Self-Supervised Learning to Prove Equivalence Between Straight-Line Programs via Rewrite Rules
arxiv(2023)
摘要
We target the problem of automatically synthesizing proofs of semantic equivalence between two programs made of sequences of statements. We represent programs using abstract syntax trees (AST), where a given set of semantics-preserving rewrite rules can be applied on a specific AST pattern to generate a transformed and semantically equivalent program. In our system, two programs are equivalent if there exists a sequence of application of these rewrite rules that leads to rewriting one program into the other. We propose a neural network architecture based on a transformer model to generate proofs of equivalence between program pairs. The system outputs a sequence of rewrites, and the validity of the sequence is simply checked by verifying it can be applied. If no valid sequence is produced by the neural network, the system reports the programs as non-equivalent, ensuring by design no programs may be incorrectly reported as equivalent. Our system is fully implemented for a given grammar which can represent straight-line programs with function calls and multiple types. To efficiently train the system to generate such sequences, we develop an original incremental training technique, named self-supervised sample selection. We extensively study the effectiveness of this novel training approach on proofs of increasing complexity and length. Our system, S4Eq, achieves 97% proof success on a curated dataset of 10,000 pairs of equivalent programs
更多查看译文
关键词
Machine learning,program equivalence,self-supervised learning,symbolic reasoning
AI 理解论文
溯源树
样例
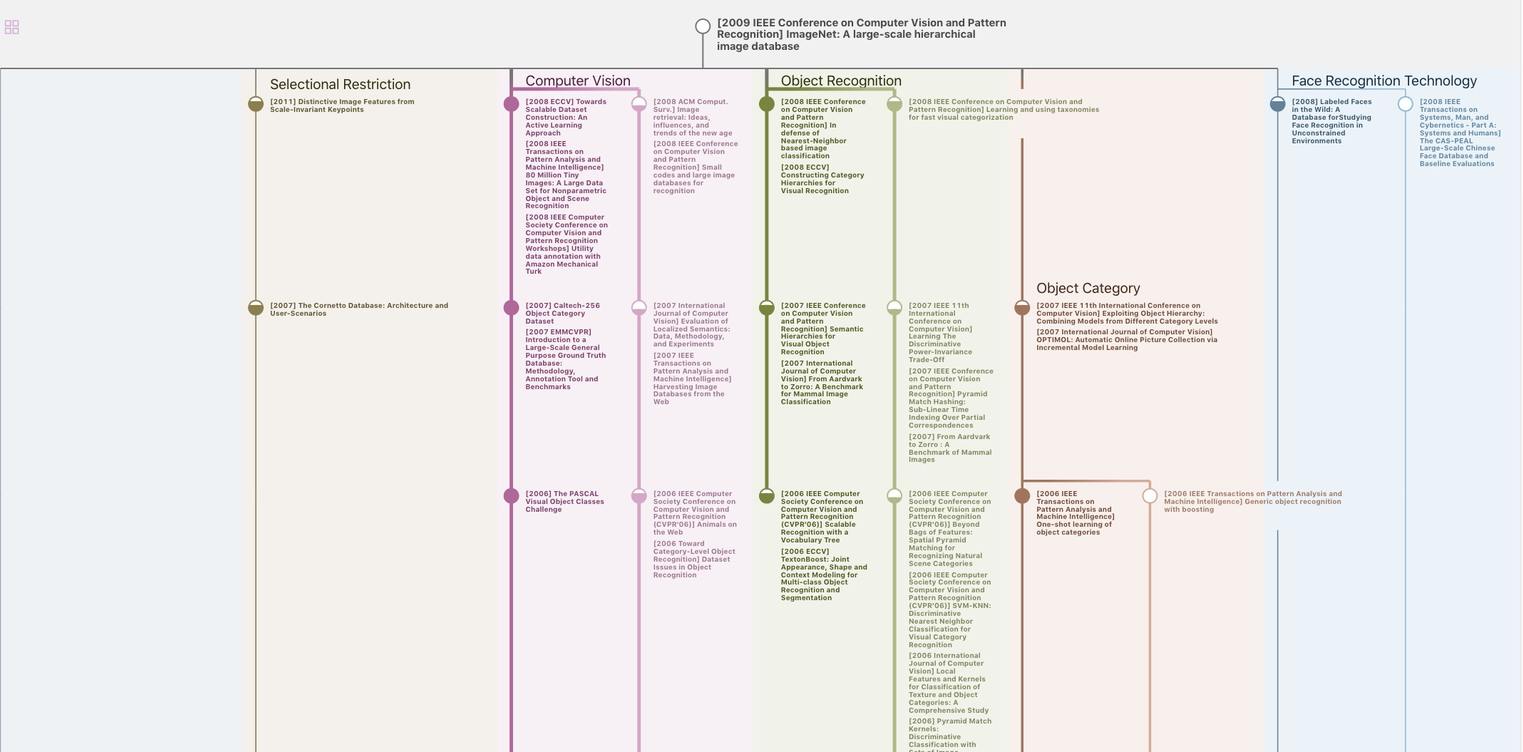
生成溯源树,研究论文发展脉络
Chat Paper
正在生成论文摘要