Multi-agent Deep Reinforcement Learning with Spatio-Temporal Feature Fusion for Traffic Signal Control
MACHINE LEARNING AND KNOWLEDGE DISCOVERY IN DATABASES, ECML PKDD 2021: APPLIED DATA SCIENCE TRACK, PT IV(2021)
摘要
Traffic signal control (TSC) plays an important role in intelligent transportation system. It is helpful to improve the efficiency of urban transportation by controlling the traffic signal intelligently. Recently, various deep reinforcement learning methods have been proposed to solve TSC. However, most of these methods ignore the fusion of spatial and temporal features in traffic roadnets. Besides, these methods pay no attention to the correlations of the intersections in several local areas. This paper proposes a novel multi-agent deep reinforcement learning method with spatio-temporal feature fusion to solve TSC. The proposed method firstly calculates the correlations among different time steps to capture their temporal dependencies. Secondly, the proposed method constructs connected subnetworks to capture interactive relations among intersections in the subnetwork. Experimental results demonstrate that our method achieves state-of-the-art performance on synthetic and real-world datasets.
更多查看译文
关键词
Traffic signal control, Multi-agent deep reinforcement learning, Spatio-temporal feature fusion
AI 理解论文
溯源树
样例
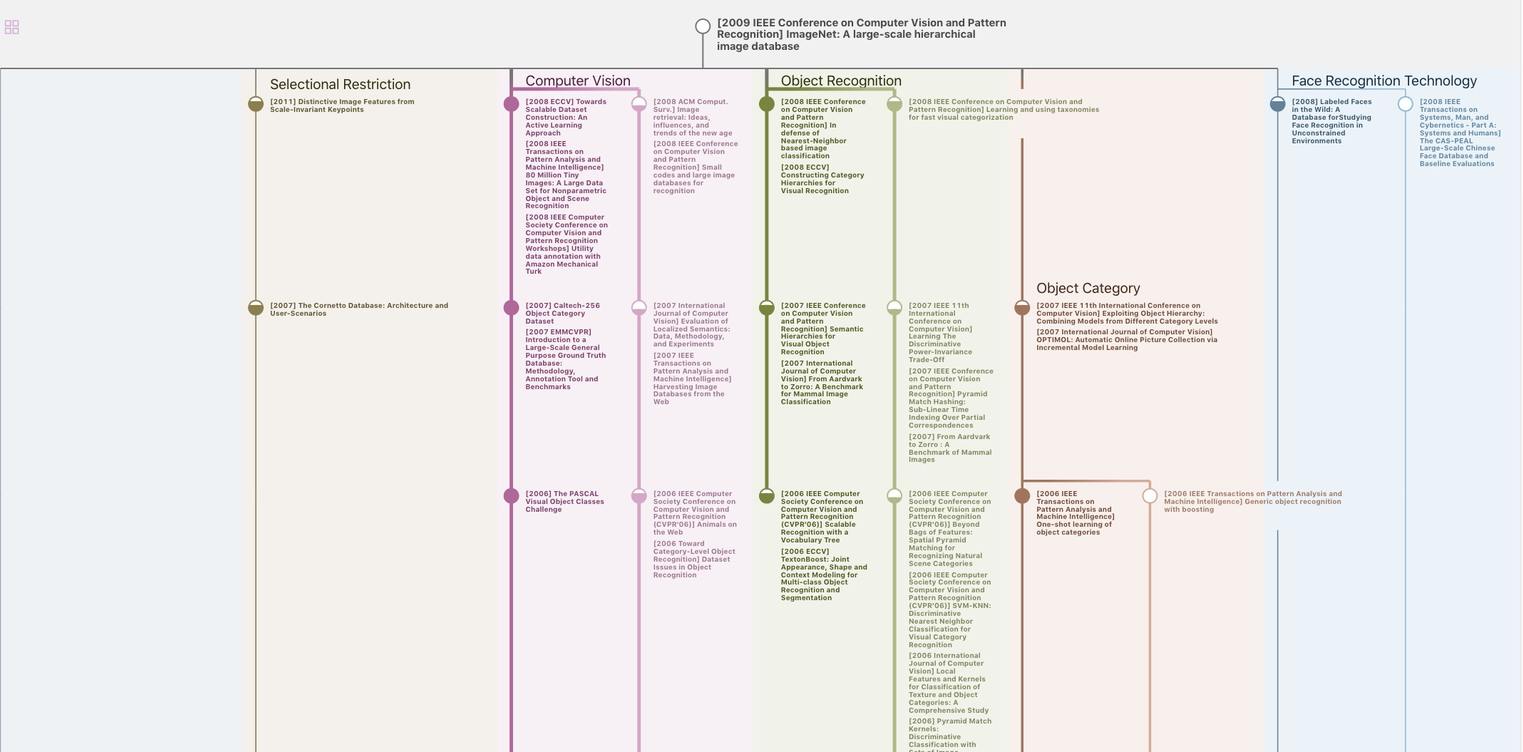
生成溯源树,研究论文发展脉络
Chat Paper
正在生成论文摘要