Robust Selection Stability Estimation in Correlated Spaces
MACHINE LEARNING AND KNOWLEDGE DISCOVERY IN DATABASES, ECML PKDD 2021: RESEARCH TRACK, PT III(2021)
摘要
The stability of feature selection refers to the variability of the selected feature sets induced by small changes of data sampling or analysis pipeline. Instability may strongly limit a sound interpretation of the selected features by domain experts. This work addresses the problem of assessing stability in the presence of correlated features. Correctly measuring selection stability in this context amounts to estimate to which extent several correlated variables contribute to predictive models, and how such contributions may change with the data sampling. We propose here a novel stability index taking into account such multivariate contributions. The shared contributions of several variables to predictive models do not only depend on the possible correlations between them. Computing this stability index therefore requires to solve a weighted bipartite matching problem to discover which variables actually share such contributions. We demonstrate that our novel approach provides more robust stability estimates than current measures, including existing ones taking into account feature correlations. The benefits of the proposed approach are demonstrated on simulated and real data, including microarray and mass spectrometry datasets. The code and datasets used in this paper are publicly available: https://github.com/hamerv/ecml21.
更多查看译文
关键词
robust selection stability estimation,correlated
AI 理解论文
溯源树
样例
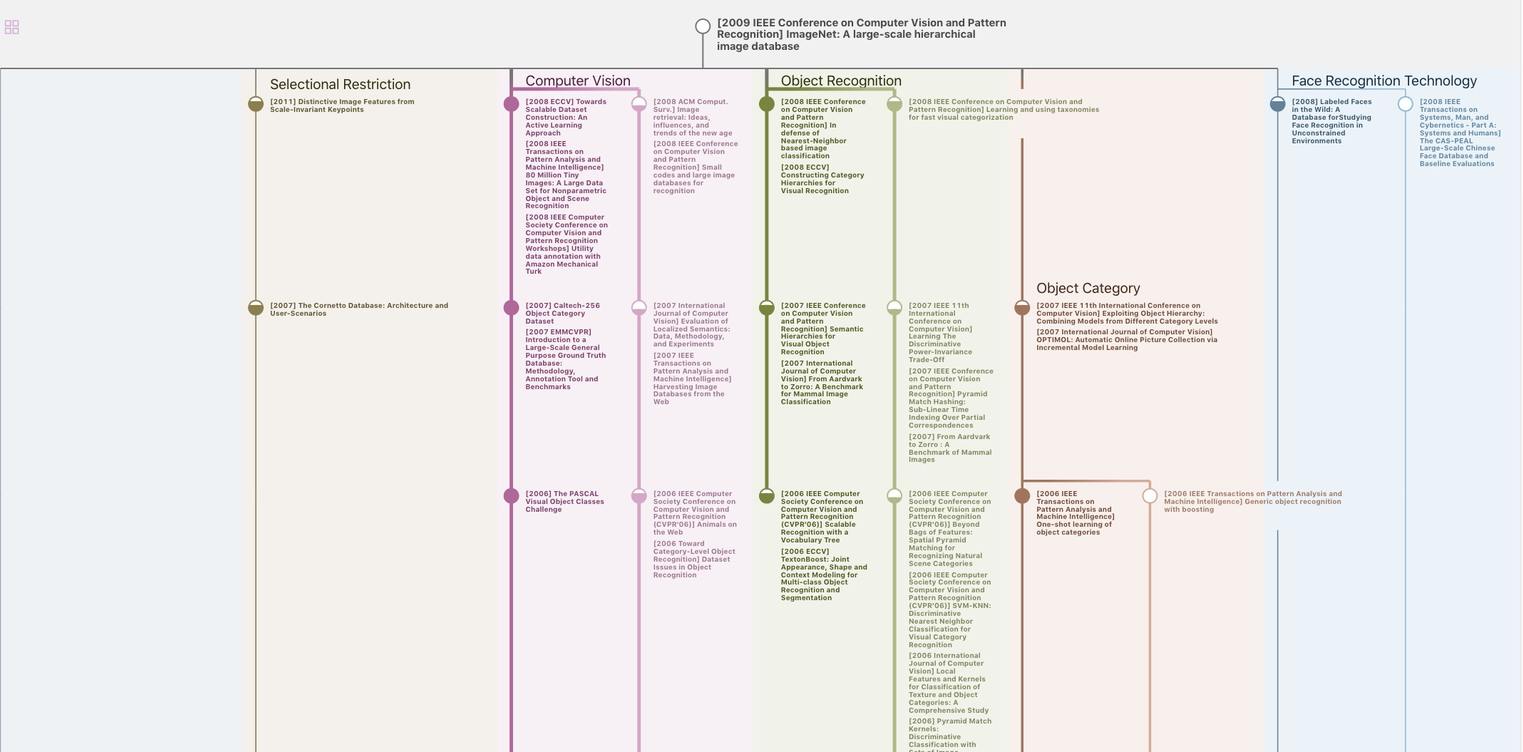
生成溯源树,研究论文发展脉络
Chat Paper
正在生成论文摘要