The supremum principle selects simple, transferable models
arxiv(2021)
摘要
Humans use analogical reasoning to connect understanding of one system to another. Can machines use similar abstractions to transfer their learning from training data to other regimes? The Manifold Boundary Approximation Method constructs simple, reduced models of target phenomena in a data-driven way. We consider the set of all such reduced models and use the topological relationships among them to reason about model selection for new, unobserved phenomena. Given minimal models for several target behaviors, we introduce the supremum principle as a criterion for selecting a new, transferable model. The supremal model, i.e., the least upper bound, is the simplest model that reduces to each of the target behaviors. By unifying the relevant mechanisms, the supremal model successfully transfers to new target domains. We illustrate on a simple epidemiological example and elaborate on Wnt signaling from developmental biology. We present a general algorithm for constructing a supremal model and note formal connections to theories of analogical reasoning in cognitive psychology.
更多查看译文
关键词
models,supremum principle
AI 理解论文
溯源树
样例
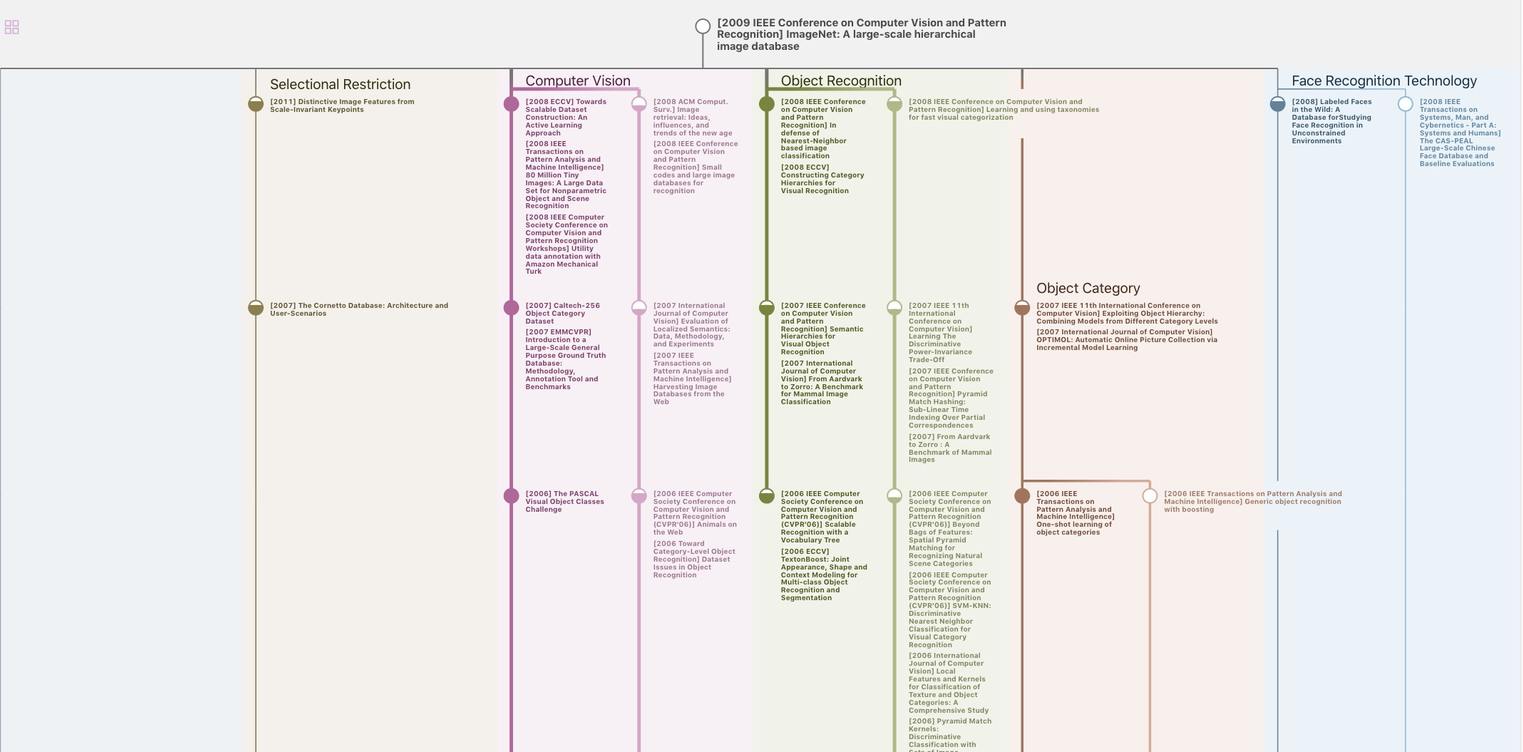
生成溯源树,研究论文发展脉络
Chat Paper
正在生成论文摘要