Multi-supply multi-capacitated p-median location optimization via a hybrid bi-level intelligent algorithm
COMPUTERS & INDUSTRIAL ENGINEERING(2021)
摘要
The Capacitated P-Median Problem (CPMP) is a classic discrete facility location problem in which p capacitated facilities (medians) are selected from an economic point of view to serve a set of demand vertexes so that the total demand assigned to each of the facilities (medians) does not exceed its capacity. Since the capacity used by each facility is fixed and does not depend on the vertex demand, the traditional capacitated p median problem's total capacity is underutilized. To make full use of the total capacity, a more generalized model, namely, the Multi-supply Multi-Capacitated Location Problem (MMCLP), is considered in this paper, which assumes not only that each facility is allowed to open with different capacities while meeting the constraint of total capacity but also that each demand vertex can accept supplies from different facilities. To solve the MMCLP effectively, we propose a hybrid bi-level optimization framework based on decomposition. First, the original MMCLP is decomposed into numerous mixed-integer programming (MIP) sub-problems, i.e., inner optimization is performed, and for outer optimization, a Genetic Algorithm based on Co-Evolution of Population and Neighborhood (GACEPN) is proposed to find better sub-problems near the good sub-problems among all the candidate sub-problems. The inner optimization problem is solved by a commercial MIP solver. The results obtained are returned to the outer layer so that the neighborhood of the outer layer optimization can be adjusted appropriately to generate better sub-problems, thereby approaching the optimal solution of the original problem. The experiments show that for a large-scale MMCLP, compared to the Gurobi solver, the proposed bi-level algorithm (a hybrid of the GACEPN and Gurobi) can find a better solution in less time.
更多查看译文
关键词
Facility location,Decomposition,Bi-level optimization,Co-evolution,Gurobi
AI 理解论文
溯源树
样例
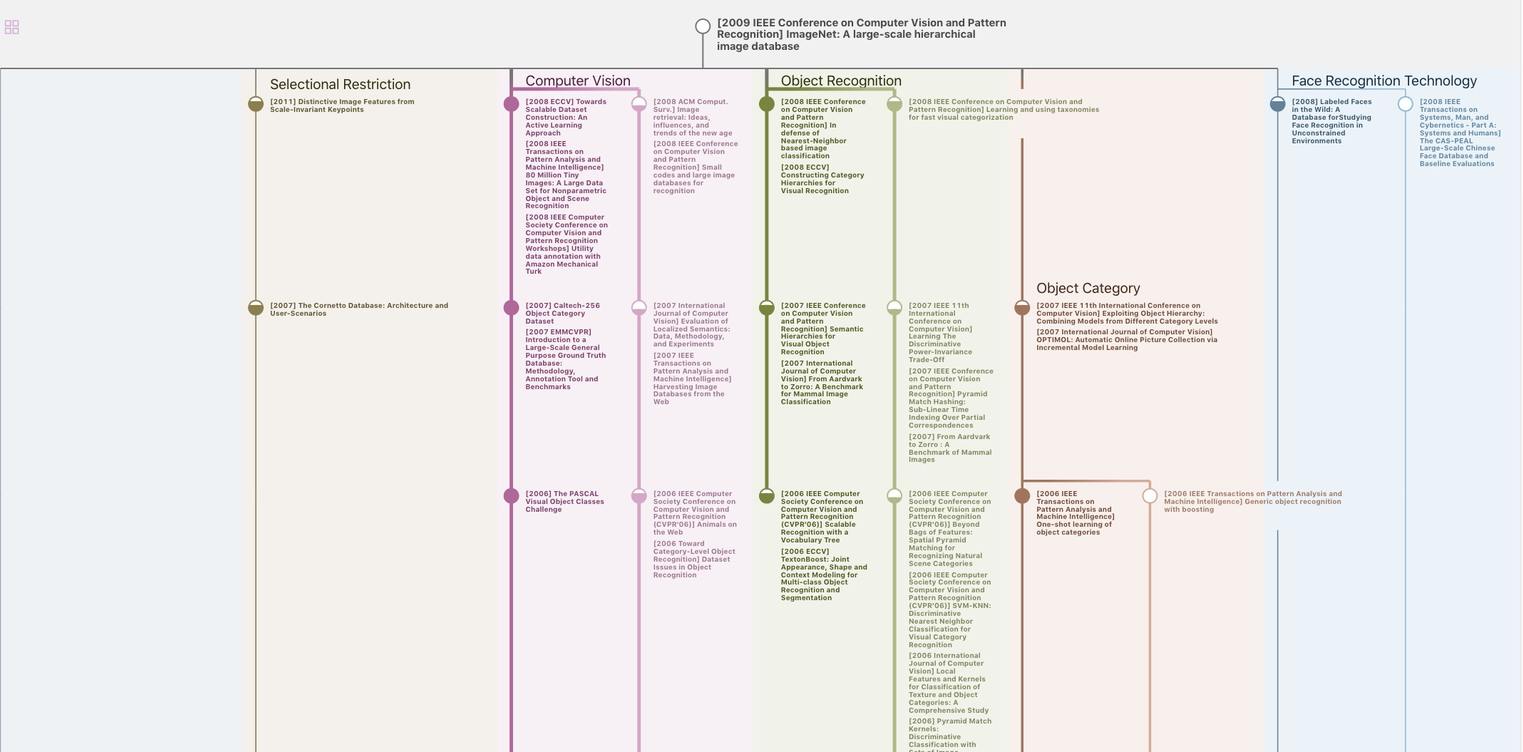
生成溯源树,研究论文发展脉络
Chat Paper
正在生成论文摘要