Echocardiogram Segmentation Using Active Shape Model And Mean Squared Eigenvalue Error
BIOMEDICAL SIGNAL PROCESSING AND CONTROL(2021)
摘要
Cardiac function assessment using echocardiography is an essential step in daily cardiology. For this purpose, segmentation of images and, in particular, ventricle boundary segmentation poses several challenges due to shadows and speckle noise. Currently, the segmentation is done manually which is time-consuming and subjective. Therefore, providing an efficient and autonomous segmentation method could improve the evaluation efficiency and outcome. The focus of this paper is on active shape models (ASMs) to handle speckle noise, shadows, and dropouts in echocardiograms effectively, without the need for large training data. In this paper, an echocardiogram segmentation method using ASM, Nakagami and mean squared eigenvalues error (MSEE) is proposed. We propose the use of Nakagami distribution in providing a preprocess classification and hence improving the system performance. Additionally, we depart from the current practice in using (and manually entering) a percentage of eigenvalues in an appearance model of ASM. We show that the higher the percentage does not necessarily mean the better the performance. By integrating a mean squared error (MSE) evaluation method, we automate the choice of that percentage to warrant the minimum MSE. The simulation results are provided to show the effectiveness of the approach. In particular, we illustrate that using Nakagami would reduce the segmentation error. Also mean squared eigenvalues error (MSEE) will reduce the required total variance per landmark, and hence, the memory requirement will be decreased. Also, the proposed technique will provide the optimal total variance for each landmark, resulting in improved accuracy. The final results from segmentation ASM with Nakagami and MSEE illustrate a considerable reduction of the MSE error from 14.521 +/- 7.36 in original ASM to 9.21 +/- 3.85 pixels, and produced an average of 0.93 +/- 0.02 DSI with insignificant additional processing time.
更多查看译文
关键词
Echocardiography, Segmentation, Active shape models
AI 理解论文
溯源树
样例
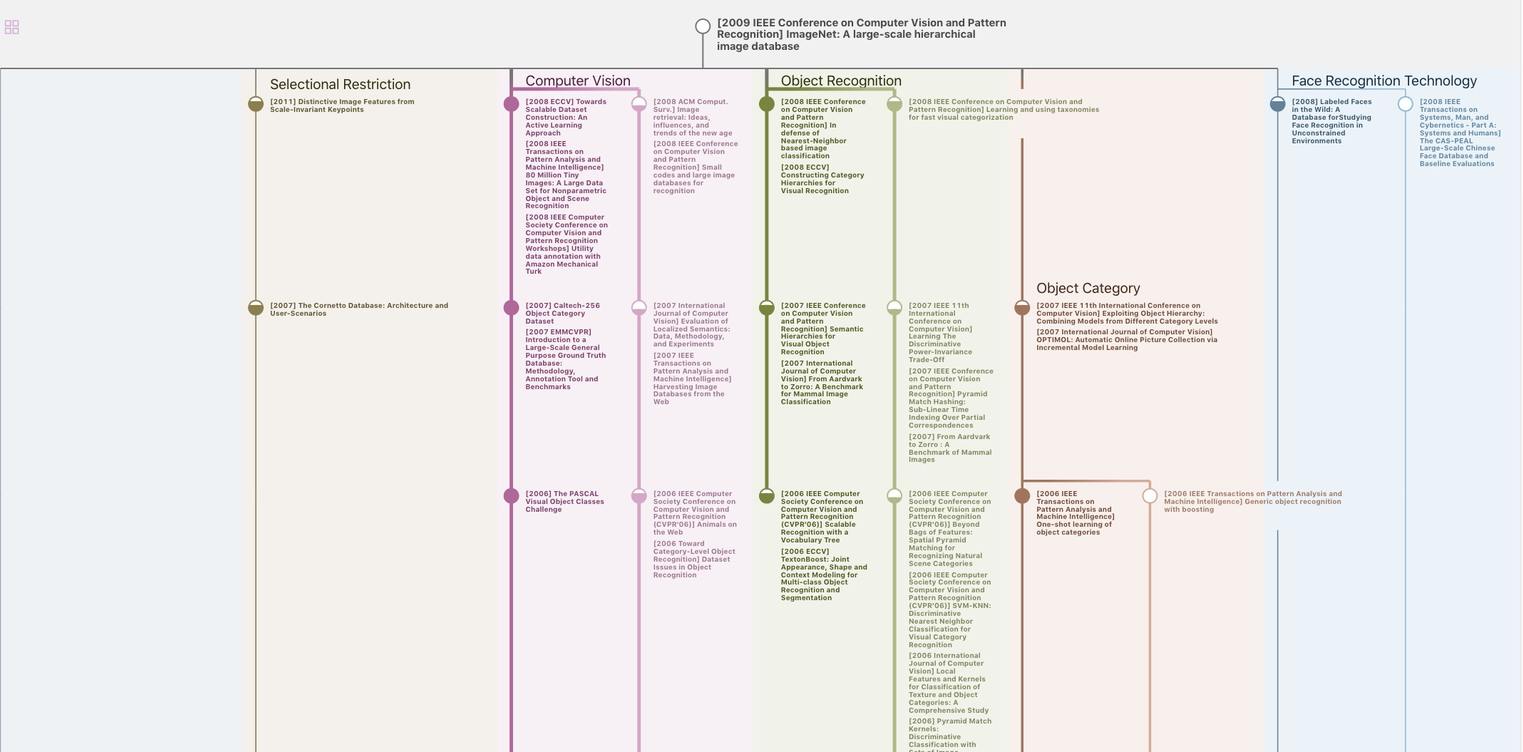
生成溯源树,研究论文发展脉络
Chat Paper
正在生成论文摘要