Biologically motivated learning method for deep neural networks using hierarchical competitive learning.
Neural networks : the official journal of the International Neural Network Society(2021)
摘要
This study proposes a novel biologically motivated learning method for deep convolutional neural networks (CNNs). The combination of CNNs and backpropagation learning is the most powerful method in recent machine learning regimes. However, it requires a large amount of labeled data for training, and this requirement can occasionally become a barrier for real world applications. To address this problem and use unlabeled data, we introduce unsupervised competitive learning, which only requires forward propagating signals for CNNs. The method was evaluated on image discrimination tasks using the MNIST, CIFAR-10, and ImageNet datasets, and it achieved state-of-the-art performance with respect to other biologically motivated methods in the ImageNet benchmark. The results suggest that the method enables higher-level learning representations solely based on the forward propagating signals without the need for a backward error signal for training convolutional layers. The proposed method could be useful for a variety of poorly labeled data, for example, time series or medical data.
更多查看译文
AI 理解论文
溯源树
样例
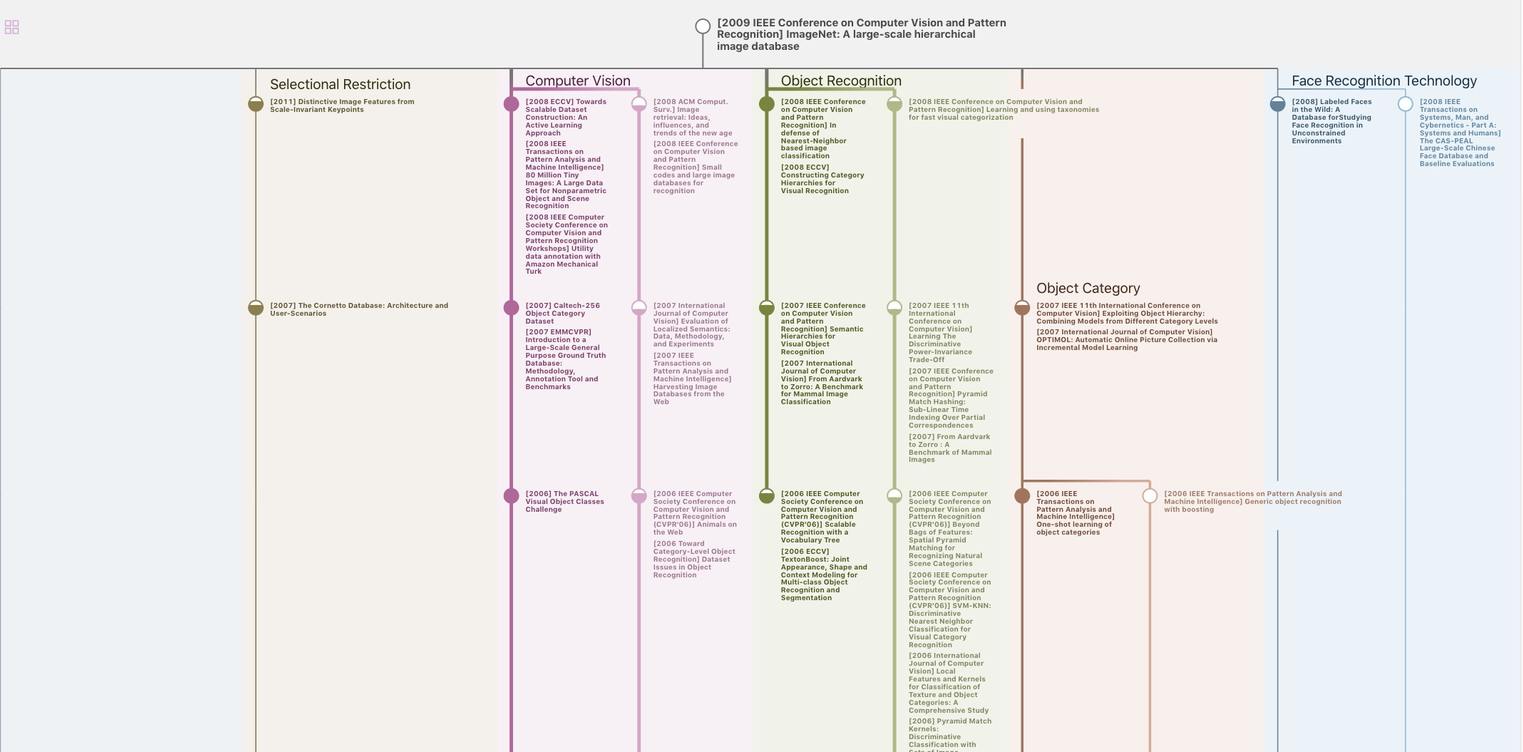
生成溯源树,研究论文发展脉络
Chat Paper
正在生成论文摘要