Extending Density Surface Models To Include Multiple And Double-Observer Survey Data
PEERJ(2021)
摘要
Spatial models of density and abundance are widely used in both ecological research (e.g., to study habitat use) and wildlife management (e.g., for population monitoring and environmental impact assessment). Increasingly, modellers are tasked with integrating data from multiple sources, collected via different observation processes. Distance sampling is an efficient and widely used survey and analysis technique. Within this framework, observation processes are modelled via detection functions. We seek to take multiple data sources and fit them in a single spatial model. Density surface models (DSMs) are a two-stage approach: first accounting for detectability via distance sampling methods, then modelling distribution via a generalized additive model. However, current software and theory does not address the issue of multiple data sources. We extend the DSM approach to accommodate data from multiple surveys, collected via conventional distance sampling, double-observer distance sampling (used to account for incomplete detection at zero distance) and strip transects. Variance propagation ensures that uncertainty is correctly accounted for in final estimates of abundance. Methods described here are implemented in the dsm R package. We briefly analyse two datasets to illustrate these new developments. Our new methodology enables data from multiple distance sampling surveys of different types to be treated in a single spatial model, enabling more robust abundance estimation, potentially over wider geographical or temporal domains.
更多查看译文
关键词
Density surface model, Distance sampling, Generalized additive model, Spatial modelling, Variance propagation, Abundance estimation
AI 理解论文
溯源树
样例
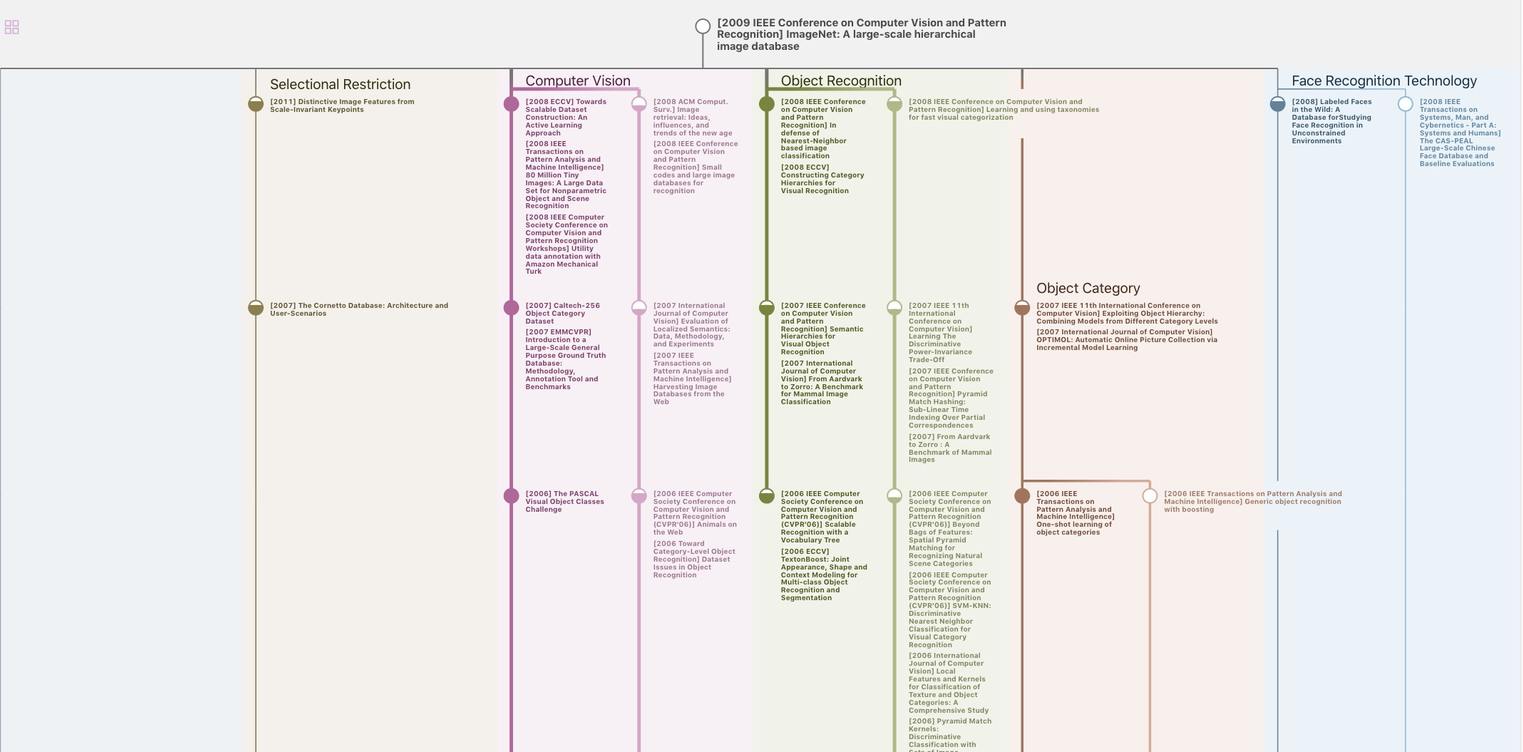
生成溯源树,研究论文发展脉络
Chat Paper
正在生成论文摘要