Hybrid Variational Autoencoder for Recommender Systems
ACM Transactions on Knowledge Discovery from Data(2022)
摘要
AbstractE-commerce platforms heavily rely on automatic personalized recommender systems, e.g., collaborative filtering models, to improve customer experience. Some hybrid models have been proposed recently to address the deficiency of existing models. However, their performances drop significantly when the dataset is sparse. Most of the recent works failed to fully address this shortcoming. At most, some of them only tried to alleviate the problem by considering either user side or item side content information. In this article, we propose a novel recommender model called Hybrid Variational Autoencoder (HVAE) to improve the performance on sparse datasets. Different from the existing approaches, we encode both user and item information into a latent space for semantic relevance measurement. In parallel, we utilize collaborative filtering to find the implicit factors of users and items, and combine their outputs to deliver a hybrid solution. In addition, we compare the performance of Gaussian distribution and multinomial distribution in learning the representations of the textual data. Our experiment results show that HVAE is able to significantly outperform state-of-the-art models with robust performance.
更多查看译文
关键词
Recommender systems, hybrid filtering
AI 理解论文
溯源树
样例
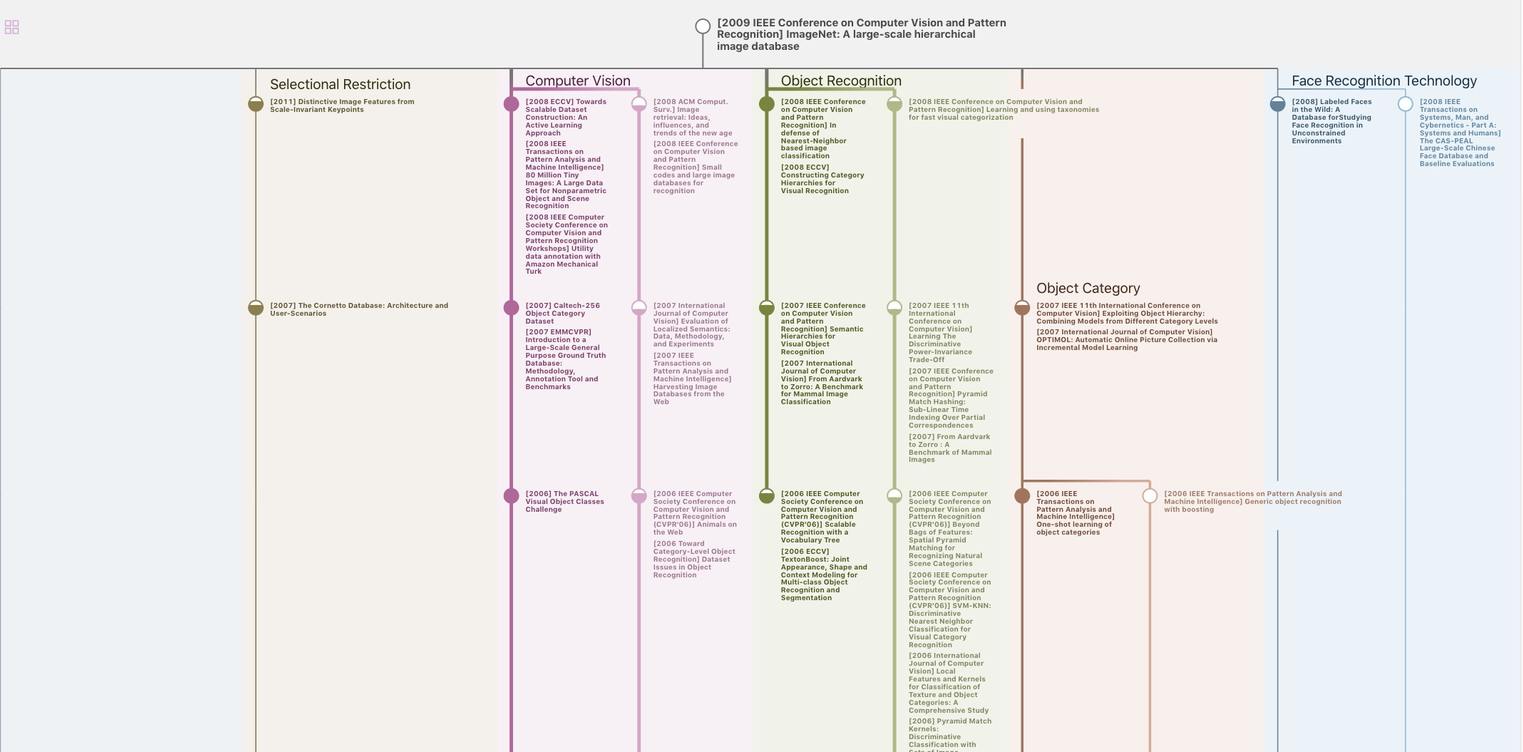
生成溯源树,研究论文发展脉络
Chat Paper
正在生成论文摘要