Can Language Models Identify Wikipedia Articles with Readability and Style Issues?
ICTIR(2021)
摘要
Wikipedia is frequently criticised for having poor readability and style issues. In this article, we investigate using GPT-2, a neural language model, to identify poorly written text in Wikipedia by ranking documents by their perplexity. We evaluated the properties of this ranking using human assessments of text quality, including readability, narrativity and language use. We demonstrate that GPT-2 perplexity scores correlate moderately to strongly with narrativity, but only weakly with reading comprehension scores. Importantly, the model reflects even small improvements to text as would be seen in Wikipedia edits. We conclude by highlighting that Wikipedia's featured articles counter-intuitively contain text with the highest perplexity scores. However, these examples highlight many of the complexities that need to be resolved for such an approach to be used in practice.
更多查看译文
AI 理解论文
溯源树
样例
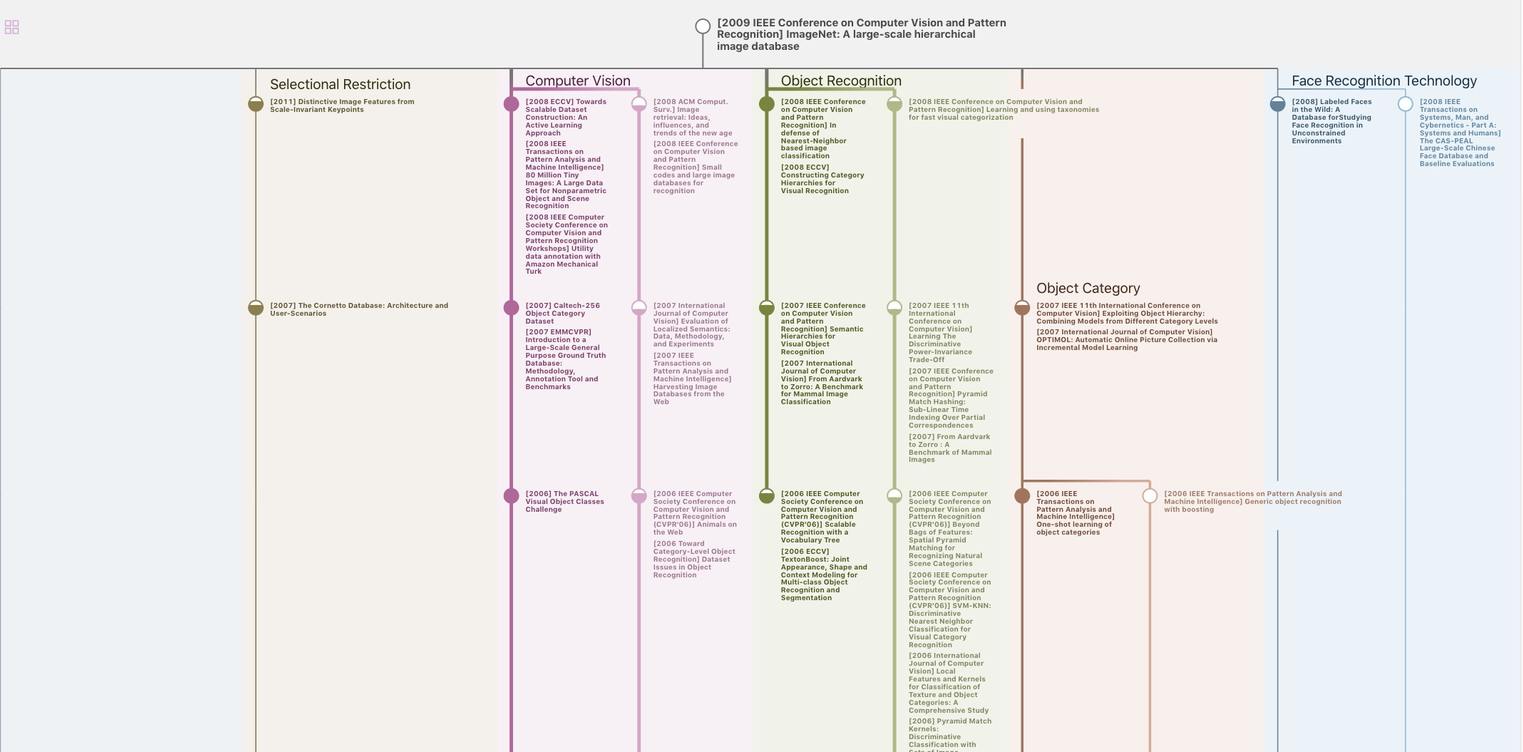
生成溯源树,研究论文发展脉络
Chat Paper
正在生成论文摘要