Semi-supervised Semantic Visualization for Networked Documents
MACHINE LEARNING AND KNOWLEDGE DISCOVERY IN DATABASES, ECML PKDD 2021: RESEARCH TRACK, PT III(2021)
摘要
Semantic interpretability and visual expressivity are important objectives in exploratory analysis of text. On the one hand, while some documents may have explicit categories, we could develop a better understanding of a corpus by studying its finer-grained structures, which may be latent. By inferring latent topics and discovering keywords associated with each topic, one obtains a semantic interpretation of the corpus. One the other hand, by visualizing documents, latent topics, and category labels on the same plot, one gains a bird's eye view of the relationships among documents, topics, and various categories. Semantic visualization is a class of methods that unify both topic modeling and visualization. In this paper, we propose a novel semantic visualization model for networked documents that incorporates partial labels. We introduce coordinate-based label distribution and label-dependent topic distribution to visualize documents, topics, and labels in a semi-supervised way. We further derive three variants for singly-labeled, multi-labeled, and hierarchically-labeled documents. The focus on semi-supervision that employs variants of labeling structures is particularly novel. Experiments verify the efficacy of our model against baselines.
更多查看译文
关键词
Semantic visualization, Topic modeling, Dimensionality reduction, Generative models
AI 理解论文
溯源树
样例
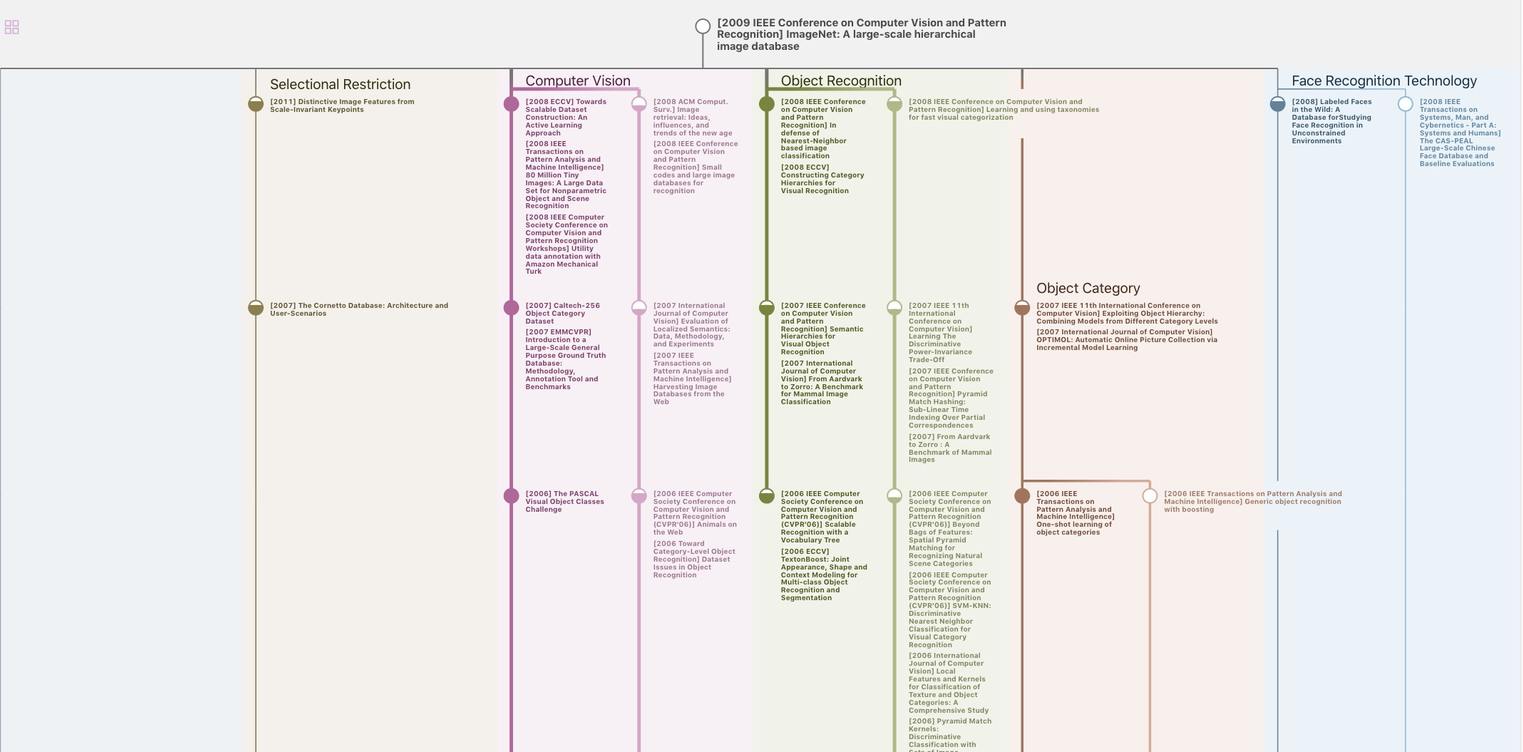
生成溯源树,研究论文发展脉络
Chat Paper
正在生成论文摘要