Learning Dynamic Insurance Recommendations from Users’ Click Sessions
RECSYS(2021)
摘要
ABSTRACT While personalised recommendations have been most successful in domains like retail due to large volume of users’ feedback on items, it is challenging to implement traditional recommender systems into the insurance domain where such prior information is very small in volume. This work addresses the problem of sparse feedback by studying users’ click sessions as signals for learning insurance recommendations. Our preliminary results show limitations in representing click sessions by manually engineered features. The proposed framework uses an autoencoder approach to automatically learns representation of sessions, then a neural network approach to model dependencies across sessions that can be used to predict recommendations. Thereby, it is further able to capture users’ dynamic needs of insurance products evolving over time.
更多查看译文
关键词
session-based recommender systems, insurance domain, click-based models
AI 理解论文
溯源树
样例
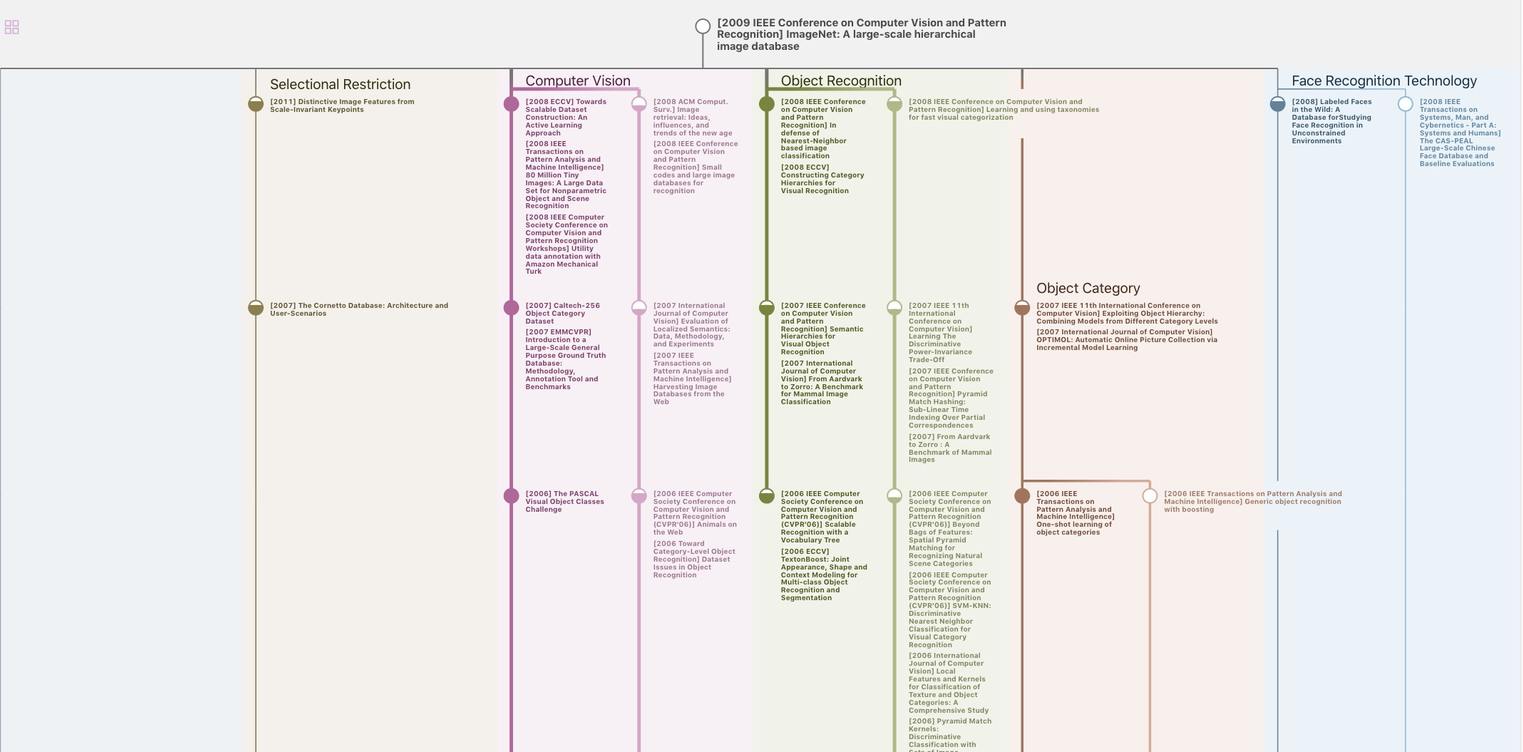
生成溯源树,研究论文发展脉络
Chat Paper
正在生成论文摘要