Sparse Factorization of Large Square Matrices
CoRR(2021)
摘要
Square matrices appear in many machine learning problems and models. Optimization over a large square matrix is expensive in memory and in time. Therefore an economic approximation is needed. Conventional approximation approaches factorize the square matrix into a number matrices of much lower ranks. However, the low-rank constraint is a performance bottleneck if the approximated matrix is intrinsically high-rank or close to full rank. In this paper, we propose to approximate a large square matrix with a product of sparse full-rank matrices. In the approximation, our method needs only $N(\log N)^2$ non-zero numbers for an $N\times N$ full matrix. We present both non-parametric and parametric ways to find the factorization. In the former, we learn the factorizing matrices directly, and in the latter, we train neural networks to map input data to the non-zero matrix entries. The sparse factorization method is tested for a variety of synthetic and real-world square matrices. The experimental results demonstrate that our method gives a better approximation when the approximated matrix is sparse and high-rank. Based on this finding, we use our parametric method as a scalable attention architecture that performs strongly in learning tasks for long sequential data and defeats Transformer and its several variants.
更多查看译文
AI 理解论文
溯源树
样例
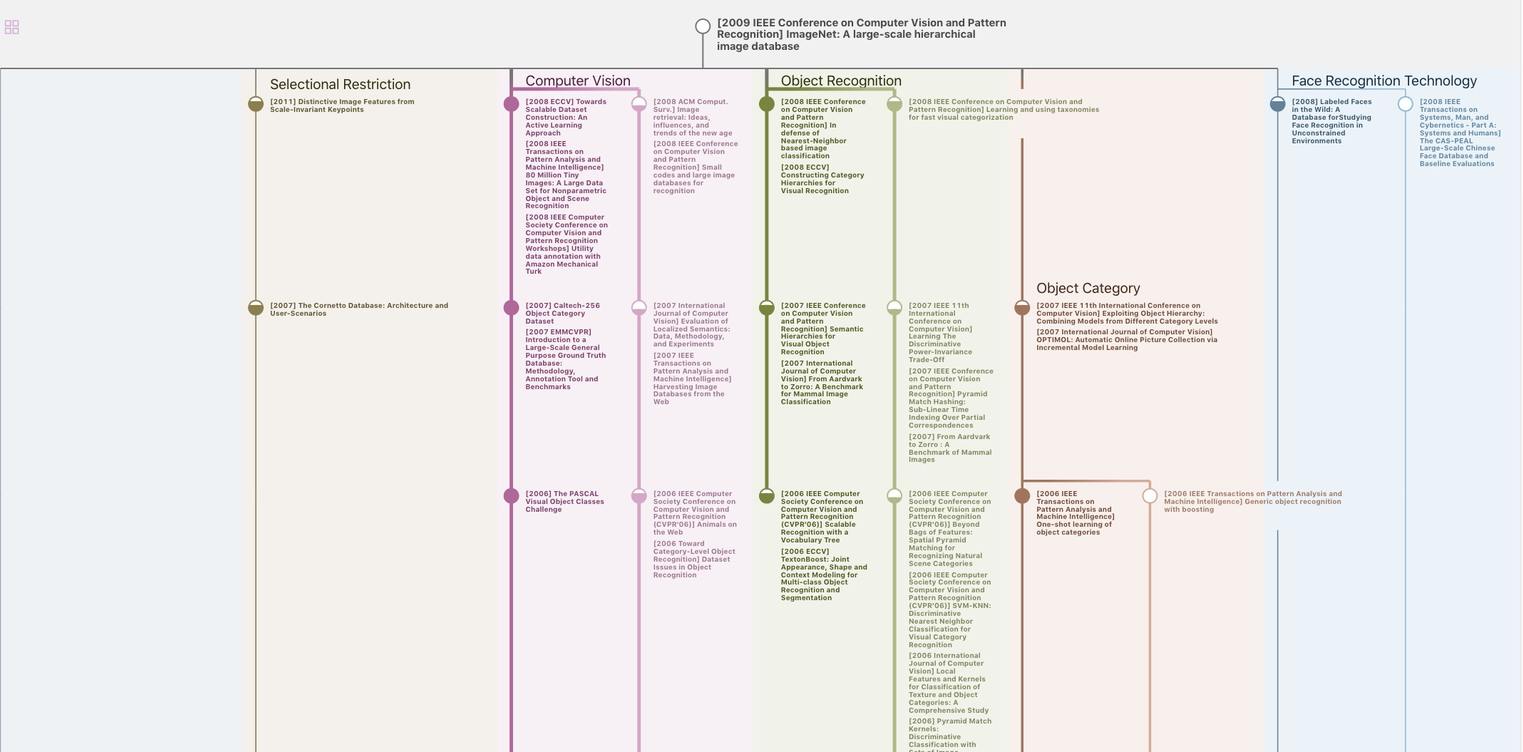
生成溯源树,研究论文发展脉络
Chat Paper
正在生成论文摘要