ProtoCF: Prototypical Collaborative Filtering for Few-shot Recommendation
RECSYS(2021)
摘要
ABSTRACTIn recent times, deep learning methods have supplanted conventional collaborative filtering approaches as the backbone of modern recommender systems. However, their gains are skewed towards popular items with a drastic performance drop for the vast collection of long-tail items with sparse interactions. Moreover, we empirically show that prior neural recommenders lack the resolution power to accurately rank relevant items within the long-tail. In this paper, we formulate long-tail item recommendations as a few-shot learning problem of learning-to-recommend few-shot items with very few interactions. We propose a novel meta-learning framework ProtoCF that learns-to-compose robust prototype representations for few-shot items. ProtoCF utilizes episodic few-shot learning to extract meta-knowledge across a collection of diverse meta-training tasks designed to mimic item ranking within the tail. To further enhance discriminative power, we propose a novel architecture-agnostic technique based on knowledge distillation to extract, relate, and transfer knowledge from neural base recommenders. Our experimental results demonstrate that ProtoCF consistently outperforms state-of-art approaches on overall recommendation (by 5% [email protected]) while achieving significant gains (of 60-80% [email protected]) for tail items with less than 20 interactions.
更多查看译文
关键词
Recommendation System, Collaborative Filtering, Meta Learning, Few-shot learning
AI 理解论文
溯源树
样例
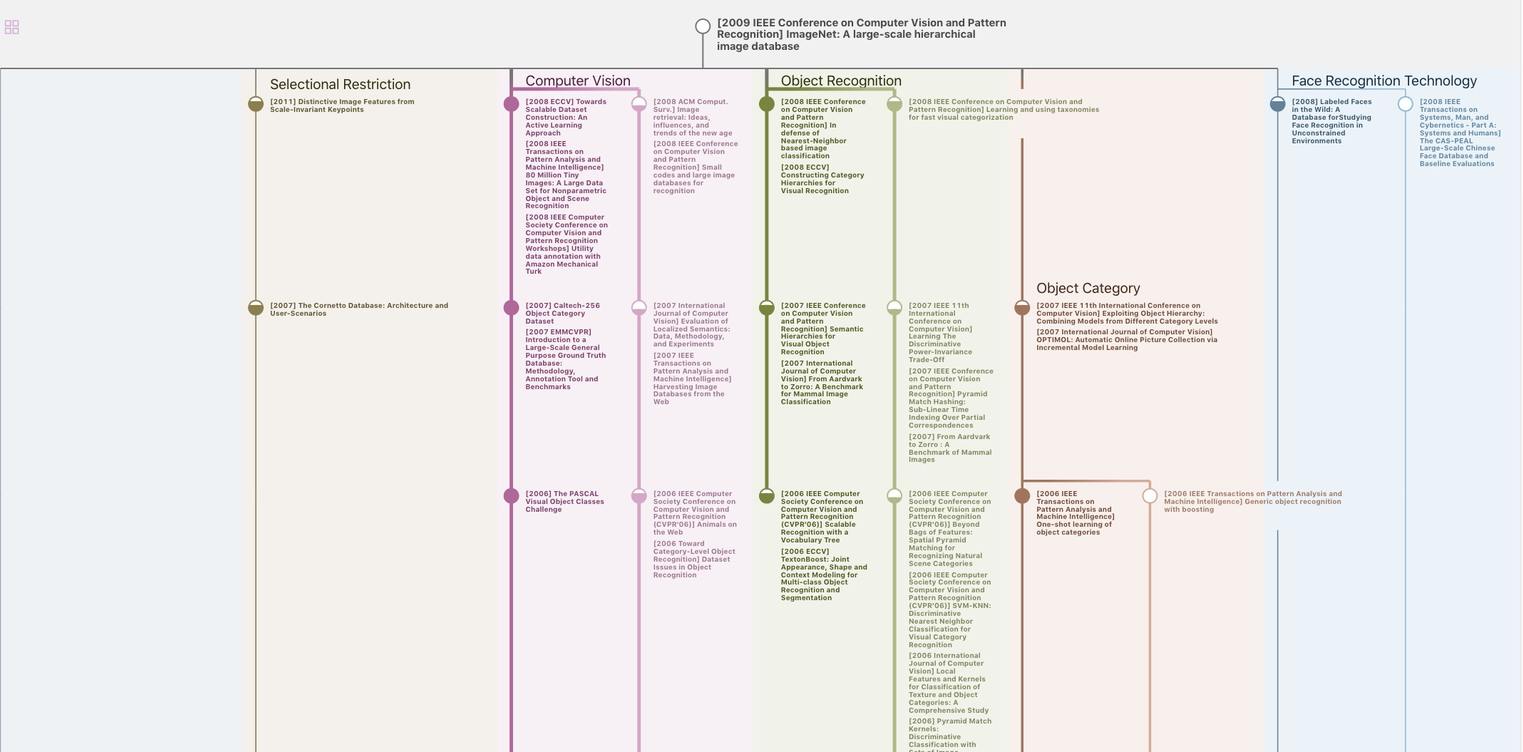
生成溯源树,研究论文发展脉络
Chat Paper
正在生成论文摘要