Virtual Adversarial Training-Based Deep Feature Aggregation Network from Dynamic Effective Connectivity for MCI Identification.
IEEE transactions on medical imaging(2022)
摘要
Dynamic functional connectivity (dFC) network inferred from resting-state fMRI reveals macroscopic dynamic neural activity patterns for brain disease identification. However, dFC methods ignore the causal influence between the brain regions. Furthermore, due to the complex non-Euclidean structure of brain networks, advanced deep neural networks are difficult to be applied for learning high-dimensional representations from brain networks. In this paper, a group constrained Kalman filter (gKF) algorithm is proposed to construct dynamic effective connectivity (dEC), where the gKF provides a more comprehensive understanding of the directional interaction within the dynamic brain networks than the dFC methods. Then, a novel virtual adversarial training convolutional neural network (VAT-CNN) is employed to extract the local features of dEC. The VAT strategy improves the robustness of the model to adversarial perturbations, and therefore avoids the overfitting problem effectively. Finally, we propose the high-order connectivity weight-guided graph attention networks (cwGAT) to aggregate features of dEC. By injecting the weight information of high-order connectivity into the attention mechanism, the cwGAT provides more effective high-level feature representations than the conventional GAT. The high-level features generated from the cwGAT are applied for binary classification and multiclass classification tasks of mild cognitive impairment (MCI). Experimental results indicate that the proposed framework achieves the classification accuracy of 90.9%, 89.8%, and 82.7% for normal control (NC) vs. early MCI (EMCI), EMCI vs. late MCI (LMCI), and NC vs. EMCI vs. LMCI classification respectively, outperforming the state-of-the-art methods significantly.
更多查看译文
关键词
Feature extraction,Diseases,Heuristic algorithms,Brain modeling,Time series analysis,Deep learning,Training,Computer-aided analysis,convolutional neural networks,deep learning,dynamic effective connectivity,graph attention networks,virtual adversarial training
AI 理解论文
溯源树
样例
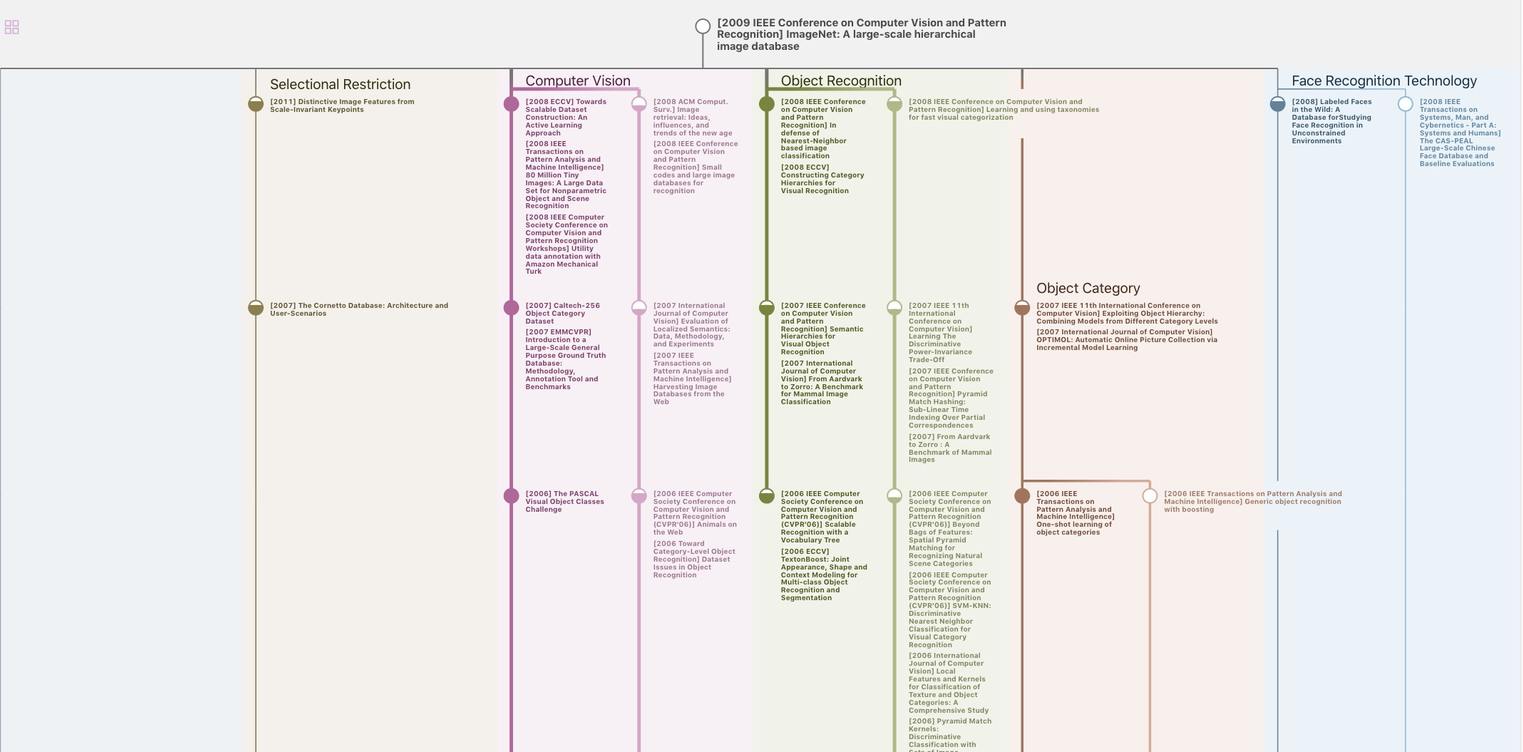
生成溯源树,研究论文发展脉络
Chat Paper
正在生成论文摘要