Personalized Vital Signs Control Based On Continuous Action-Space Reinforcement Learning With Supervised Experience
BIOMEDICAL SIGNAL PROCESSING AND CONTROL(2021)
摘要
Vital signs reflect patients' current health status. Different patients are suitable for distinct references of vital signs due to the disease type and individual physique. Personalized Vital Signs Control (PVSC) helps clinicians with the recommendation of the optimal references for real-time treatment. However, a data-driven approach needs to overcome the unclear ground truth problem, requires complex feature integration and continuous value space, and is expected to ensure the safety of fragile patients. But none of the existing approaches can overcome all of these challenges simultaneously. This work emphasizes PVSC as a sequence decision-making problem and applies multiple reinforcement learning methods to it. We propose a novel adaptive medical control model. The model combines the deep deterministic policy gradient reinforcement learning algorithm, supervised experience knowledge, and recurrent neural network module, named PVSC-RL. We test the model on a real medical database, MIMIC-III, with 15,232 sepsis and 13,608 heart failure records. Experimental results show that using the policy of PVSC-RL, the survival rate of sepsis and heart failure patients by blood glucose control and blood pressure control can be increased by 3.45%, 15.21%, 4.19%, 12.13%. Meanwhile, the safety rate is also be increased by 3.09%, 1.02%, 3.54%, 4.04%.
更多查看译文
关键词
Personalized vital signs control, Clinical decision support, Safety control, Reinforcement learning, Supervised learning
AI 理解论文
溯源树
样例
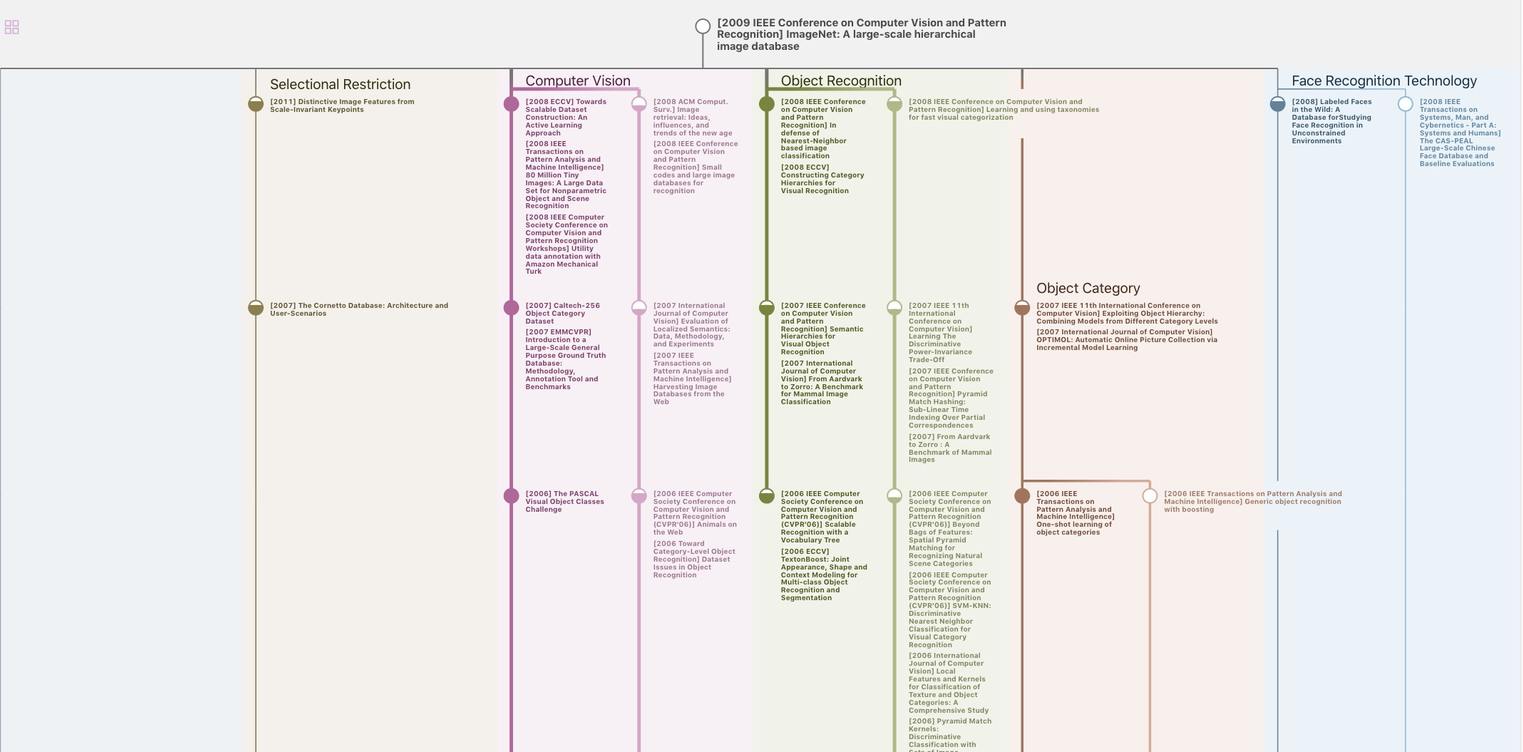
生成溯源树,研究论文发展脉络
Chat Paper
正在生成论文摘要