Knowledge Distillation with Distribution Mismatch
MACHINE LEARNING AND KNOWLEDGE DISCOVERY IN DATABASES, ECML PKDD 2021: RESEARCH TRACK, PT II(2021)
摘要
Knowledge distillation (KD) is one of the most efficient methods to compress a large deep neural network (called teacher) to a smaller network (called student). Current state-of-the-art KD methods assume that the distributions of training data of teacher and student are identical to maintain the student's accuracy close to the teacher's accuracy. However, this strong assumption is not met in many real-world applications where the distribution mismatch happens between teacher's training data and student's training data. As a result, existing KD methods often fail in this case. To overcome this problem, we propose a novel method for KD process, which is still effective when the distribution mismatch happens. We first learn a distribution based on student's training data, from which we can sample images well-classified by the teacher. By doing this, we can discover the data space where the teacher has good knowledge to transfer to the student. We then propose a new loss function to train the student network, which achieves better accuracy than the standard KD loss function. We conduct extensive experiments to demonstrate that our method works well for KD tasks with or without distribution mismatch. To the best of our knowledge, our method is the first method addressing the challenge of distribution mismatch when performing KD process.
更多查看译文
关键词
Knowledge distillation, Model compression, Distribution mismatch, Distribution shift, Mismatched teacher
AI 理解论文
溯源树
样例
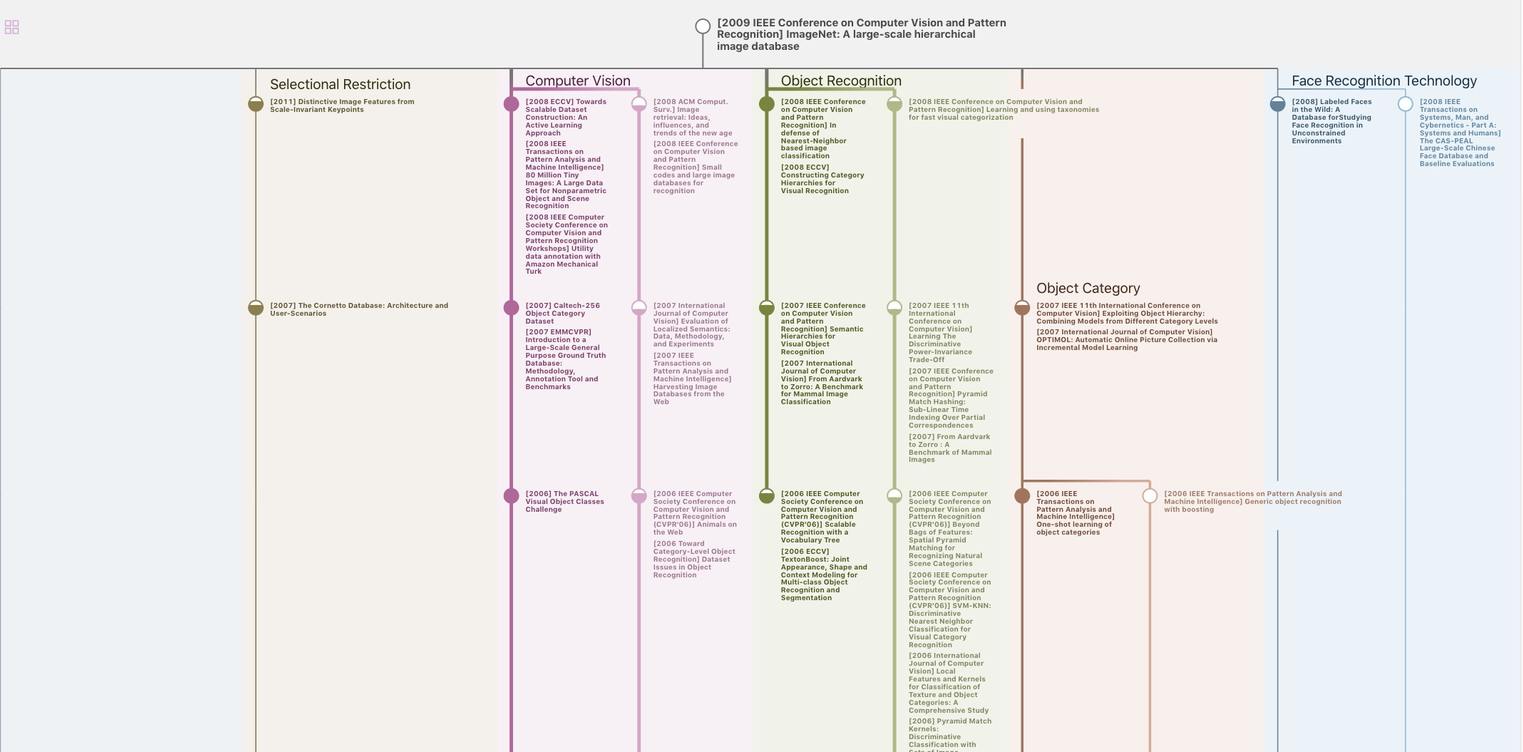
生成溯源树,研究论文发展脉络
Chat Paper
正在生成论文摘要