A Blockchain Based Decentralized Gradient Aggregation Design for Federated Learning
ARTIFICIAL NEURAL NETWORKS AND MACHINE LEARNING - ICANN 2021, PT II(2021)
摘要
Based on the concept of letting training organizations only exchange their partial gradients instead of the proprietary datasets owned by them, federated learning has become a promising approach for organizations to train deep learning models collaboratively. However, conventional federated learning based on a centralized parameter server is susceptible to "recovery" attacks, in which the original data can be recovered if the attacker can collect enough gradients from the organizations. To solve the problem, we first propose a blockchain-based decentralized model training architecture for federated learning, which is more robust than the centralized architecture. Based on this architecture, we develop a joint efficiency and randomness aware gradient aggregation approach. Our real-world experiments show that our design is not affected by a single point of failure. Moreover, it can increase the model accuracy of the participating organization, while mitigating the data privacy disclosure risk and improving the gradient aggregation performance.
更多查看译文
关键词
Federated learning, Blockchain, Smart contract
AI 理解论文
溯源树
样例
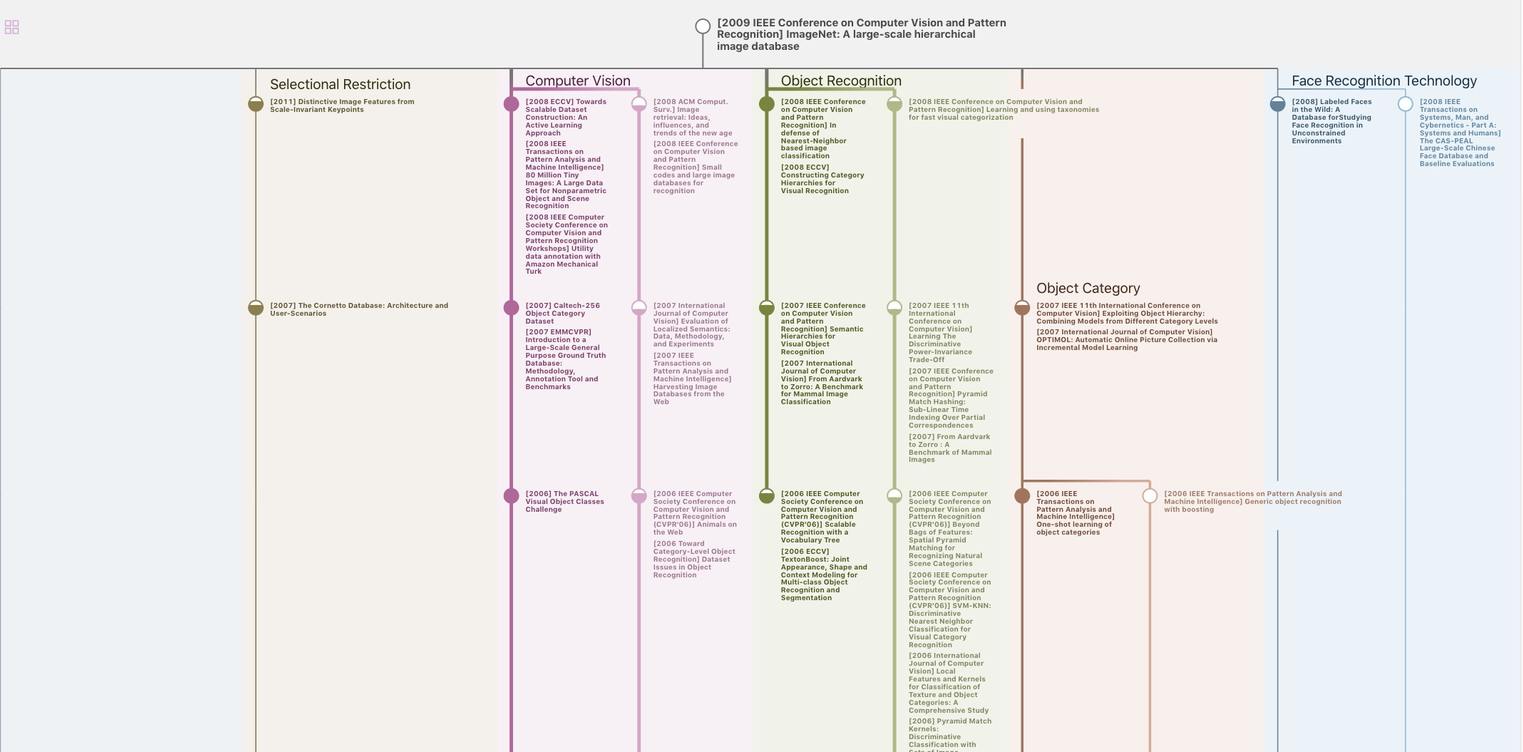
生成溯源树,研究论文发展脉络
Chat Paper
正在生成论文摘要