Resolution-robust Large Mask Inpainting with Fourier Convolutions.
2022 IEEE WINTER CONFERENCE ON APPLICATIONS OF COMPUTER VISION (WACV 2022)(2021)
摘要
Modern image inpainting systems, despite the significant progress, often struggle with large missing areas, complex geometric structures, and high-resolution images. We find that one of the main reasons for that is the lack of an effective receptive field in both the inpainting network and the loss function. To alleviate this issue, we propose a new method called large mask inpainting (LaMa). LaMa is based on i) a new inpainting network architecture that uses fast Fourier convolutions (FFCs), which have the image-wide receptive field; ii) a high receptive field perceptual loss; iii) large training masks, which unlocks the potential of the first two components. Our inpainting network improves the state-of-the-art across a range of datasets and achieves excellent performance even in challenging scenarios, e.g. completion of periodic structures. Our model generalizes surprisingly well to resolutions that are higher than those seen at train time, and achieves this at lower parameter&time costs than the competitive baselines. The code is available at https://github.com/saic-mdal/lama.
更多查看译文
关键词
fourier,resolution-robust
AI 理解论文
溯源树
样例
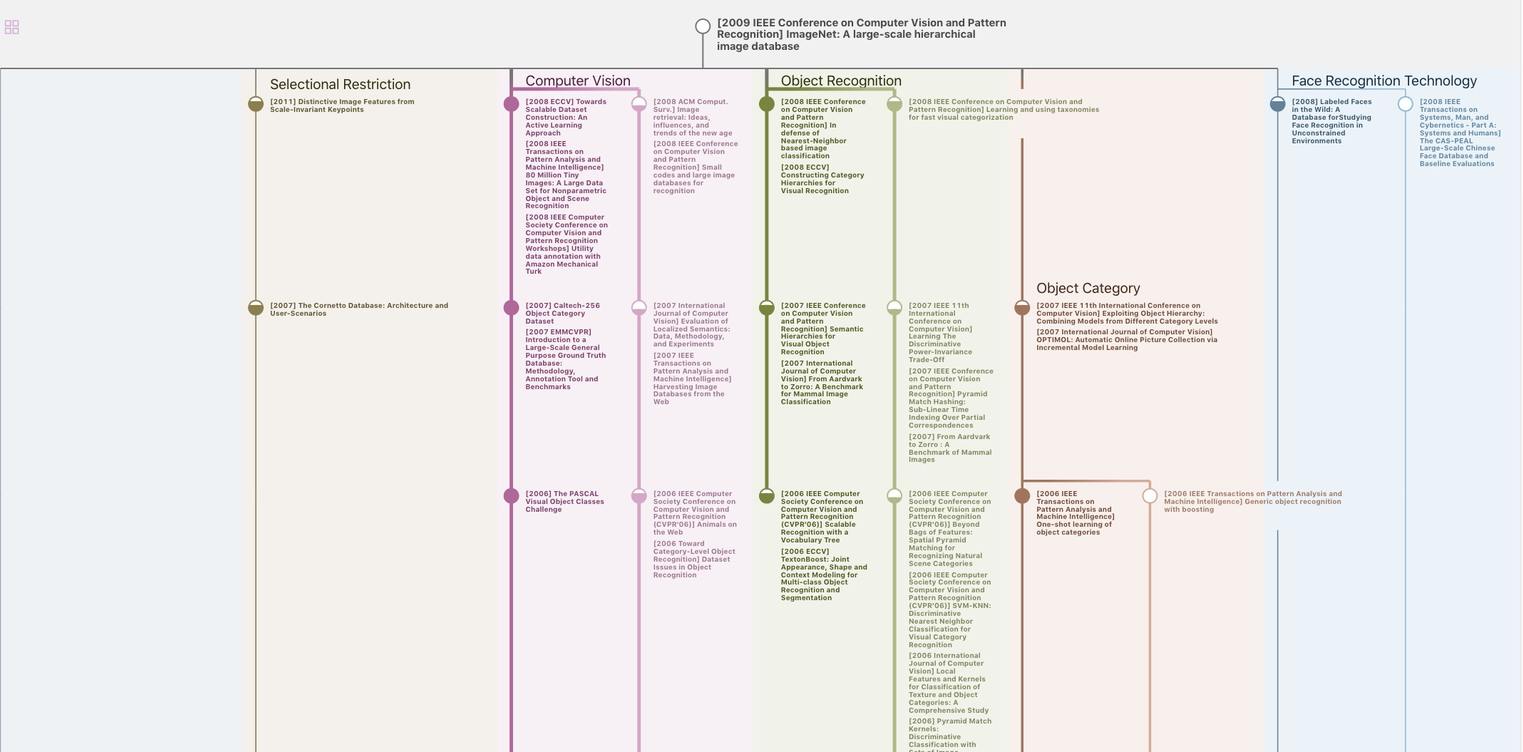
生成溯源树,研究论文发展脉络
Chat Paper
正在生成论文摘要