Bayesian statistics versus classical statistics in survival analysis: an applicable example
AMERICAN JOURNAL OF CARDIOVASCULAR DISEASE(2021)
摘要
Background: Heart disease is the leading cause of death in the world and 17 million people die from cardiovascular diseases around the world each year, so finding factors that affect the survival of these patients is of particular importance. Therefore, finding the best model to analyze patient survival can help to find more accurate results. Methods: There are different methods to survival analysis that assess one or more risk factors; the classic Kaplan-Meier method, Cox regression, parametric survival models, and newer models such as Bayesian survival. Cox regression is most common and is generally used for time-dependent data, and the main difference between cox regression and Bayesian models is that the prior distribution in Bayesian models can affect the values of the parameters. Some survival analysis models have certain conditions that need to be considered before analyzing the data. In this paper, we use a dataset from Kaggle and discuss these conditions. This dataset contains medical records of 299 patients with heart failure collected at the Faisalabad Institute of Cardiology and the Allied Hospital in Faisalabad (Punjab, Pakistan) from April to December 2015. Results: This paper discusses that if the effective sample size is not sufficient, Bayesian survival models can be used to achieve more accurate results because this model is not affected by the sample size. The results of both methods are shown on a sample of cardiac data and based on the results of Bayesian Cox regression model, it was observed that Age, Anemia, Ejection fraction, High blood pressure and Serum creatinine were effective on patient survival. Conclusion: The Bayesian models are much more accurate to determine survival and determine risk factors when dealing with data on rare diseases or diseases with low mortality, including heart patients whose survival probability is higher than that of cancer patients.
更多查看译文
关键词
Heart failure,survival,cox regression,Bayesian
AI 理解论文
溯源树
样例
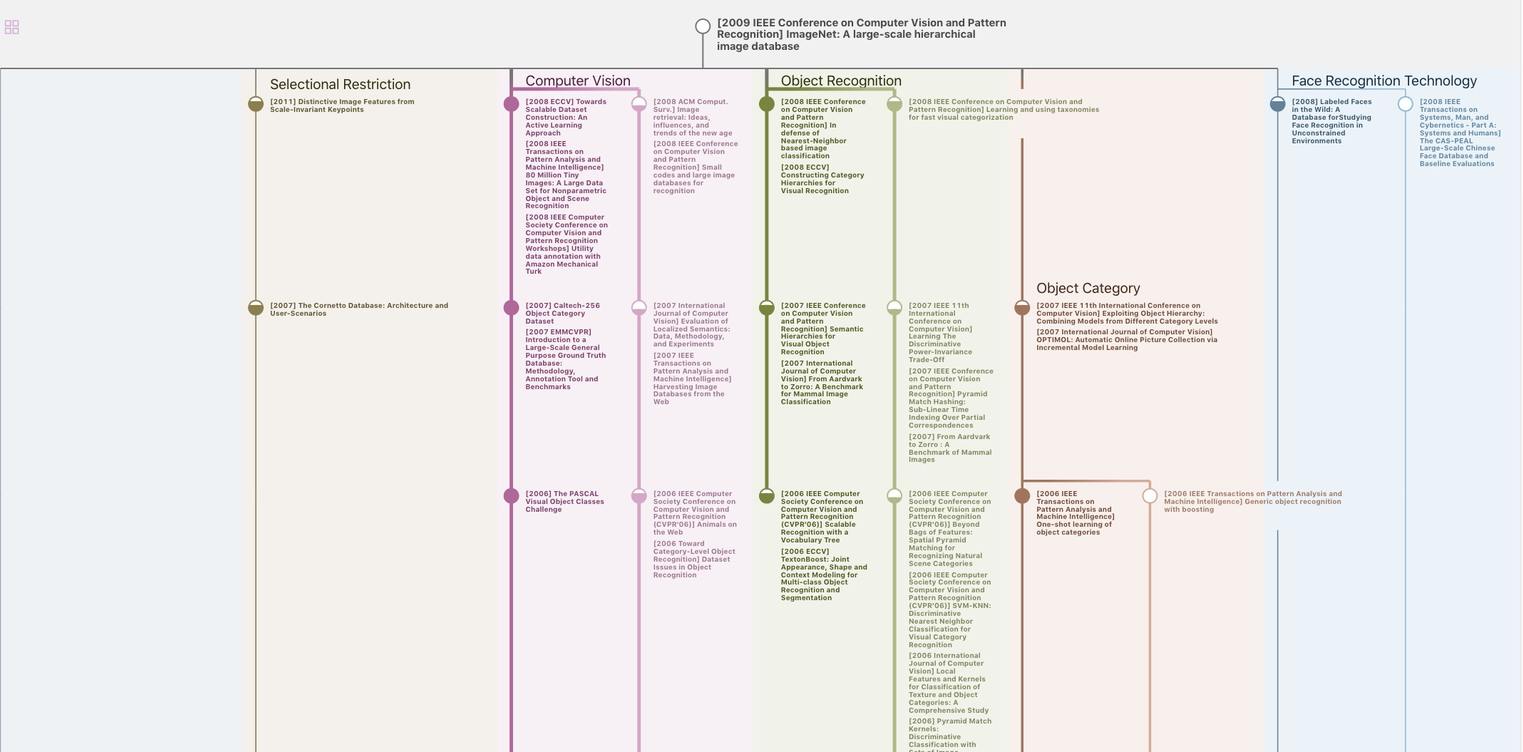
生成溯源树,研究论文发展脉络
Chat Paper
正在生成论文摘要