Model-Agnostic Explanations Using Minimal Forcing Subsets
2021 INTERNATIONAL JOINT CONFERENCE ON NEURAL NETWORKS (IJCNN)(2021)
摘要
How can we find a subset of training samples that are most responsible for a specific prediction made by a complex black-box machine learning model? More generally, how can we explain the model's decisions to end-users in a transparent way? We propose a new model-agnostic algorithm to identify a minimal set of training samples that are indispensable for a given model's decision at a particular test point, i.e., the model's decision would have changed upon the removal of this subset from the training dataset. Our algorithm identifies such a set of “indispensable” samples iteratively by solving a constrained optimization problem. Further, we speed up the algorithm through efficient approximations and provide theoretical justification for its performance. To demonstrate the applicability and effectiveness of our approach, we apply it to a variety of tasks including data poisoning detection, training set debugging and understanding loan decisions. The results show that our algorithm is an effective and easy-to-comprehend tool that helps to better understand local model behavior, and therefore facilitates the adoption of machine learning in domains where such understanding is a requisite.
更多查看译文
关键词
Training,Machine learning algorithms,Machine learning,Predictive models,Tools,Approximation algorithms,Data models
AI 理解论文
溯源树
样例
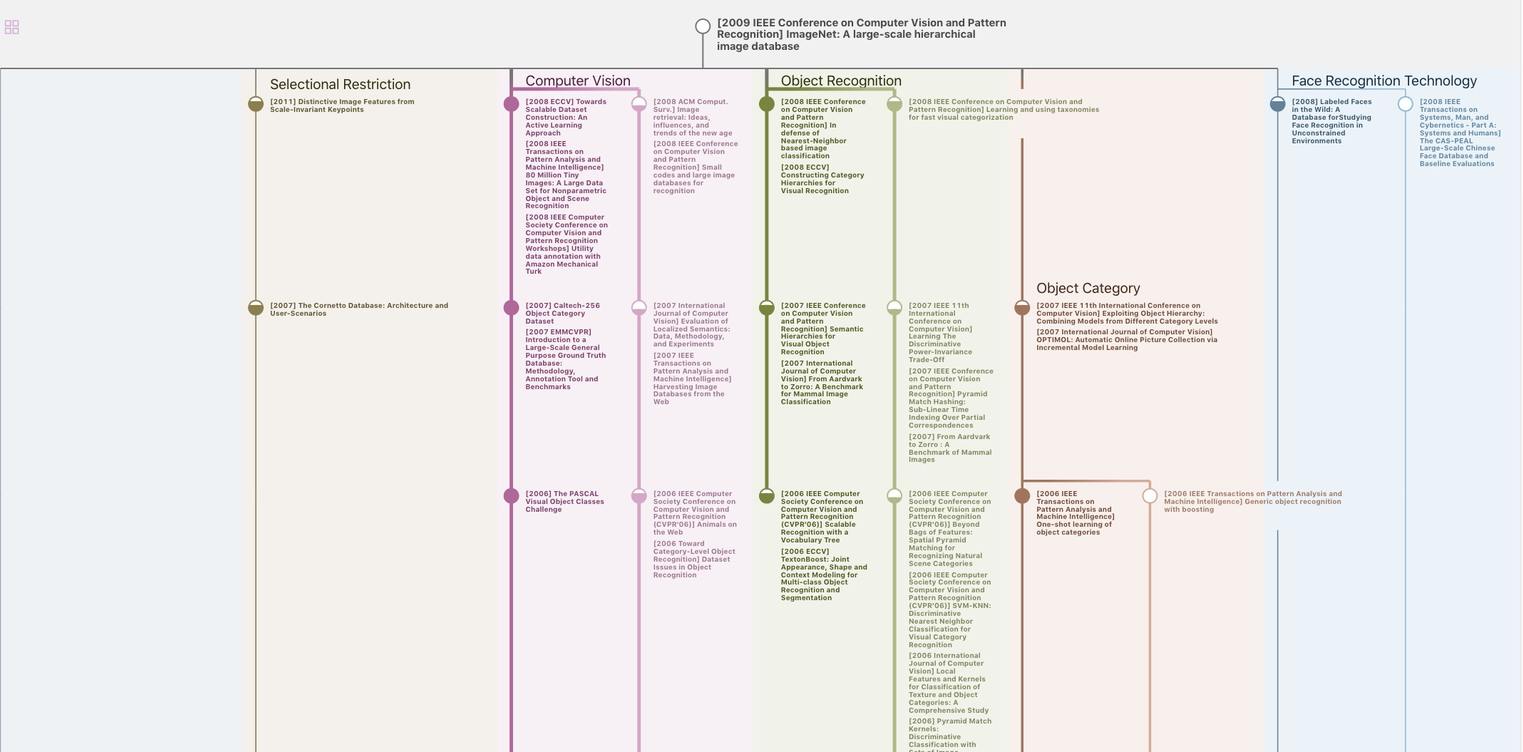
生成溯源树,研究论文发展脉络
Chat Paper
正在生成论文摘要