Single-shot Semantic Matching Network for Moment Localization in Videos
ACM Transactions on Multimedia Computing, Communications, and Applications(2021)
摘要
AbstractMoment localization in videos using natural language refers to finding the most relevant segment from videos given a natural language query. Most of the existing methods require video segment candidates for further matching with the query, which leads to extra computational costs, and they may also not locate the relevant moments under any length evaluated. To address these issues, we present a lightweight single-shot semantic matching network (SSMN) to avoid the complex computations required to match the query and the segment candidates, and the proposed SSMN can locate moments of any length theoretically. Using the proposed SSMN, video features are first uniformly sampled to a fixed number, while the query sentence features are generated and enhanced by GloVe, long-term short memory (LSTM), and soft-attention modules. Subsequently, the video features and sentence features are fed to an enhanced cross-modal attention model to mine the semantic relationships between vision and language. Finally, a score predictor and a location predictor are designed to locate the start and stop indexes of the query moment. We evaluate the proposed method on two benchmark datasets and the experimental results demonstrate that SSMN outperforms state-of-the-art methods in both precision and efficiency.
更多查看译文
关键词
Multimodal retrieval, moment localization, visual comprehension, natural language understanding
AI 理解论文
溯源树
样例
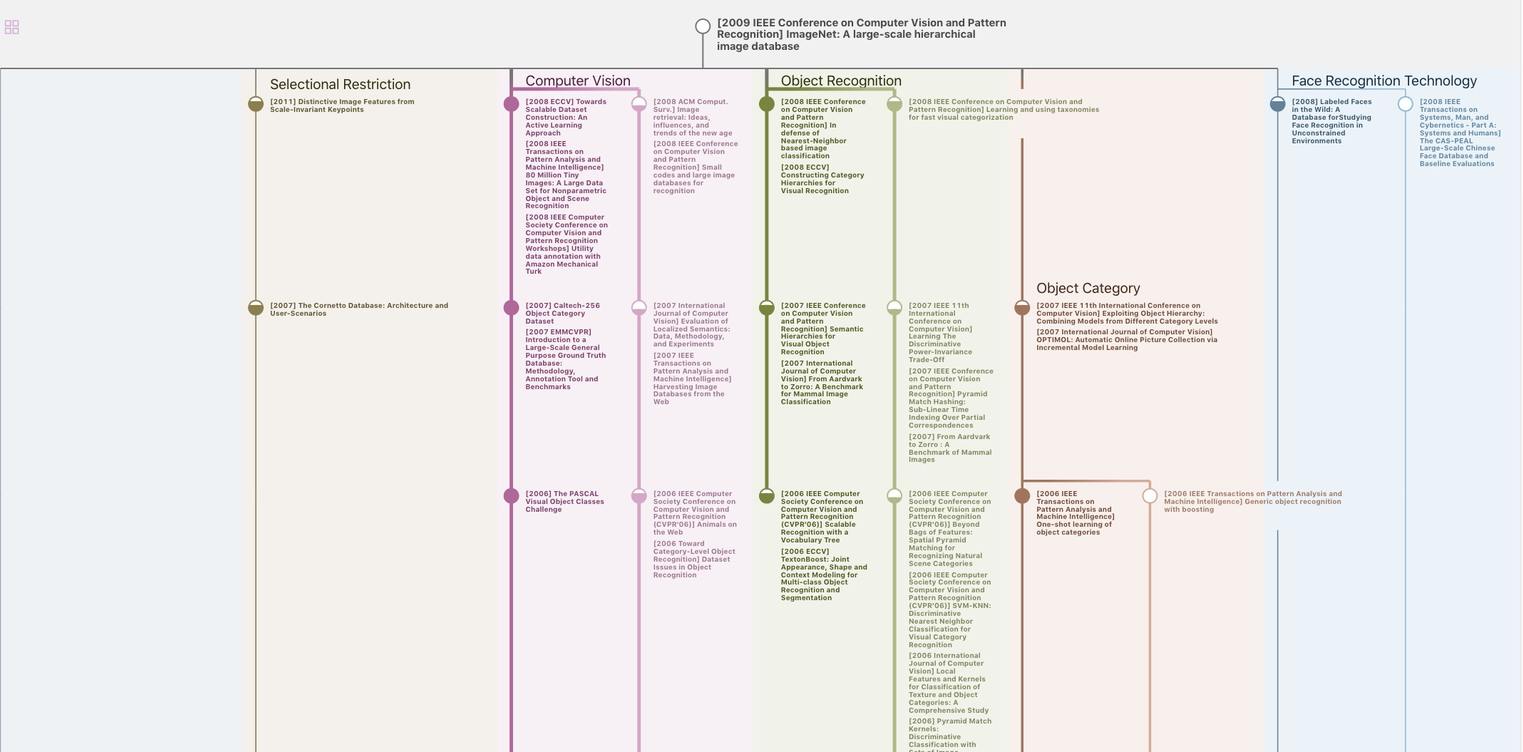
生成溯源树,研究论文发展脉络
Chat Paper
正在生成论文摘要