Computationally-Efficient Climate Predictions using Multi-Fidelity Surrogate Modelling
arxiv(2021)
摘要
Accurately modelling the Earth's climate has widespread applications ranging from forecasting local weather to understanding global climate change. Low-fidelity simulations of climate phenomena are readily available, but high-fidelity simulations are expensive to obtain. We therefore investigate the potential of Gaussian process-based multi-fidelity surrogate modelling as a way to produce high-fidelity climate predictions at low cost. Specifically, our model combines the predictions of a low-fidelity Global Climate Model (GCM) and those of a high-fidelity Regional Climate Model (RCM) to produce high-fidelity temperature predictions for a mountainous region on the coastline of Peru. We are able to produce high-fidelity temperature predictions at significantly lower computational cost compared to the high-fidelity model alone: our predictions have an average error of $15.62^\circ\text{C}^2$ yet our approach only evaluates the high-fidelity model on 6% of the region of interest.
更多查看译文
关键词
climate,modelling,computationally-efficient,multi-fidelity
AI 理解论文
溯源树
样例
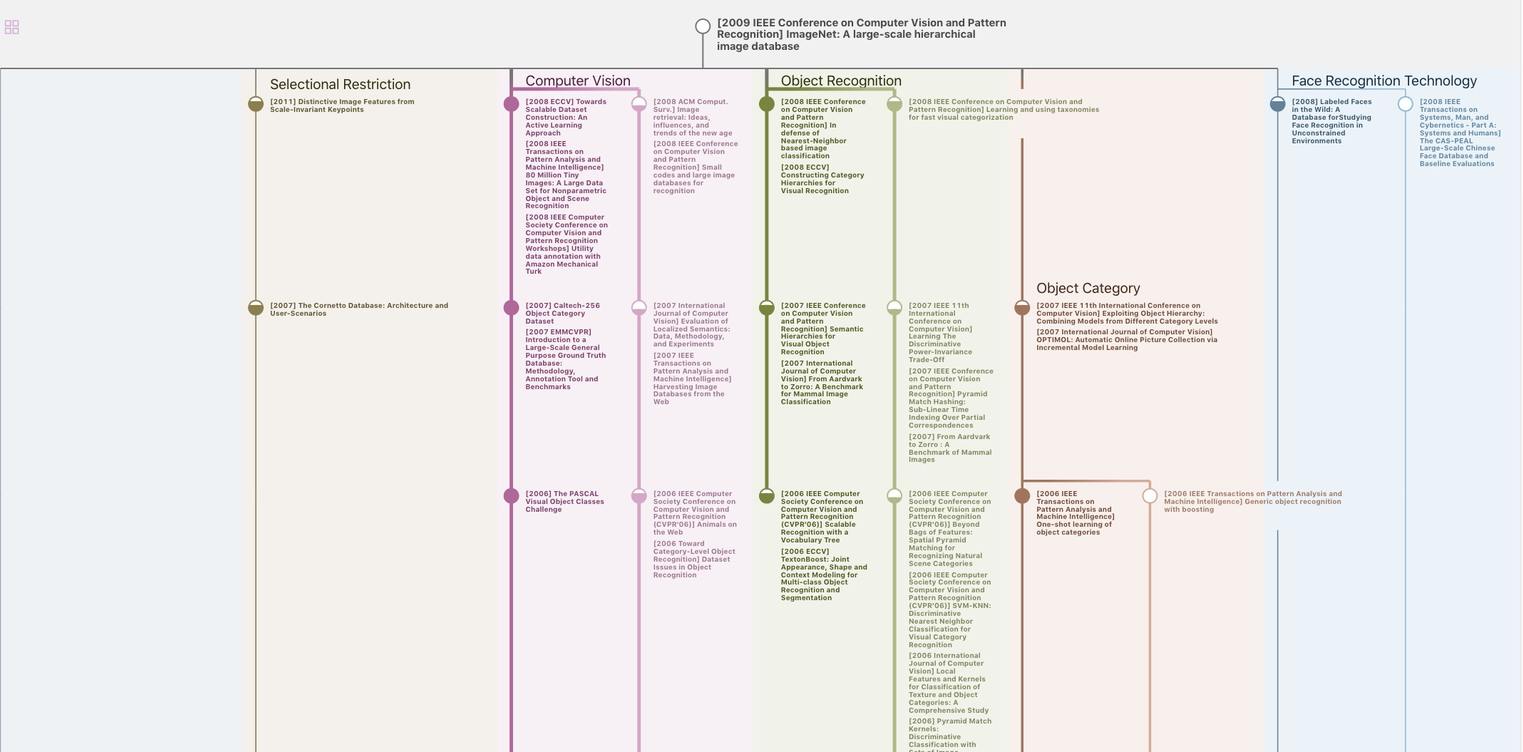
生成溯源树,研究论文发展脉络
Chat Paper
正在生成论文摘要