A Content-based Approach for the Analysis and Classification of Vaccine-related Stances on Twitter: the Italian Scenario.
International Conference on Web and Social Media (ICWSM)(2021)
摘要
One year after the outbreak of the SARS-CoV-2, several vaccines have been successfully developed to prevent its spreading, and vaccine roll-out campaigns are taking place worldwide. However, an increasing number of individuals is still hesitant towards getting vaccinated, and this poses a serious threat to reaching herd immunity. We collect and analyze Italian online conversations about COVID-19 vaccines on Twitter. We define a hashtag-based semi-automatic approach to label large volumes of tweets as supporters or skeptical about the vaccine. We investigate the geographical, temporal and lexical distribution of data, and we train an accurate binary classifier that predicts the stance of tweets towards vaccines, i.e., it applies a “Pro-vax” or “No-vax” label. This classification approach can be used, in parallel with other affirmed techniques, to promptly detect and prevent the spread of negative and misleading messages about vaccines, ensuring higher rates of vaccine uptake. Introduction and Related Work A year after the outbreak in China, the SARS-CoV-2 has radically changed our lives, and despite the countermeasures adopted by countries across the world to prevent its spreading (Bonaccorsi et al. 2020; Spelta et al. 2020), the pandemic has infected more than 123M individuals and caused more than 2.7M deaths worldwide1. Nevertheless, we have seen the rapid development of several vaccines with over 90% effectiveness, the foremost being the one developed by PfizerBioNTech, announced in November 20202. As of March 22nd, 2021, more than 439M vaccine doses have been administered worldwide, which translates to almost 5.7 doses every 100 individuals3. Italy, in particular, has started its vaccination program on December 27th 2020, with 8M doses given to citizens4 as of March 22nd, 2021. Copyright © 2021, Association for the Advancement of Artificial Intelligence (www.aaai.org). All rights reserved. https://gisanddata.maps.arcgis.com/apps/opsdashboard/index. html#/bda7594740fd40299423467b48e9ecf6 https://www.pfizer.com/news/press-release/press-releasedetail/pfizer-and-biontech-conclude-phase-3-study-covid-19vaccine https://www.nytimes.com/interactive/2021/world/covidvaccinations-tracker.html https://www.governo.it/it/cscovid19/report-vaccini/ Although vaccination is considered one of the greatest achievements of public health, it is still perceived as unsafe and unnecessary by a growing number of individuals and the causes of this phenomenon involve emotional, cultural, social, spiritual, political and cognitive factors (Dubé et al. 2013). In particular, after the decline in measles coverage in 12 European countries in 2018, vaccine hesitancy has been included in the top-10 threats to global health in 2019 by the World Health Organization 5. Over the last decades, social media experienced a quick growth in their user-base and daily usage. Echo chamber effects, i.e. reinforcement of users’ beliefs via the interaction with a closed set of similar users, have been observed during debates about political and socially relevant topics (Colleoni, Rozza, and Arvidsson 2014; Del Vicario et al. 2016). Cossard et al. (2020) observed a similar phenomenon regarding Italian Twitter conversations about vaccines in 2019, focusing on the worrying asymmetry of the chambers’ topology. The alarming growth of skepticism, powered by social media, caused an increase of scientific contributions inspecting the phenomena from different points of view. Pierri et al. (2021a) studied online misinformation about vaccines in US, Kang et al. (2017) constructed semantic networks of vaccine information from highly shared websites of Twitter users in the United States, D’Andrea et al. (2019) trained an SVM classifier to detect the stance of tweets about vaccines, Gargiulo et al. (2020) discovered an asymmetric behaviour of defenders and critics of vaccines in the French-speaking Twitter, Broniatowski et al. (2018) focused on the effect of bots and trolls in the debate, Guarino et al. (2021) investigated the information disorders on social media. Specific to the Italian context, many contributions have been published after the Law on Mandatory Vaccinations in 2017 (Donzelli et al. 2018; Lovari, Martino, and Righetti 2021; Righetti 2020). In this work we inspect the SARS-CoV-2 vaccination debate on Italian-speaking Twitter from a textual content point of view. Our goal is to train an accurate stance classifier that detects patterns in tweets shared by supporters and skeptics of the vaccine. We design a semi-automated, humanhttps://www.who.int/news-room/spotlight/ten-threats-toglobal-health-in-2019 vaccini vaccinarsi vaccinerai vaccino vaccinare vaccineremo vaccinazioni vacciniamoci vaccinerete iononmivaccino vaccinareh24 iononmivaccinero vaccinazione vaccinerò novaccinoainovax vaccinocovid vaccinoanticovid iononsonounacavia Table 1: List of keywords used to filter tweets. They refer to vaccine, vaccinate, vaccination. in-the-loop, hashtag-based approach to label a large set of Italian tweets. We inspect the obtained labeled dataset by focusing on the location and date of tweets, and lexical patterns, looking at possible correlations and induced biases. Finally, we successfully train a BERT (Devlin et al. 2019) model to classify the stance of tweets (“No-Vax” vs “ProVax”), observing high values of AUROC and F1 score also on a dataset of manually labeled tweets that cannot be classified by the semi-automated approach previously defined. Our model can be used to monitor on real time the vaccination debate, independently on both the shared trending hashtags and the underneath social graph.
