A Cnn Deep Local And Global Asd Classification Approach With Continuous Wavelet Transform Using Task-Based Fmri
SENSORS(2021)
摘要
Autism spectrum disorder (ASD) is a neurodegenerative disorder characterized by lingual and social disabilities. The autism diagnostic observation schedule is the current gold standard for ASD diagnosis. Developing objective computer aided technologies for ASD diagnosis with the utilization of brain imaging modalities and machine learning is one of main tracks in current studies to understand autism. Task-based fMRI demonstrates the functional activation in the brain by measuring blood oxygen level-dependent (BOLD) variations in response to certain tasks. It is believed to hold discriminant features for autism. A novel computer aided diagnosis (CAD) framework is proposed to classify 50 ASD and 50 typically developed toddlers with the adoption of CNN deep networks. The CAD system includes both local and global diagnosis in a response to speech task. Spatial dimensionality reduction with region of interest selection and clustering has been utilized. In addition, the proposed framework performs discriminant feature extraction with continuous wavelet transform. Local diagnosis on cingulate gyri, superior temporal gyrus, primary auditory cortex and angular gyrus achieves accuracies ranging between 71% and 80% with a four-fold cross validation technique. The fused global diagnosis achieves an accuracy of 86% with 82% sensitivity, 92% specificity. A brain map indicating ASD severity level for each brain area is created, which contributes to personalized diagnosis and treatment plans.
更多查看译文
关键词
autism, ASD, computer-aided diagnosis, deep learning, CNN, CWT
AI 理解论文
溯源树
样例
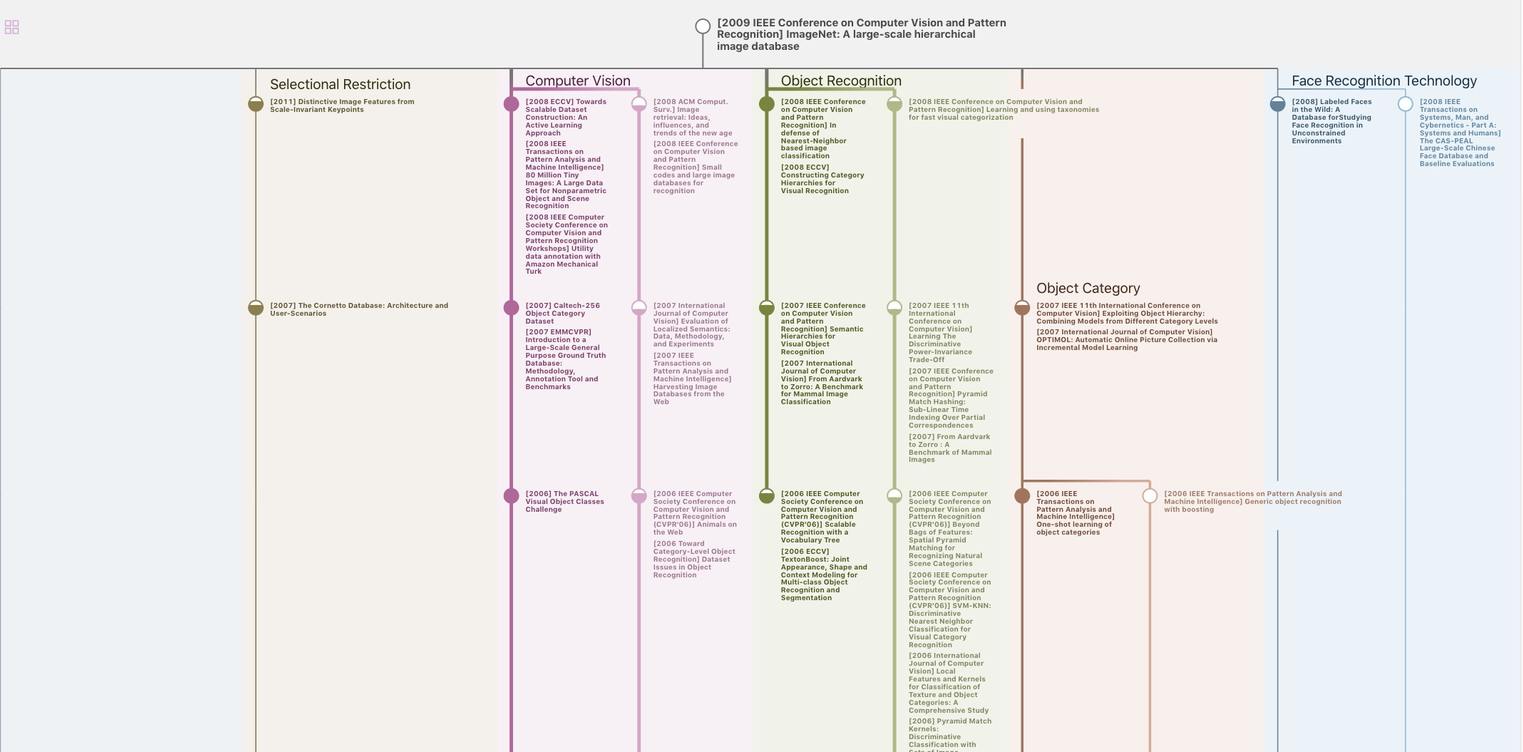
生成溯源树,研究论文发展脉络
Chat Paper
正在生成论文摘要