Deep Neural Network-Aided Soft-Demapping in Coherent Optical Systems: Regression Versus Classification
arxiv(2022)
摘要
We examine here what type of predictive modelling, classification, or regression, using neural networks (NN), fits better the task of soft-demapping based post-processing in coherent optical communications, where the transmission channel is nonlinear and dispersive. For the first time, we present possible drawbacks in using each type of predictive task in a machine learning context, considering the nonlinear coherent optical channel equalization/soft-demapping problem. We study two types of equalizers based on the feed-forward and recurrent NNs, for several transmission scenarios, in linear and nonlinear regimes of the optical channel. We point out that even though from the information theory perspective the cross-entropy loss (classification) is the most suitable option for our problem, the NN models based on the cross-entropy loss function can severely suffer from learning problems. The latter translates into the fact that regression-based learning is typically superior in terms of delivering higher Q-factor and achievable information rates. In short, we show by empirical evidence that loss functions based on cross-entropy may not be necessarily the most suitable option for training communication systems in practical scenarios when overfitting- and vanishing gradients-related problems come into play.
更多查看译文
关键词
Neural networks,nonlinear equalizer,classification,regression,coherent detection,digital signal processing,optical communications
AI 理解论文
溯源树
样例
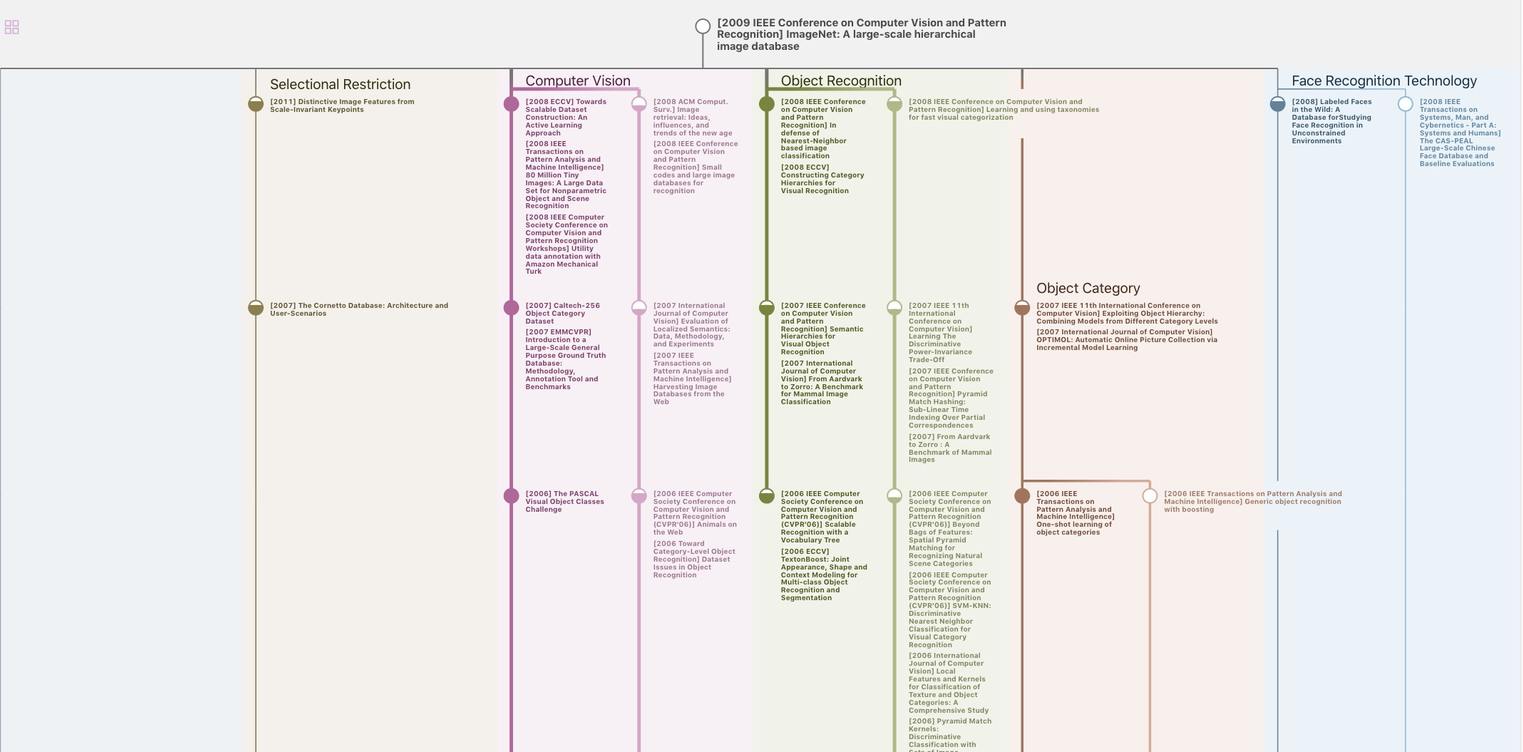
生成溯源树,研究论文发展脉络
Chat Paper
正在生成论文摘要