The Perils of Using Mechanical Turk to Evaluate Open-Ended Text Generation.
EMNLP(2021)
摘要
Recent text generation research has increasingly focused on open-ended domains such as story and poetry generation. Because models built for such tasks are difficult to evaluate automatically, most researchers in the space justify their modeling choices by collecting crowdsourced human judgments of text quality (e.g., Likert scores of coherence or grammaticality) from Amazon Mechanical Turk (AMT). In this paper, we first conduct a survey of 45 open-ended text generation papers and find that the vast majority of them fail to report crucial details about their AMT tasks, hindering reproducibility. We then run a series of story evaluation experiments with both AMT workers and English teachers and discover that even with strict qualification filters, AMT workers (unlike teachers) fail to distinguish between model-generated text and human-generated references. We show that AMT worker judgments improve when they are shown model-generated output alongside human-generated references, which enables the workers to better calibrate their ratings. Finally, interviews with the English teachers provide deeper insights into the challenges of the evaluation process, particularly when rating model-generated text.
更多查看译文
关键词
text generation,mechanical turk,open-ended
AI 理解论文
溯源树
样例
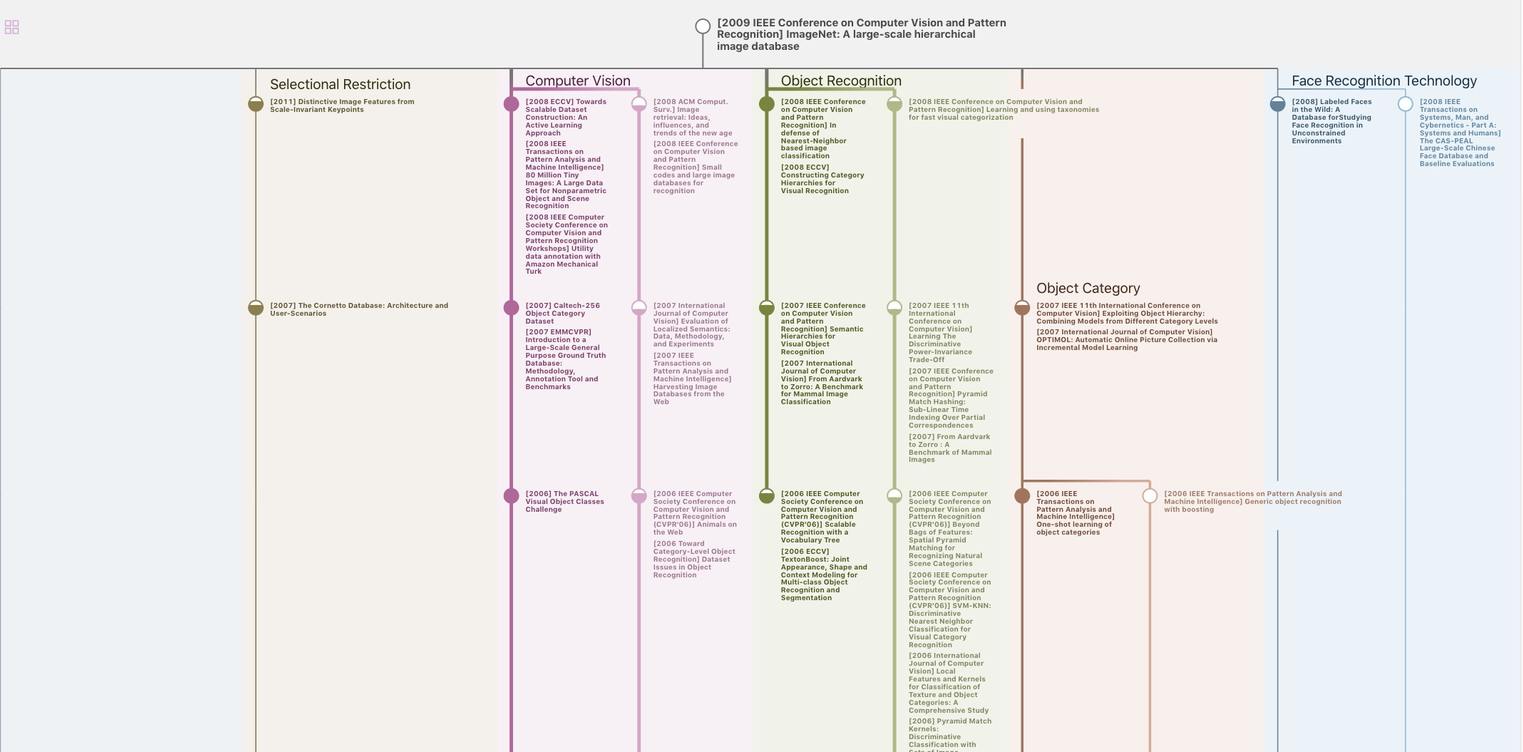
生成溯源树,研究论文发展脉络
Chat Paper
正在生成论文摘要