Evaluate Pseudo Labeling and CNN for Multi-variate Time Series Classification in Low-Data Regimes
ARTIFICIAL NEURAL NETWORKS AND MACHINE LEARNING, ICANN 2021, PT V(2021)
摘要
Nowadays, huge amount of data are being produced by a large and diverse family of sensors (e.g., remote sensors, biochemical sensors, wearable devices). These sensors typically measure multiple variables over time, resulting in data streams that can be profitably organized as multivariate time-series. In practical scenarios, the speed at which such information is collected often makes the data labeling a difficult task. This results in a low-data regime scenario where only a small set of labeled samples is available and standard supervised learning algorithms cannot be employed. To cope with the task of multi-variate time series classification in low-data regime scenarios, here, we propose a framework that combines convolutional neural networks (CNNs) with self-training (pseudo labeling) in a transductive setting (test data are already available at training time). Our framework, named ResNetIPL, wraps a CNN based classifier into an iterative procedure that, at each step, enlarges the training set with new samples and their associated pseudo labels. An experimental evaluation on several benchmarks, coming from different domains, has demonstrated the value of the proposed approach and, more generally, the ability of the deep learning approaches to effectively deal with scenarios characterized by low-data regimes.
更多查看译文
关键词
pseudo labeling,classification,cnn,multi-variate,low-data
AI 理解论文
溯源树
样例
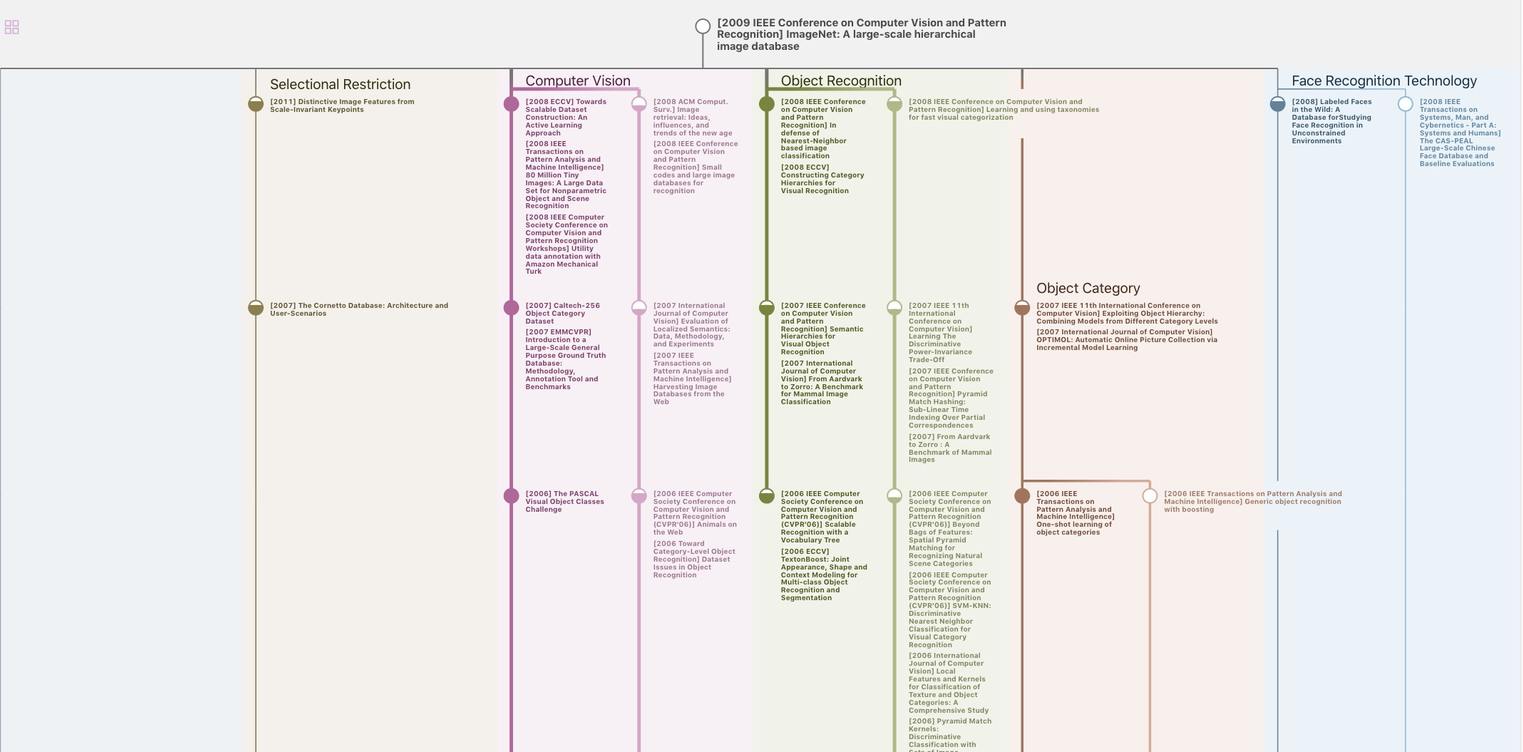
生成溯源树,研究论文发展脉络
Chat Paper
正在生成论文摘要