot manner. Once the in-domain data is detected by the classifier, the NMT model is then adapted to the new domain by jointly learning translation and domain discrimination tasks. We evaluate our cross-lingual data selection method on NMT across five diverse domains in three language pairs, as well as a real-world scenario of translation for COVID-19. The results show that our proposed method outperforms other selection baselines up to +1.5 BLEU score.
更多查看译文
关键词
generalised unsupervised domain adaptation,domain adaptation,neural machine translation
AI 理解论文
溯源树
样例
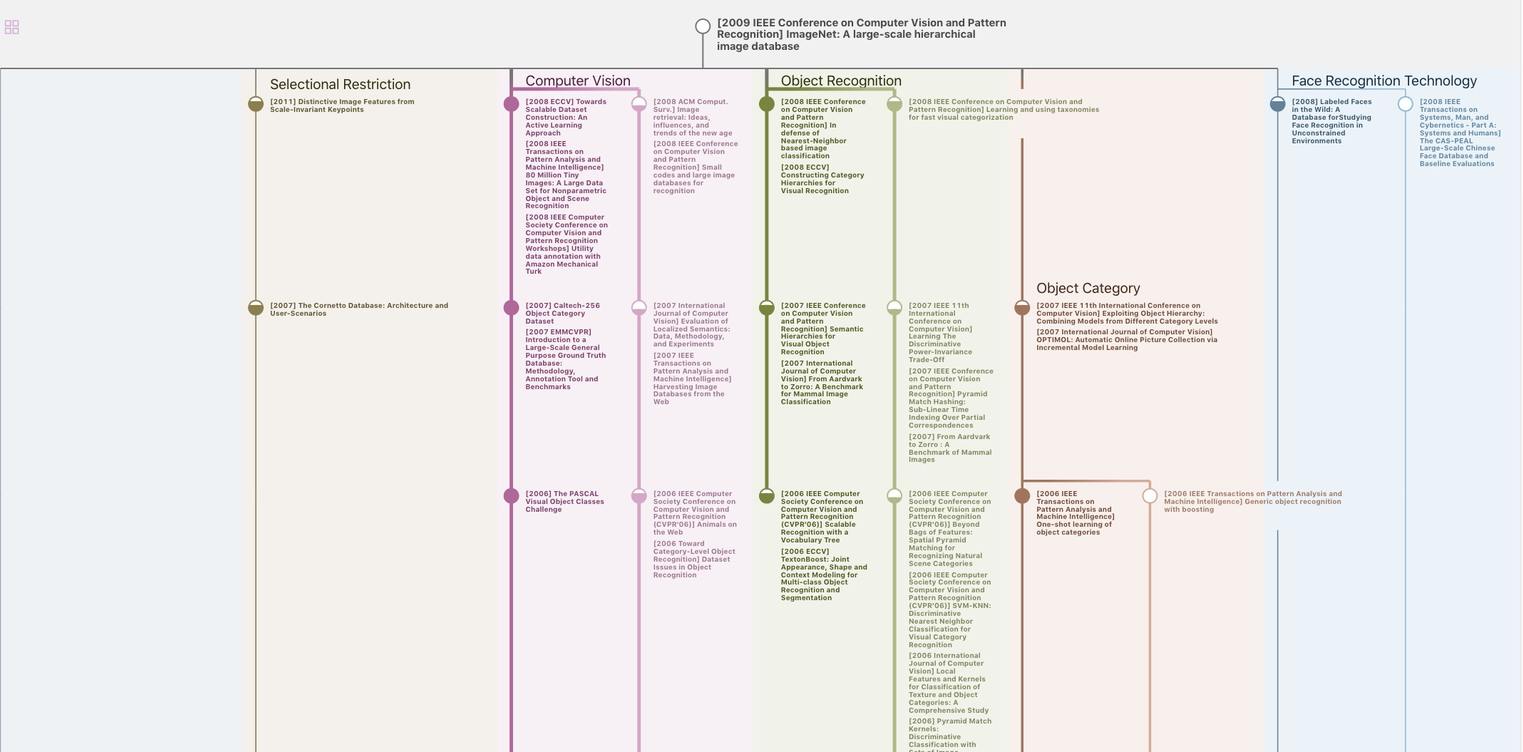
生成溯源树,研究论文发展脉络
Chat Paper
正在生成论文摘要