Attention-based Contrastive Learning for Winograd Schemas.
EMNLP(2021)
摘要
Self-supervised learning has recently attracted considerable attention in the NLP community for its ability to learn discriminative features using a contrastive objective. This paper investigates whether contrastive learning can be extended to Transfomer attention to tackling the Winograd Schema Challenge. To this end, we propose a novel self-supervised framework, leveraging a contrastive loss directly at the level of self-attention. Experimental analysis of our attention-based models on multiple datasets demonstrates superior commonsense reasoning capabilities. The proposed approach outperforms all comparable unsupervised approaches while occasionally surpassing supervised ones.
更多查看译文
关键词
winograd schemas,contrastive learning,attention-based
AI 理解论文
溯源树
样例
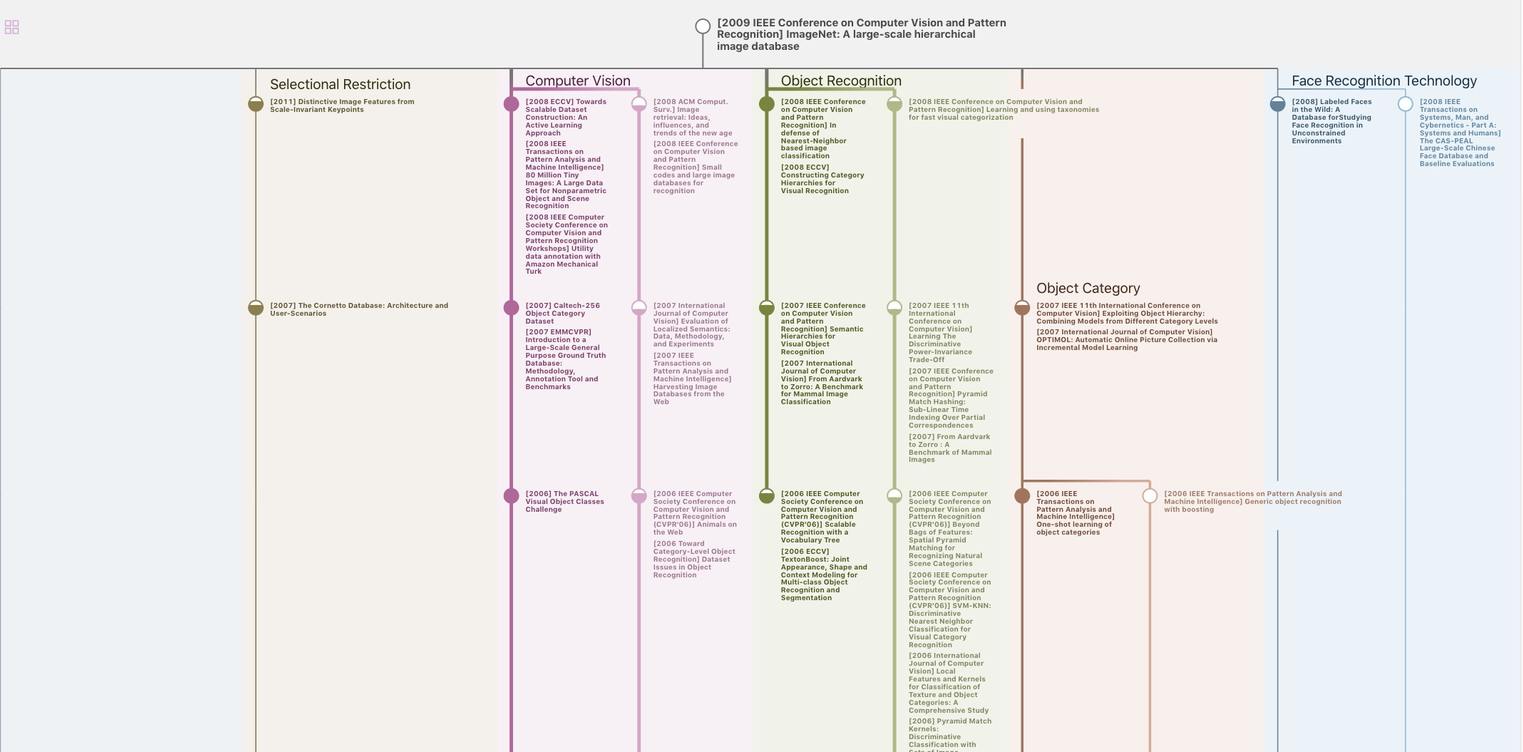
生成溯源树,研究论文发展脉络
Chat Paper
正在生成论文摘要