Asymptotic Optimality for Decentralised Bandits
SIGMETRICS Performance Evaluation Review(2022)
摘要
We consider a large number of agents collaborating on a multi-armed bandit problem with a large number of arms. The goal is to minimise the regret of each agent in a communication-constrained setting. We present a decentralised algorithm which builds upon and improves the Gossip-Insert-Eliminate method of Chawla et al. (International conference on artificial intelligence and statistics, pp 3471–3481, 2020). We provide a theoretical analysis of the regret incurred which shows that our algorithm is asymptotically optimal. In fact, our regret guarantee matches the asymptotically optimal rate achievable in the full communication setting. Finally, we present empirical results which support our conclusions.
更多查看译文
关键词
decentralised bandits,asymptotic optimality
AI 理解论文
溯源树
样例
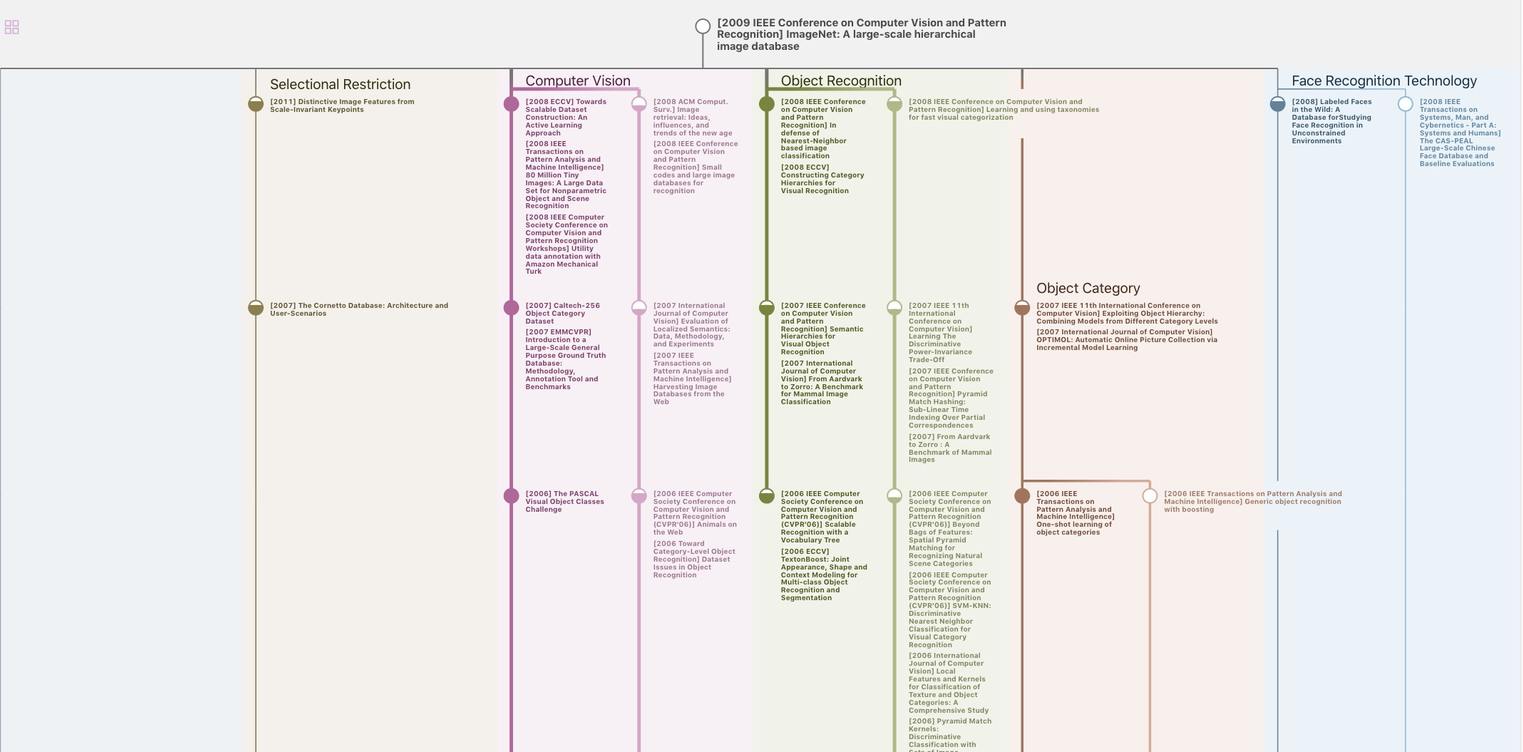
生成溯源树,研究论文发展脉络
Chat Paper
正在生成论文摘要