Systematic Generalization on gSCAN - What is Nearly Solved and What is Next?
EMNLP(2021)
摘要
We analyze the grounded SCAN (gSCAN) benchmark, which was recently proposed to study systematic generalization for grounded language understanding. First, we study which aspects of the original benchmark can be solved by commonly used methods in multi-modal research. We find that a general-purpose Transformer-based model with cross-modal attention achieves strong performance on a majority of the gSCAN splits, surprisingly outperforming more specialized approaches from prior work. Furthermore, our analysis suggests that many of the remaining errors reveal the same fundamental challenge in systematic generalization of linguistic constructs regardless of visual context. Second, inspired by this finding, we propose challenging new tasks for gSCAN by generating data to incorporate relations between objects in the visual environment. Finally, we find that current models are surprisingly data inefficient given the narrow scope of commands in gSCAN, suggesting another challenge for future work.
更多查看译文
关键词
gscan
AI 理解论文
溯源树
样例
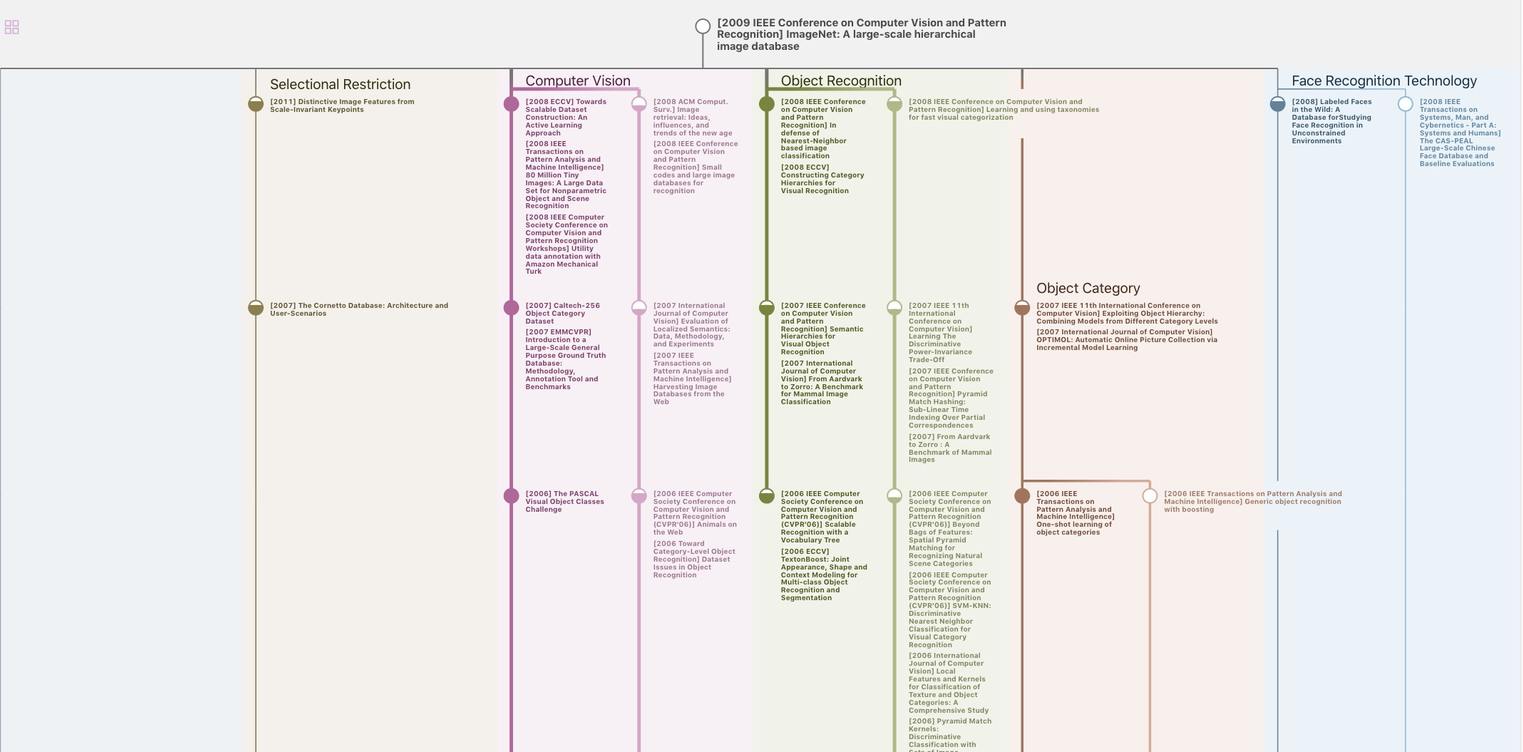
生成溯源树,研究论文发展脉络
Chat Paper
正在生成论文摘要