Keypoint-Based Disentangled Pose Network for Category-Level 6-D Object Pose Tracking
IEEE Computer Graphics and Applications(2022)
摘要
Category-level 6-D object pose tracking is very challenging in the field of 3-D computer vision. Keypoint-based object pose estimation has demonstrated its effectiveness in dealing with it. However, current approaches first estimate the keypoints through a neural network and further compute the interframe pose change via least-squares optimization. They estimate rotation and translation in the same way, ignoring the differences between them. In this work, we propose a keypoint-based disentangled pose network, which disentangles the 6-D object pose change to 3-D rotation and 3-D translation. Specifically, the translation is directly estimated by the network and the rotation is indirectly calculated by singular value decomposition according to the keypoints. Extensive experiments on the NOCS-REAL275 dataset demonstrate the superiority of our method.
更多查看译文
关键词
Three-dimensional displays, Feature extraction, Pose estimation, Solid modeling, Neural networks, Transforms, Training data
AI 理解论文
溯源树
样例
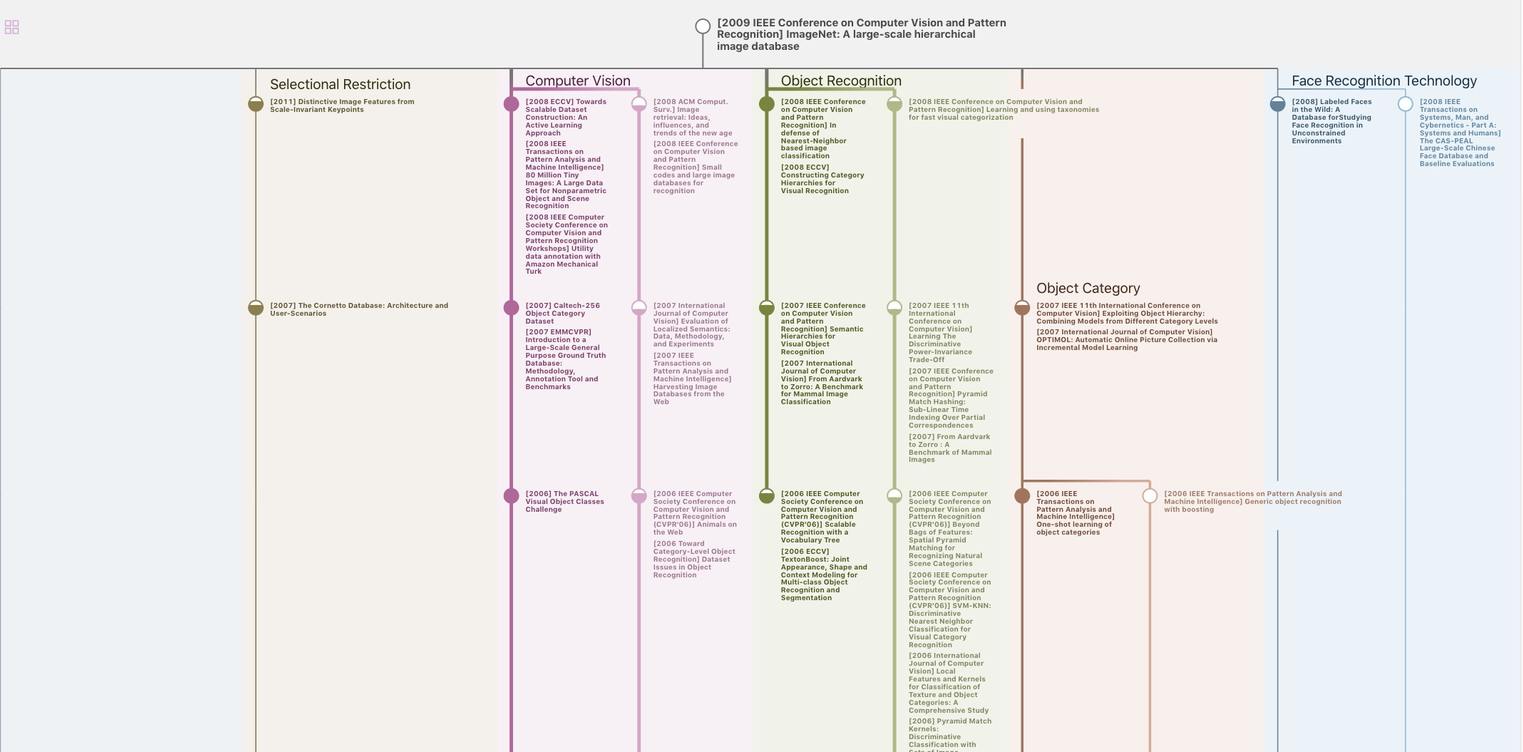
生成溯源树,研究论文发展脉络
Chat Paper
正在生成论文摘要