A Constrained Optimization Approach for Calibrated Recommendations
RECSYS(2021)
摘要
ABSTRACTIn recommender systems (RS) it is important to ensure that the various (past) areas of interest of a user are reflected with their corresponding proportions in the recommendation lists. In other words, when a user has watched, say, 60 romance movies and 40 Comedy movies, then it is reasonable to expect the personalized list of recommended movies to contain about 60% romance and 40% comedy movies as well. This property is known as calibration, and it has recently received much attention in the RS community. Greedy heuristic approaches have been proposed to calibrate recommendations, and although they provide great improvements, they can result in inefficient solutions in that a better one can be missed because of the myopic nature of these algorithms. This paper addresses the calibration problem from a constrained optimization perspective and provides a model to combine both accuracy and calibration. Experimental results show that our approach outperforms the state-of-the-art heuristics for calibration in most cases on both accuracy of the recommendations and the level of calibrations the recommendation lists achieve. We give a small example to illustrate why the heuristic fails to find the optimal solution.
更多查看译文
关键词
Recommender systems, optimization, calibration, multi-objective
AI 理解论文
溯源树
样例
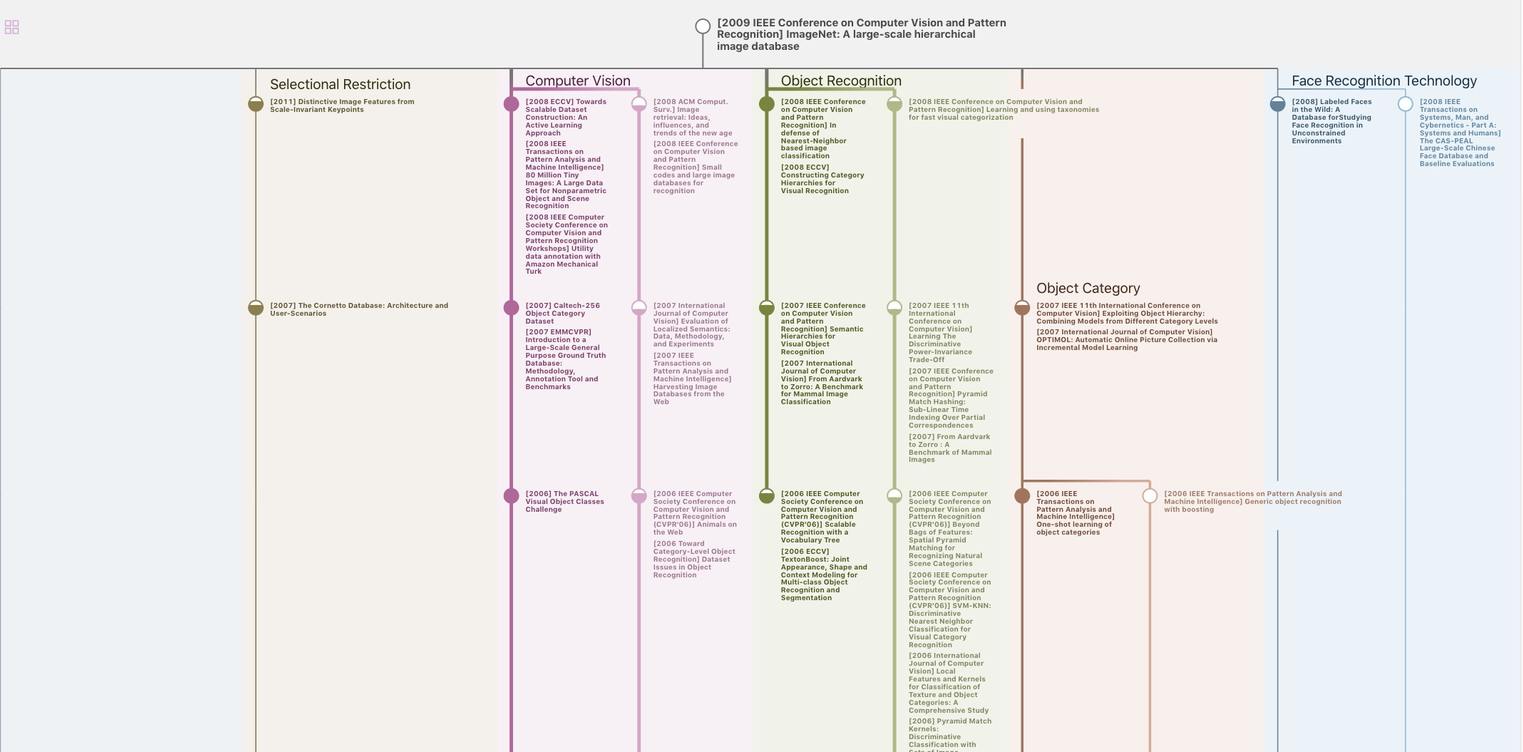
生成溯源树,研究论文发展脉络
Chat Paper
正在生成论文摘要