Using Machine Learning for Risk Classification in Brazilian Federal Voluntary Transfers
ELECTRONIC GOVERNMENT AND THE INFORMATION SYSTEMS PERSPECTIVE, EGOVIS 2021(2021)
摘要
Along with the re-democratization process in Brazil, states and municipalities have started to rely on voluntary transfers of resources through agreements with the Federal Government to execute their public policies. To improve timeliness in the recovery process of default resource usage, a classification tool is necessary to assign risk profiles of the success or failure of these transfers. In this paperwork, we propose the use of the eXtreme Gradient Boosting (XGBoost) algorithm using balanced and unbalanced data sets, using Tree-structured Parzen Bayesian Estimator (TPE) hyperparameter optimization techniques. The results achieved good success rates. Results for XGBoost using balanced data showed a recall of 89.3% and unbalanced data 87.8%. However, for unbalanced data, the AUC score was 98.1%, against 97.9% for balanced data.
更多查看译文
关键词
Metadata, Voluntary transfers, Machine learning, XGBoost, Risk prediction
AI 理解论文
溯源树
样例
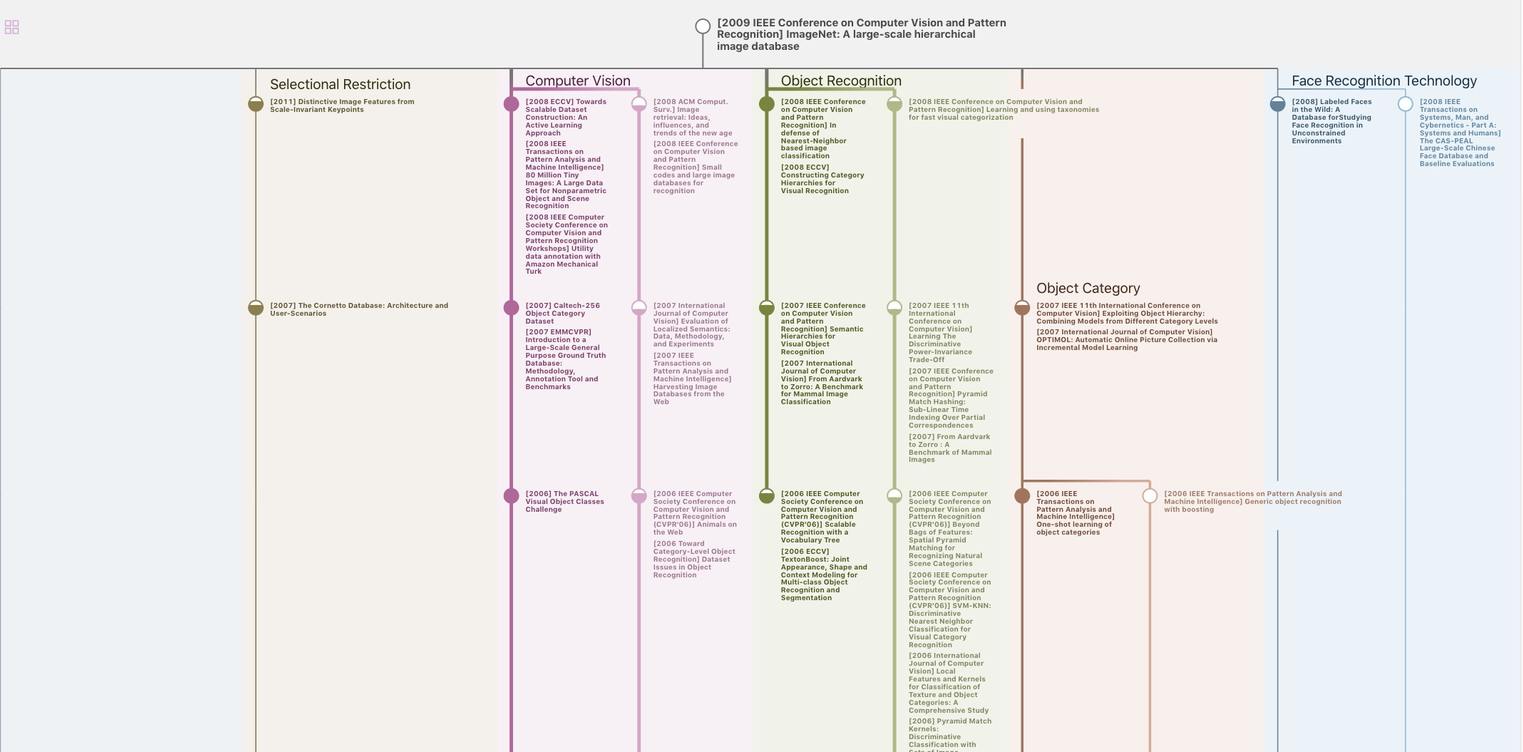
生成溯源树,研究论文发展脉络
Chat Paper
正在生成论文摘要