Pose-Guided Hierarchical Semantic Decomposition and Composition for Human Parsing
IEEE Transactions on Cybernetics(2023)
摘要
Human parsing is a fine-grained semantic segmentation task, which needs to understand human semantic parts. Most existing methods model human parsing as a general semantic segmentation, which ignores the inherent relationship among hierarchical human parts. In this work, we propose a pose-guided hierarchical semantic decomposition and composition framework for human parsing. Specifically, our method includes a semantic maintained decomposition and composition (SMDC) module and a pose distillation (PC) module. SMDC progressively disassembles the human body to focus on the more concise regions of interest in the decomposition stage and then gradually assembles human parts under the guidance of pose information in the composition stage. Notably, SMDC maintains the atomic semantic labels during both stages to avoid the error propagation issue of the hierarchical structure. To further take advantage of the relationship of human parts, we introduce pose information as explicit guidance for the composition. However, the discrete structure prediction in pose estimation is against the requirement of the continuous region in human parsing. To this end, we design a PC module to broadcast the maximum responses of pose estimation to form the continuous structure in the way of knowledge distillation. The experimental results on the look-into-person (LIP) and PASCAL-Person-Part datasets demonstrate the superiority of our method compared with the state-of-the-art methods, that is, 55.21% mean Intersection of Union (mIoU) on LIP and 69.88% mIoU on PASCAL-Person-Part.
更多查看译文
关键词
Human parsing,pose distillation (PC),semantic maintained decomposition and composition (SMDC)
AI 理解论文
溯源树
样例
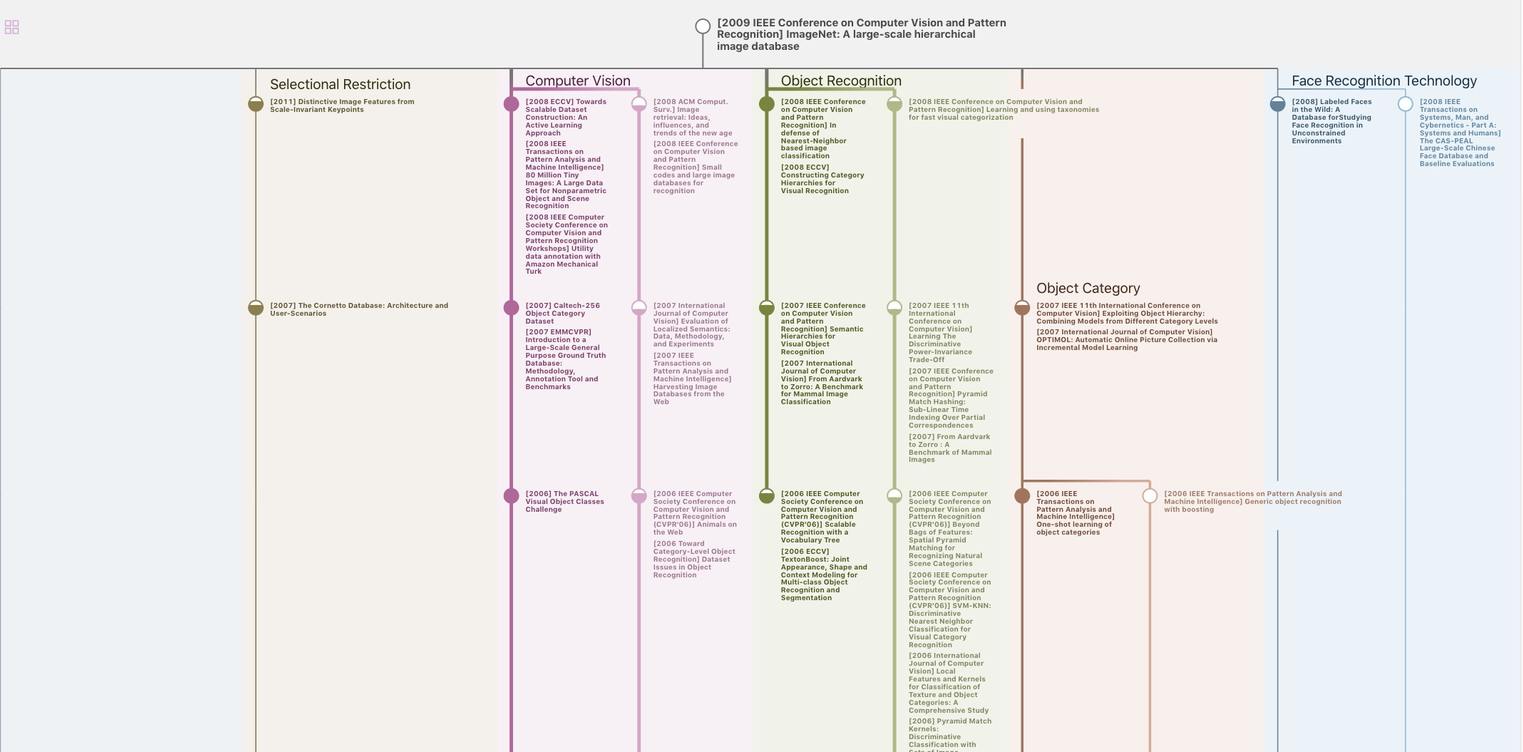
生成溯源树,研究论文发展脉络
Chat Paper
正在生成论文摘要