Exploring machine learning methods for absolute configuration determination with vibrational circular dichroism.
PHYSICAL CHEMISTRY CHEMICAL PHYSICS(2021)
摘要
The added value of supervised Machine Learning (ML) methods to determine the Absolute Configuration (AC) of compounds from their Vibrational Circular Dichroism (VCD) spectra was explored. Among all ML methods considered, Random Forest (RF) and Feedforward Neural Network (FNN) yield the best performance for identification of the AC. At its best, FNN allows near-perfect AC determination, with accuracy of prediction up to 0.995, while RF combines good predictive accuracy (up to 0.940) with the ability to identify the spectral areas important for the identification of the AC. No loss in performance of either model is observed as long as the spectral sampling interval used does not exceed the spectral bandwidth. Increasing the sampling interval proves to be the best method to lower the dimensionality of the input data, thereby decreasing the computational cost associated with the training of the models.
更多查看译文
关键词
vibrational circular dichroism,absolute configuration determination,circular dichroism,machine learning methods,machine learning
AI 理解论文
溯源树
样例
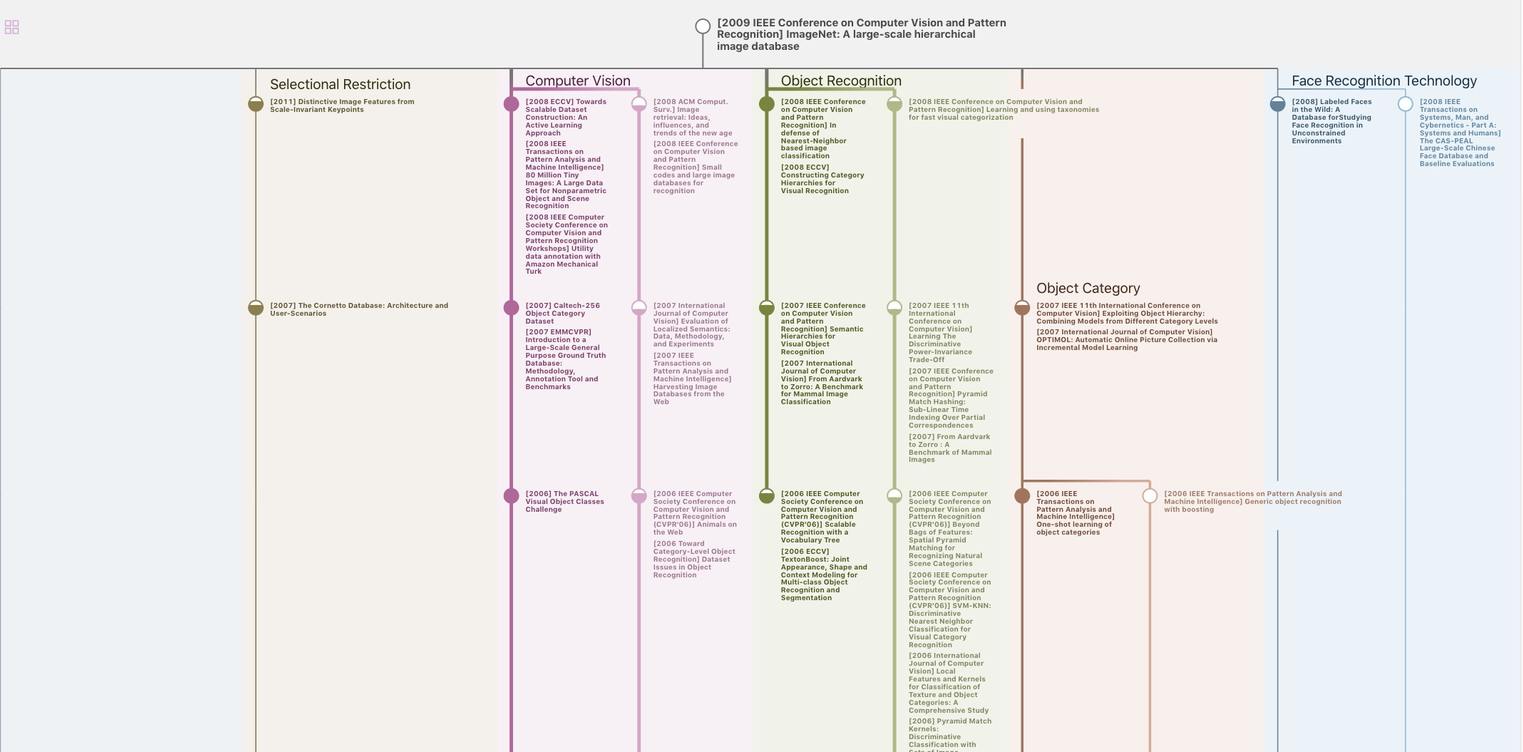
生成溯源树,研究论文发展脉络
Chat Paper
正在生成论文摘要