Understanding the role of human-inspired heuristics for retrieval models
Frontiers of Computer Science(2021)
摘要
Relevance estimation is one of the core concerns of information retrieval (IR) studies. Although existing retrieval models gained much success in both deepening our understanding of information seeking behavior and building effective retrieval systems, we have to admit that the models work in a rather different manner from how humans make relevance judgments. Users’ information seeking behaviors involve complex cognitive processes, however, the majority of these behavior patterns are not considered in existing retrieval models. To bridge the gap between practical user behavior and retrieval model, it is essential to systematically investigate user cognitive behavior during relevance judgement and incorporate these heuristics into retrieval models. In this paper, we aim to formally define a set of basic user reading heuristics during relevance judgement and investigate their corresponding modeling strategies in retrieval models. Further experiments are conducted to evaluate the effectiveness of different reading heuristics for improving ranking performance. Based on a large-scale Web search dataset, we find that most reading heuristics can improve the performance of retrieval model and establish guidelines for improving the design of retrieval models with human-inspired heuristics. Our study sheds light on building retrieval model from the perspective of cognitive behavior.
更多查看译文
关键词
reading heuristics,retrieval model,cognitive behavior
AI 理解论文
溯源树
样例
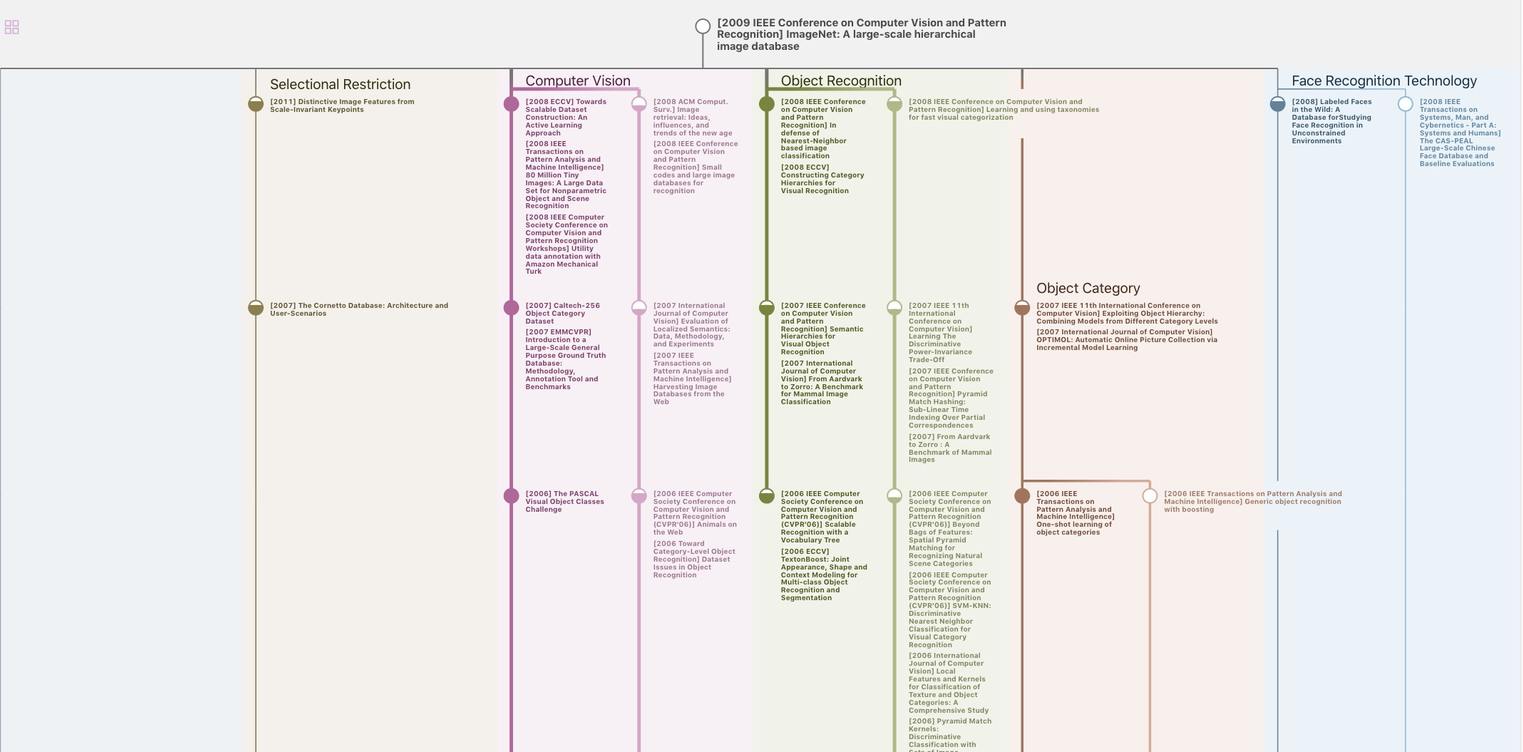
生成溯源树,研究论文发展脉络
Chat Paper
正在生成论文摘要