更多查看译文
AI 理解论文
溯源树
样例
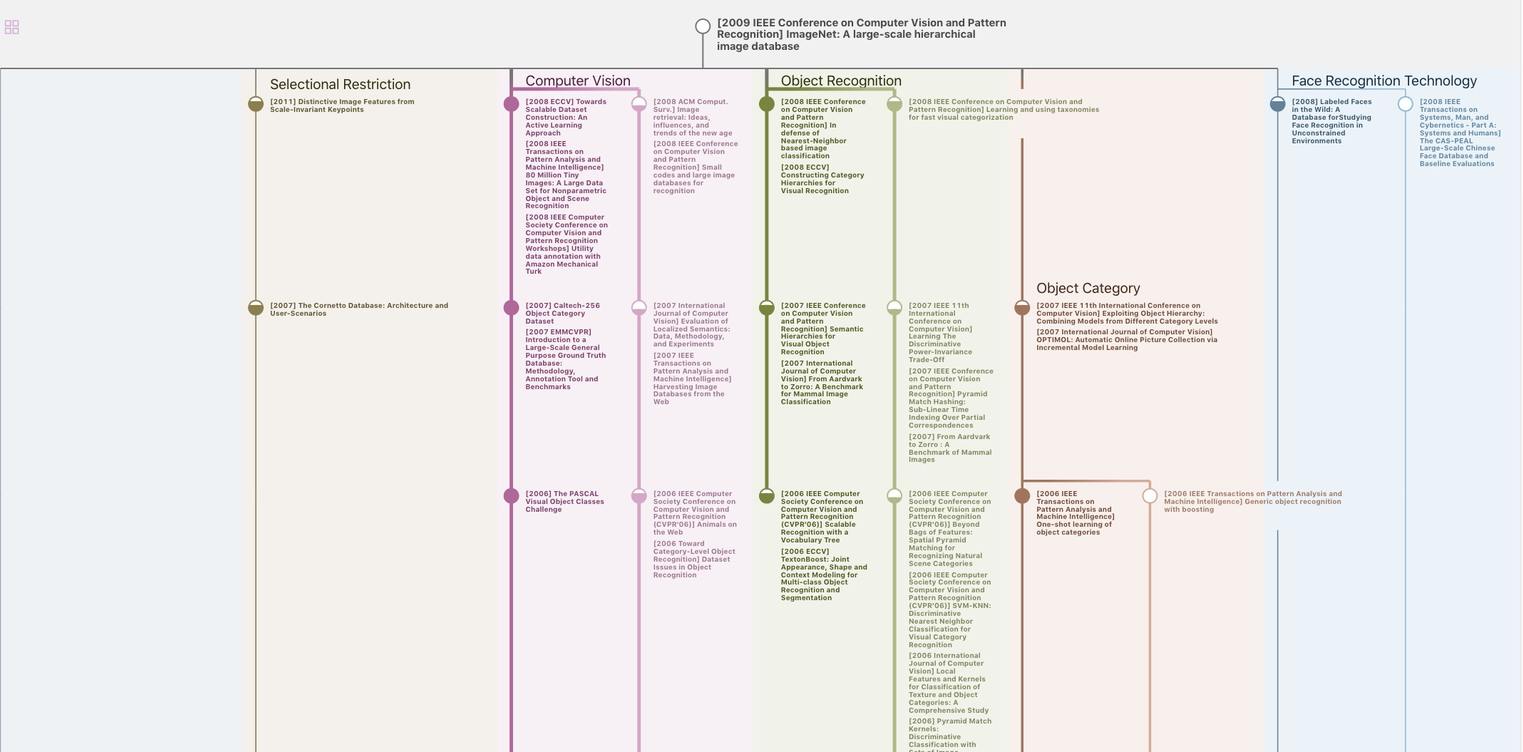
生成溯源树,研究论文发展脉络
Chat Paper
正在生成论文摘要