Weakly Supervised Semantic Segmentation with Patch-Based Metric Learning Enhancement
ARTIFICIAL NEURAL NETWORKS AND MACHINE LEARNING - ICANN 2021, PT III(2021)
摘要
Weakly supervised semantic segmentation (WSSS) methods are more flexible and less costly than supervised ones since no pixel-level annotation is required. Class activation maps (CAMs) are commonly used in existing WSSS methods with image-level annotations to identify seed localization cues. However, as CAMs are obtained from a classification network that mainly focuses on the most discriminative parts of an object, less discriminative parts may be ignored and not identified. This study aims to improve the local visual understanding on objects of the classification network by considering an additional metric learning task on patches sampled from each CAM-based object proposal. As the patches contain different object parts and surrounding backgrounds, not only the most discriminative object parts but the entire objects are learned through leveraging the patch similarity. After the joint training process with the proposed patch-based metric learning and classification tasks, we expect more discriminative local features can be learned by the backbone network. As a result, more complete class-specific regions of an object can be identified. Extensive experiments on the PASCAL VOC 2012 dataset validate the superiority of our method. Our proposed model achieves improvement compared with the state-of-the-art methods.
更多查看译文
关键词
Semantic segmentation, Fully convolutional neural networks, Weakly supervised learning, Metric learning
AI 理解论文
溯源树
样例
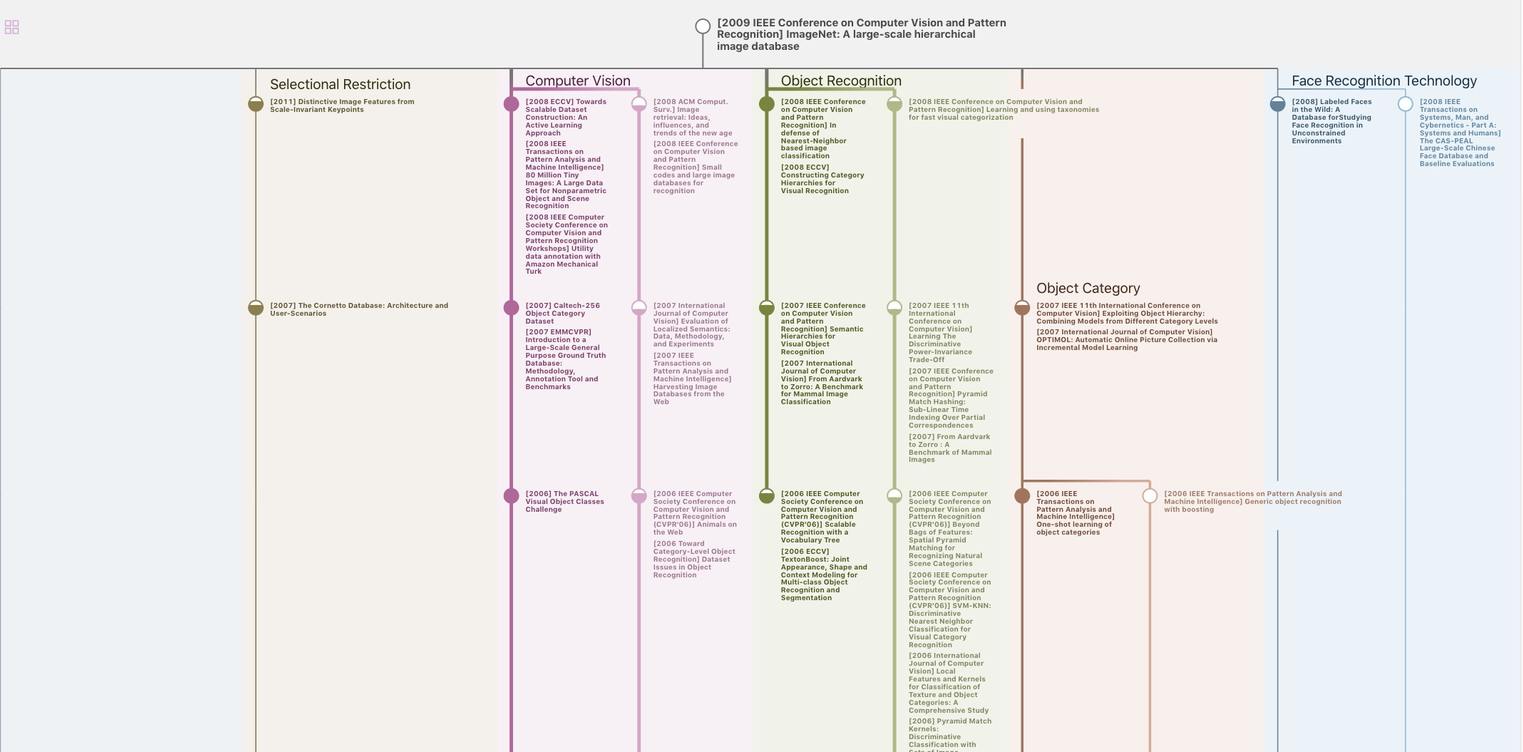
生成溯源树,研究论文发展脉络
Chat Paper
正在生成论文摘要