Mixture-of-Partitions - Infusing Large Biomedical Knowledge Graphs into BERT.
EMNLP(2021)
摘要
Infusing factual knowledge into pre-trained models is fundamental for many knowledge-intensive tasks. In this paper, we proposed Mixture-of-Partitions (MoP), an infusion approach that can handle a very large knowledge graph (KG) by partitioning it into smaller sub-graphs and infusing their specific knowledge into various BERT models using lightweight adapters. To leverage the overall factual knowledge for a target task, these sub-graph adapters are further fine-tuned along with the underlying BERT through a mixture layer. We evaluate our MoP with three biomedical BERTs (SciBERT, BioBERT, PubmedBERT) on six downstream tasks (inc. NLI, QA, Classification), and the results show that our MoP consistently enhances the underlying BERTs in task performance, and achieves new SOTA performances on five evaluated datasets.
更多查看译文
关键词
Knowledge Graph Embedding,Medical Concept Embedding,Signal Processing on Graphs,Representation Learning
AI 理解论文
溯源树
样例
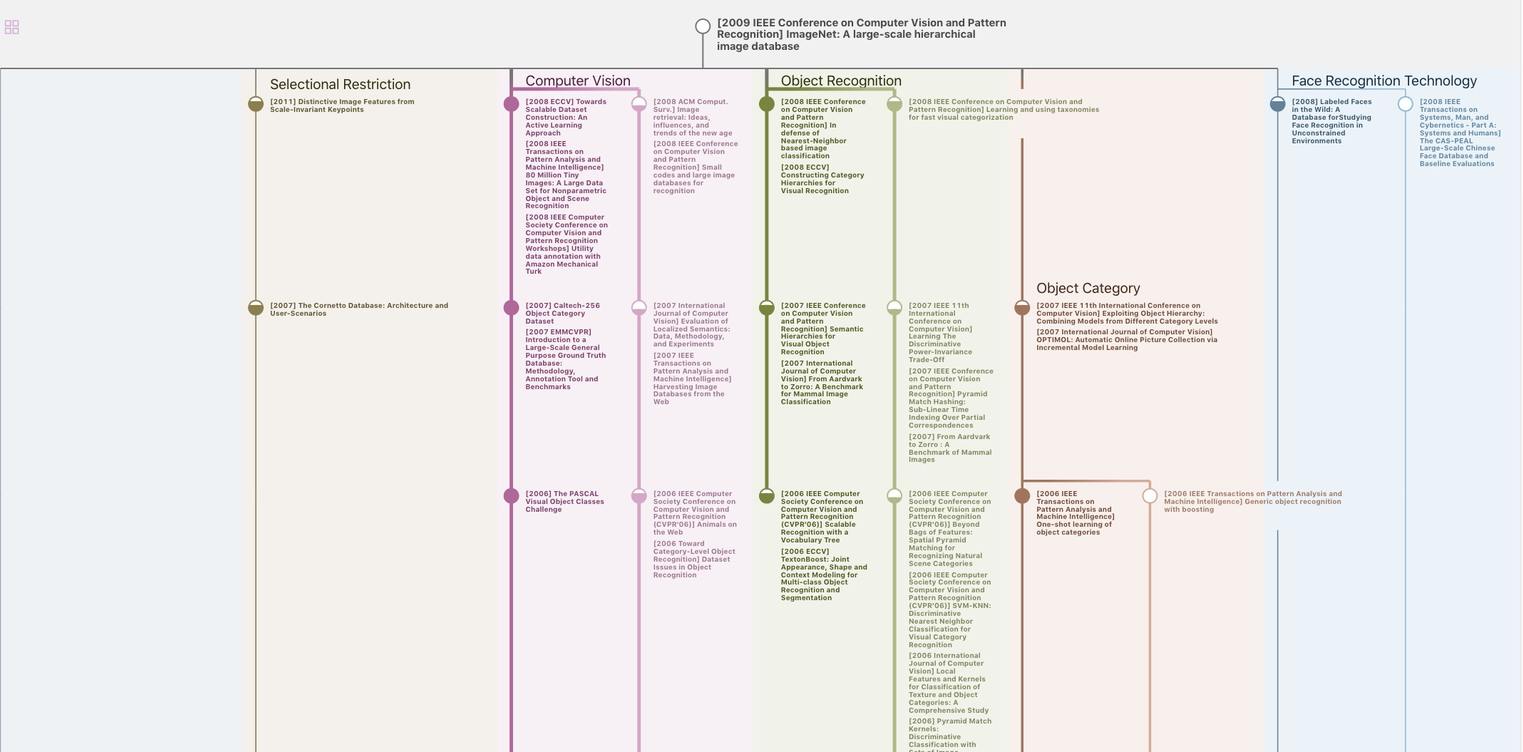
生成溯源树,研究论文发展脉络
Chat Paper
正在生成论文摘要