Directed-Graph-Learning-Based Diagnosis of Multiple Faults for High Speed Train With Switched Dynamics
IEEE Transactions on Cybernetics(2023)
摘要
This article addresses the distributed multiple fault isolation, modeling, and the closed-loop fault estimation under asynchronous switching for high speed train (HST) with switched dynamics, which is composed of traction, coasting, and braking. First, directed-graph-quantum-learning-based multiple-agent system (MAS) classifiers are introduced to characterize the joints effects of multiple faults. Some sufficient conditions are derived under the condition that the multiple fault topology contains a directed spanning tree and cycle edge, and these conditions guarantee that the multiple fault isolation problem can be solved under randomized learning techniques. Then, single-integrator agents are employed to capture the time-varying topology of multiple fault modeling, in which edge agreement and persistence condition are used to guarantee asymptotic consensus. After that, a novel robust fault estimation design along with the switched Lyapunov function and average dwell time is proposed for the possible power actuator faults subject to asynchronous switching and electromagnetic interferences. In addition, switched estimators are designed such that the closed-loop system is asymptotically stable. A multiple fault isolation and estimation case is investigated to validate the application of this methodology.
更多查看译文
关键词
Directed-graph-learning,fault estimation,fault isolation,fault modeling,high speed train (HST),multiple faults,switched dynamics
AI 理解论文
溯源树
样例
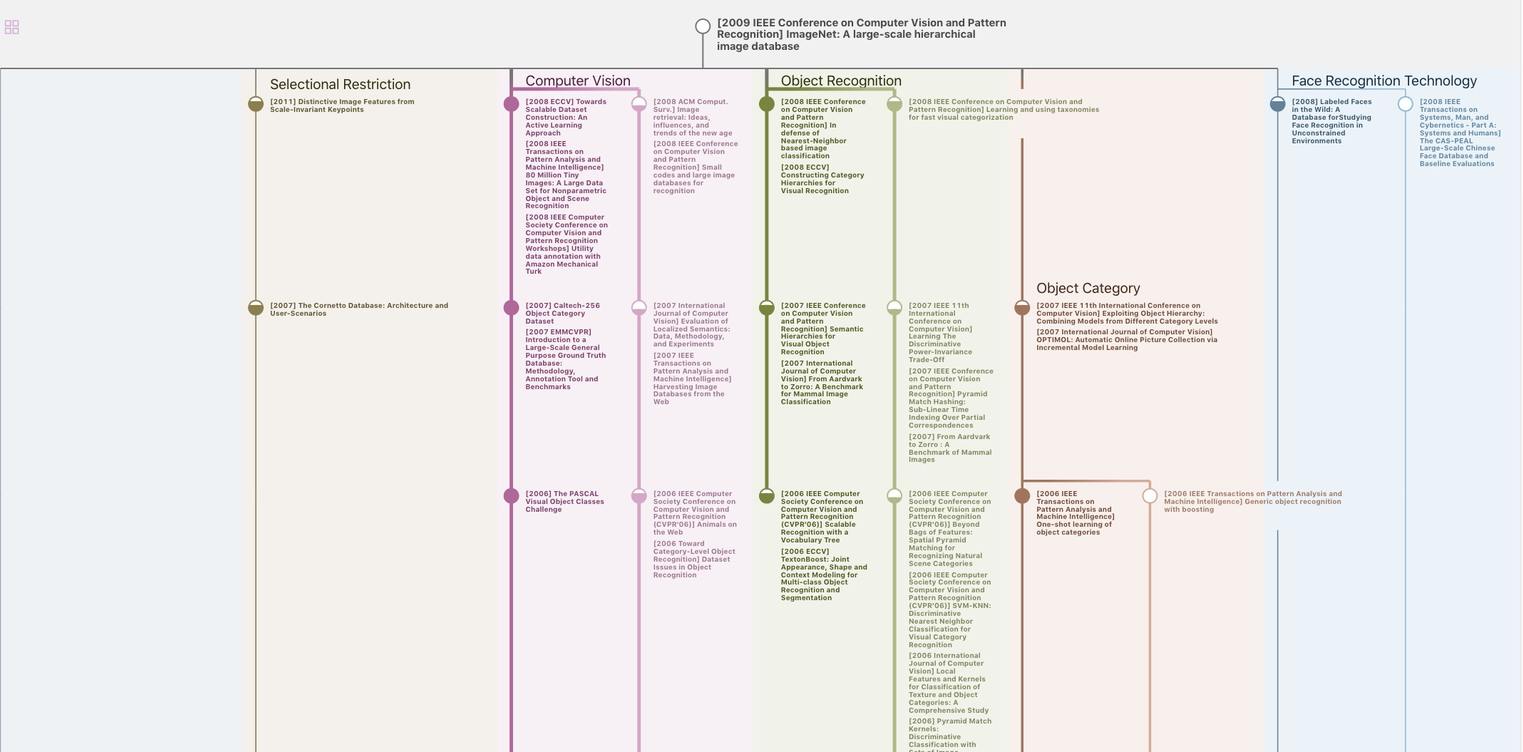
生成溯源树,研究论文发展脉络
Chat Paper
正在生成论文摘要