Audio-to-Image Cross-Modal Generation
IEEE International Joint Conference on Neural Network (IJCNN)(2022)
摘要
Cross-modal representation learning allows to integrate information from different modalities into one representation. At the same time, research on generative models tends to focus on the visual domain with less emphasis on other domains, such as audio or text, potentially missing the benefits of shared representations. Studies successfully linking more than one modality in the generative setting are rare. In this context, we verify the possibility to train variational autoencoders (VAEs) to reconstruct image archetypes from audio data. Specifically, we consider VAEs in an adversarial training framework in order to ensure more variability in the generated data and find that there is a trade-off between the consistency and diversity of the generated images - this trade-off can be governed by scaling the reconstruction loss up or down, respectively. Our results further suggest that even in the case when the generated images are relatively inconsistent (diverse), features that are critical for proper image classification are preserved.
更多查看译文
关键词
neural networks,cross-modal learning,generative models
AI 理解论文
溯源树
样例
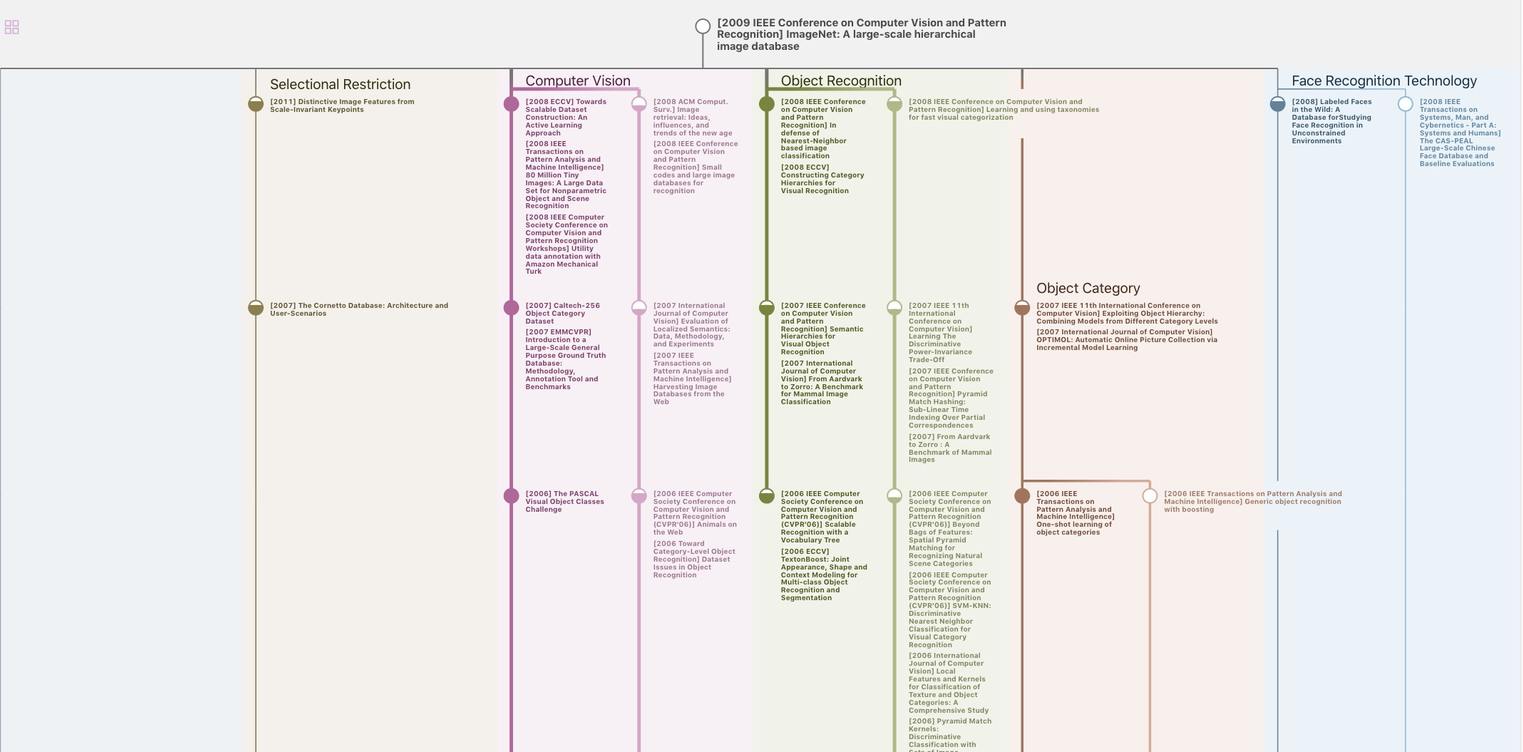
生成溯源树,研究论文发展脉络
Chat Paper
正在生成论文摘要