Using Random Effect Models to Produce Robust Estimates of Death Rates in COVID-19 Data
International journal of environmental research and public health/International journal of environmental research and public health(2022)
摘要
Tracking the progress of an infectious disease is critical during a pandemic. However, the incubation period, diagnosis, and treatment most often cause uncertainties in the reporting of both cases and deaths, leading in turn to unreliable death rates. Moreover, even if the reported counts were accurate, the “crude” estimates of death rates which simply divide country-wise reported deaths by case numbers may still be poor or even non-computable in the presence of small (or zero) counts. We present a novel methodological contribution which describes the problem of analyzing COVID-19 data by two nested Poisson models: (i) an “upper model” for the cases infected by COVID-19 with an offset of population size, and (ii) a “lower” model for deaths of COVID-19 with the cases infected by COVID-19 as an offset, each equipped with their own random effect. This approach generates robustness in both the numerator as well as the denominator of the estimated death rates to the presence of small or zero counts, by “borrowing” information from other countries in the overall dataset, and guarantees positivity of both the numerator and denominator. The estimation will be carried out through non-parametric maximum likelihood which approximates the random effect distribution through a discrete mixture. An added advantage of this approach is that it allows for the detection of latent subpopulations or subgroups of countries sharing similar behavior in terms of their death rates.
更多查看译文
关键词
count data,Poisson model,death rates,case rates,robustness,random effects,mixture model,EM algorithm,shrinkage,MAP rule,COVID-19
AI 理解论文
溯源树
样例
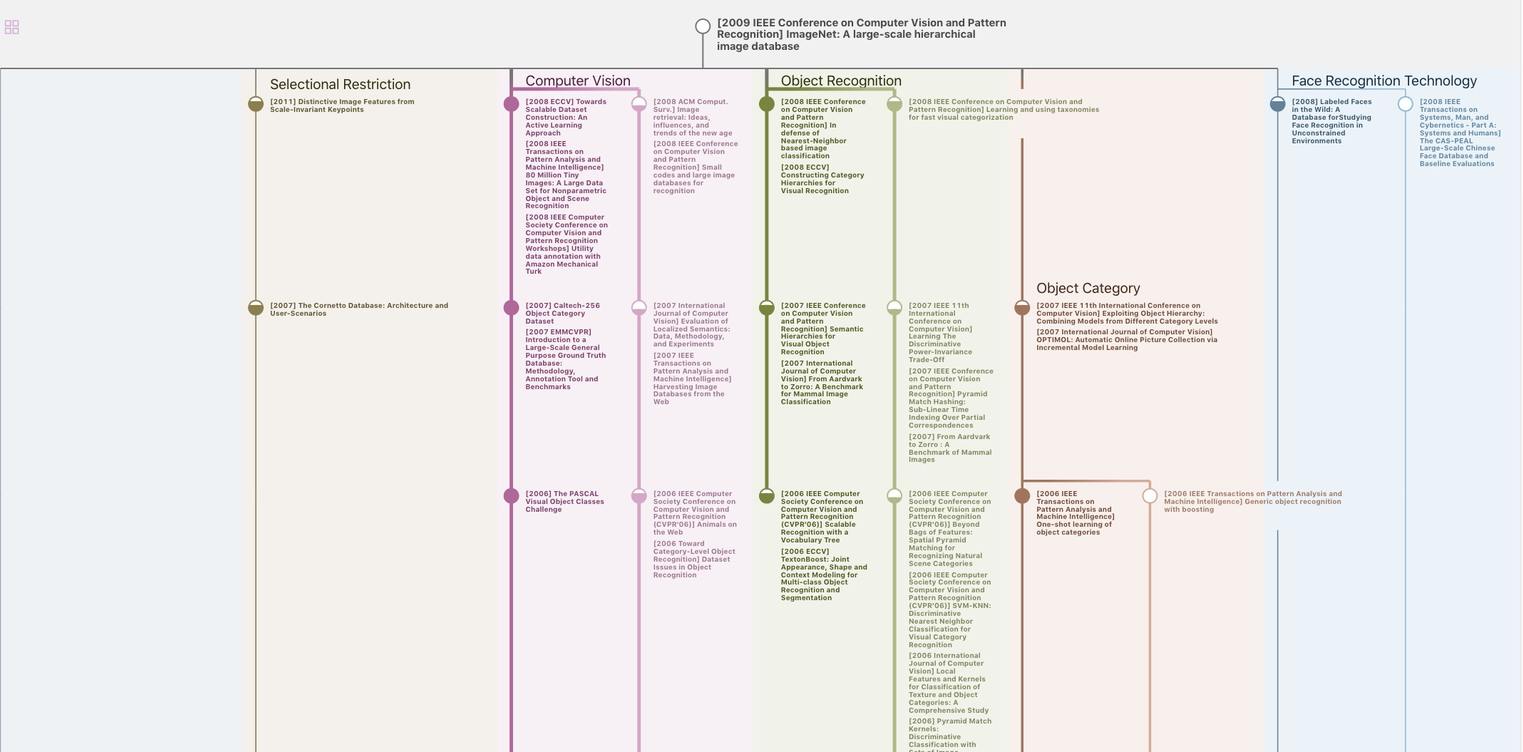
生成溯源树,研究论文发展脉络
Chat Paper
正在生成论文摘要