A Multimode Two-Stream Network for Egocentric Action Recognition
ARTIFICIAL NEURAL NETWORKS AND MACHINE LEARNING - ICANN 2021, PT I(2021)
摘要
Video-based egocentric activity recognition involves spatiotemporal and human-object interaction. With the great success of deep learning technology in image recognition, human activity recognition in videos has got increasing attention in multimedia understanding. Comprehensive visual understanding requires the detection and modeling of individual visual features and the interactions between them. The current popular human action recognition approaches based on the visual features extracted from 2-D images, and therefore often lead to unreliable salient visual feature detection and inaccurate modeling of the interaction context between individual features. In this paper, we show that these problems can be addressed by combining data from images and skeletons. First, we propose a pose-based two-stream network for action recognition that effectively fuses information from both skeleton and image at multiple levels of the video processing pipeline. In our network, one stream models the temporal dynamics of the action-related objects from video frames, and the other stream models the temporal dynamics of the targeted 2D human pose sequences which are extracted from raw video. Moreover, we demonstrate that a ConvNet trained on RGB data is able to achieve good performance in spite of limited training data. Our architecture is trained and evaluated on the standard video actions benchmarks of UCF101-24 and JHMDB, where it is competitive with the state of the art. Among them, we have got the best results currently on the JHMDB, the mAP reached 90.6%.
更多查看译文
关键词
Action recognition,Multimodal fusion,Self-attention,Two-stream network
AI 理解论文
溯源树
样例
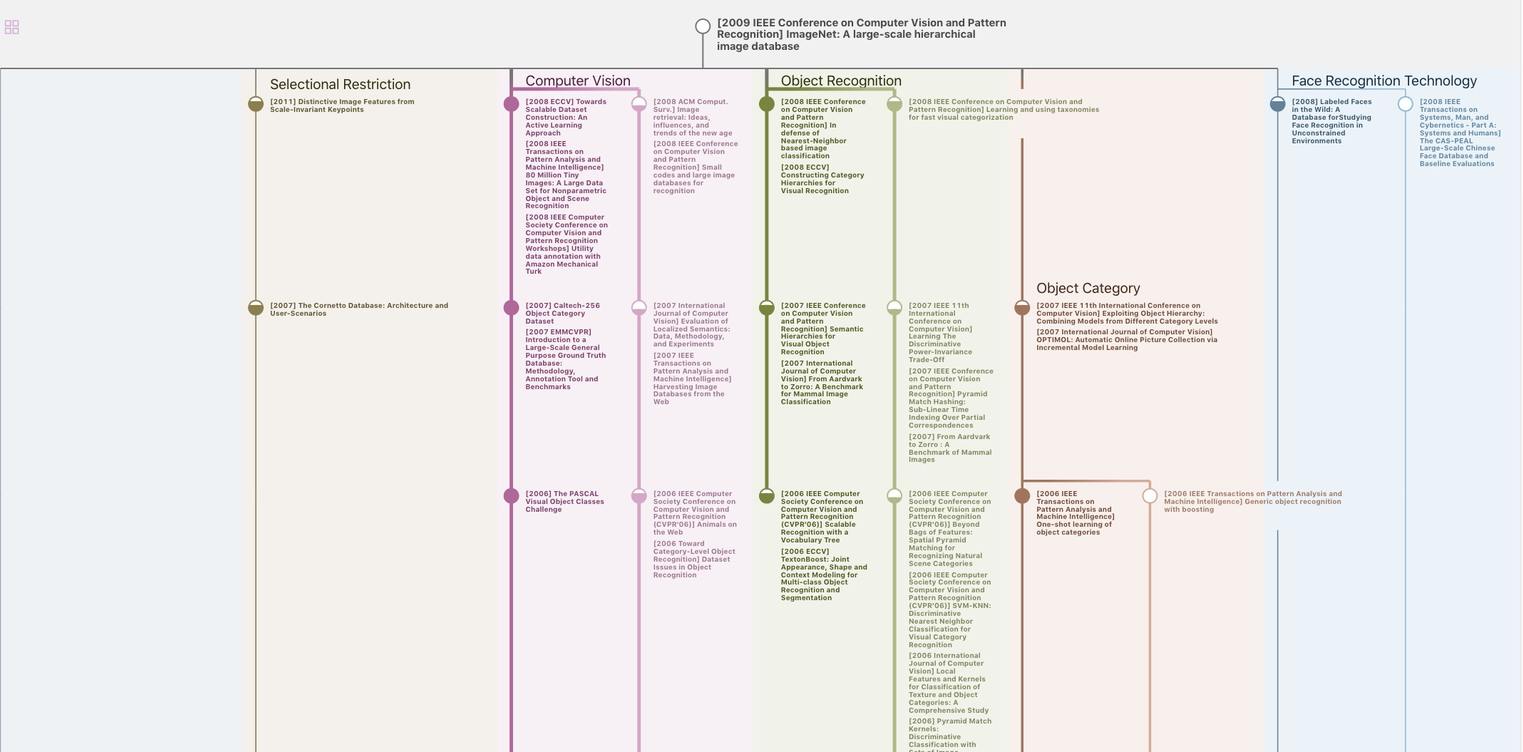
生成溯源树,研究论文发展脉络
Chat Paper
正在生成论文摘要