Understanding and Overcoming the Challenges of Efficient Transformer Quantization
EMNLP(2021)
摘要
Transformer-based architectures have become the de-facto standard models for a wide range of Natural Language Processing tasks. However, their memory footprint and high latency are prohibitive for efficient deployment and inference on resource-limited devices. In this work, we explore quantization for transformers. We show that transformers have unique quantization challenges - namely, high dynamic activation ranges that are difficult to represent with a low bit fixed-point format. We establish that these activations contain structured outliers in the residual connections that encourage specific attention patterns, such as attending to the special separator token. To combat these challenges, we present three solutions based on post-training quantization and quantization-aware training, each with a different set of compromises for accuracy, model size, and ease of use. In particular, we introduce a novel quantization scheme - per-embedding-group quantization. We demonstrate the effectiveness of our methods on the GLUE benchmark using BERT, establishing state-of-the-art results for post-training quantization. Finally, we show that transformer weights and embeddings can be quantized to ultra-low bit-widths, leading to significant memory savings with a minimum accuracy loss.
更多查看译文
关键词
quantization,efficient transformer
AI 理解论文
溯源树
样例
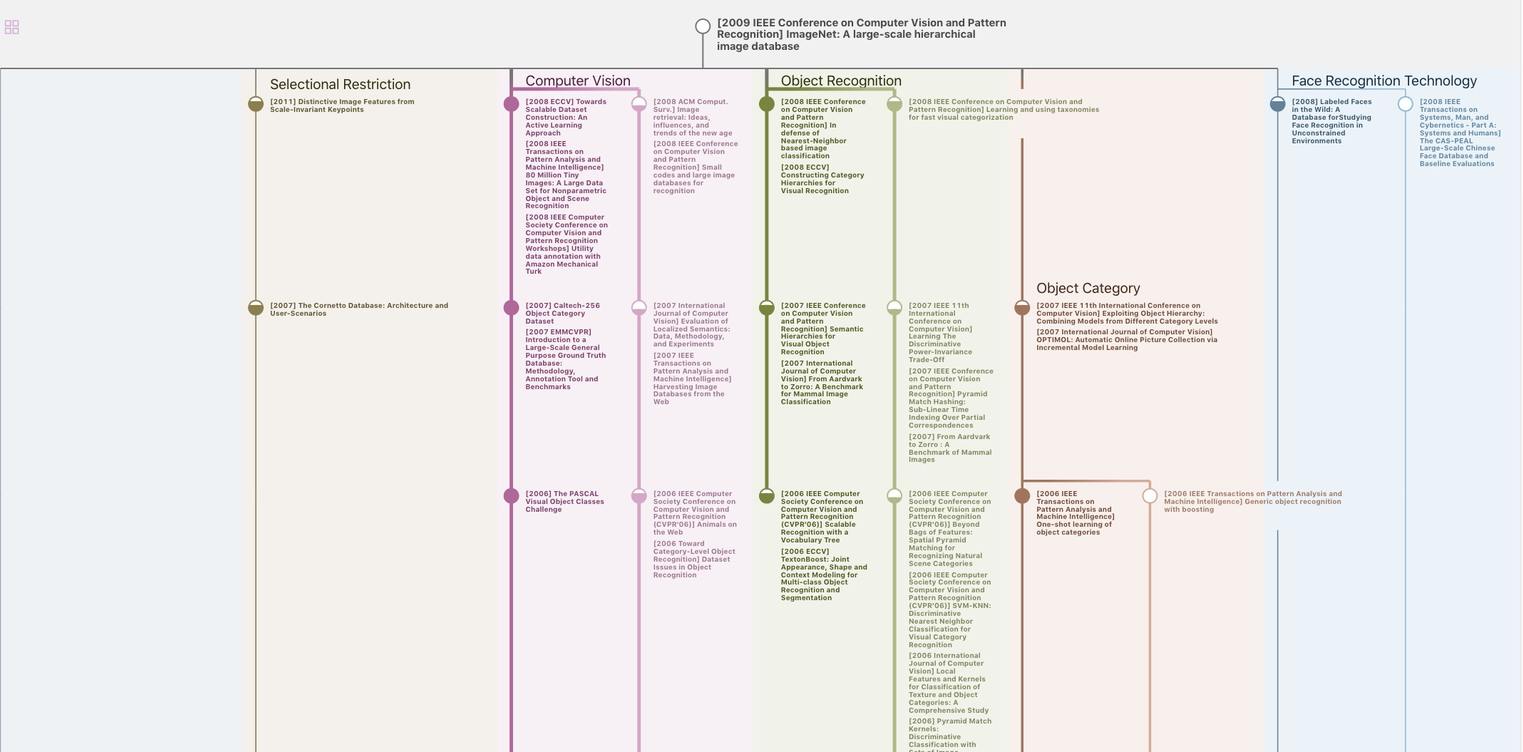
生成溯源树,研究论文发展脉络
Chat Paper
正在生成论文摘要